In-Context Exemplars as Clues to Retrieving from Large Associative Memory.
CoRR(2023)
摘要
Recently, large language models (LLMs) have made remarkable progress in natural language processing. The most representative ability of LLMs is in-context learning (ICL), which enables LLMs to learn patterns from in-context exemplars without training. The performance of ICL greatly depends on the exemplars used. However, how to choose exemplars remains unclear due to the lack of understanding of how in-context learning works. In this paper, we present a novel perspective on ICL by conceptualizing it as contextual retrieval from a model of associative memory. We establish a theoretical framework of ICL based on Hopfield Networks. Based on our framework, we look into how in-context exemplars influence the performance of ICL and propose more efficient active exemplar selection. Our study sheds new light on the mechanism of ICL by connecting it to memory retrieval, with potential implications for advancing the understanding of LLMs.
更多查看译文
关键词
memory,large associative
AI 理解论文
溯源树
样例
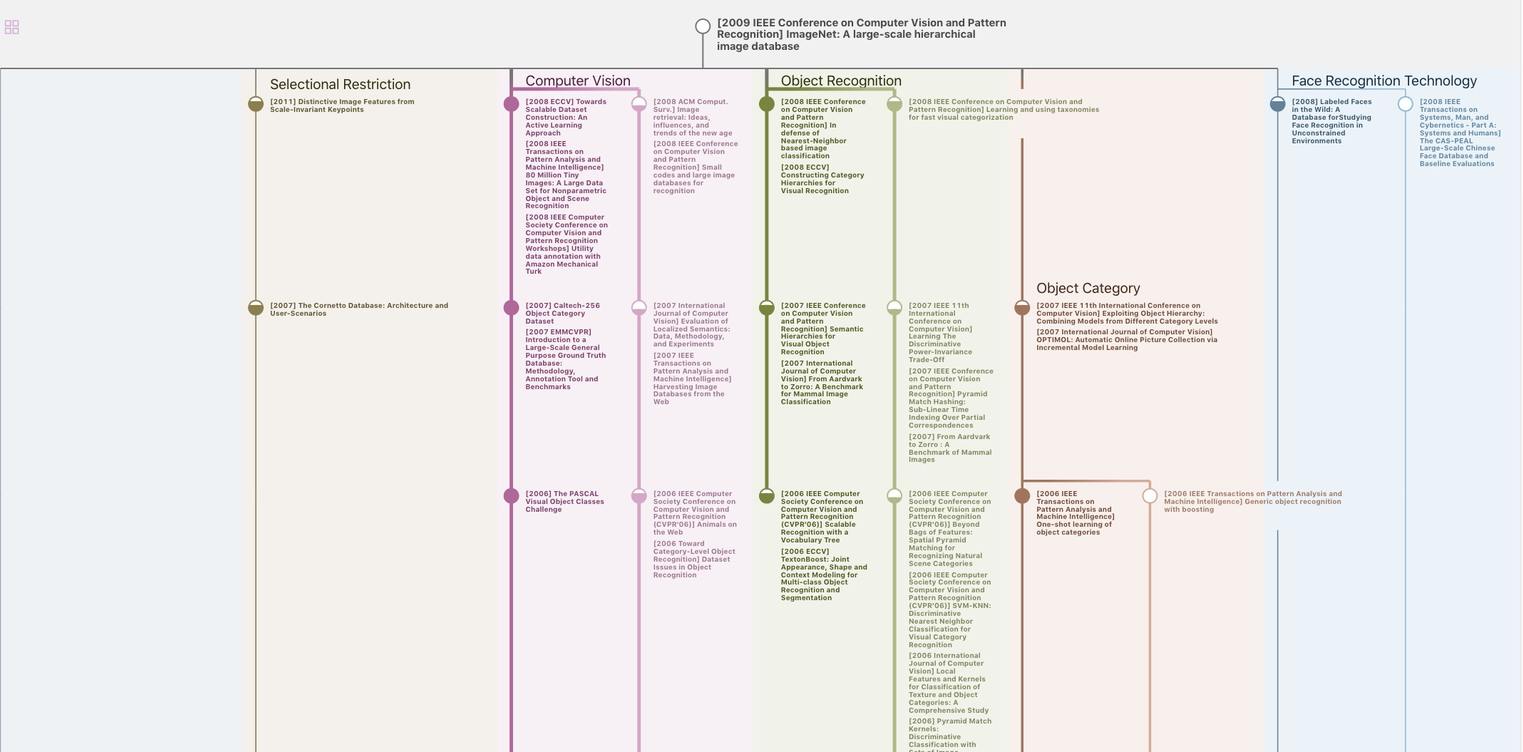
生成溯源树,研究论文发展脉络
Chat Paper
正在生成论文摘要