DiabetIA: Building Machine Learning Models for Type 2 Diabetes Complications
medRxiv (Cold Spring Harbor Laboratory)(2023)
Abstract
Background Artificial intelligence (AI) models applied to diabetes mellitus research have grown in recent years, particularly in the field of medical imaging. However little work has been done exploring real-world data (RWD) sources such as electronic health records (EHR) mostly due to the lack of reliable public diabetes databases. However, with more than 500 million patients affected worldwide, complications of this condition have catastrophic consequences. In this manuscript we aim to first extract, clean and transform a novel diabetes research database, DiabetIA, and secondly train machine learning (ML) models to predict diabetic complications.
Methods In this study, we used observational retrospective data from the Mexican Institute for Social Security (IMSS) extracting and de-identifying EHR data for almost 2 million patients seen at primary care facilities. After applying eligibility criteria for this study, we constructed a diabetes complications database. Next, we trained naïve Bayesian models with various subsets of variables, including an expert-selected model.
Results The DiabetIA database is composed of 136,674 patients (414,770 records and 447 variables), with 33,314 presenting diabetes (24.3%). The most frequent diabetic complications were diabetic foot with 2,537 patients, nephropathy with 1,914 patients, retinopathy with 1,829 patients, and neuropathy with 786 patients. These complications were accurately predicted by the Gaussian naïve Bayessian models with an average area under the curve AUC of 0.86. Our expert-selected model, achieved an average AUC of 0.84 with 21 curated variables.
Conclusion Our study offers the largest longitudinal research database from EHR data in Latin America for research. The DiabetIA database provides a useful resource to estimate the burden of diabetic complications on healthcare systems. Machine learning models can provide accurate estimations of the total cases presented in medical units. For patients and their clinicians, it is imperative to have a way to calculate this risk and start clinical interventions to slow down or prevent the complications of this condition.
Brief description The study centers on establishing the DiabetIA database, a substantial repository encompassing de-identified electronic health records from 136,674 patients sourced from primary care facilities within the Mexican Institute for Social Security (IMSS). Our efforts involved curating, cleansing, and transforming this extensive dataset, and then employing machine learning models to predict diabetic complications with high accuracy.
### Competing Interest Statement
Authors ALP and PSQA hold shares of Amphora Health. Authors JT, DSQ, MFRM, MANA, EPSF, GGA contributed to the research while employed by Amphora Health. Authors RCM, JGPS, AGG are employed by IMSS. All other authors from academic institutions declare no conflict of interest.
### Funding Statement
This project received partial funding from participating institutions and the National Council of Science, Humanities, and Technology (CONAHCyT) through its Institutional Fund for Regional Development for Scientific, Technological, and Innovation Development (FORDECyT) under the National Strategic Programs (ProNacEs), registered under number 10410.
### Author Declarations
I confirm all relevant ethical guidelines have been followed, and any necessary IRB and/or ethics committee approvals have been obtained.
Yes
The details of the IRB/oversight body that provided approval or exemption for the research described are given below:
This study was approved by the Ethics and Research Committees from the Mexican Institute of Social Security (IMSS), which are certified as Institutional Review Board (IRB) in accordance with the Mexican regulation, under protocol numbers R-2018-785-051. Since, this was a retrospective study and the author AGG and RCM carried out the anonymization of data, the IRB waived the need for consent for this study.
I confirm that all necessary patient/participant consent has been obtained and the appropriate institutional forms have been archived, and that any patient/participant/sample identifiers included were not known to anyone (e.g., hospital staff, patients or participants themselves) outside the research group so cannot be used to identify individuals.
Yes
I understand that all clinical trials and any other prospective interventional studies must be registered with an ICMJE-approved registry, such as ClinicalTrials.gov. I confirm that any such study reported in the manuscript has been registered and the trial registration ID is provided (note: if posting a prospective study registered retrospectively, please provide a statement in the trial ID field explaining why the study was not registered in advance).
Yes
I have followed all appropriate research reporting guidelines, such as any relevant EQUATOR Network research reporting checklist(s) and other pertinent material, if applicable.
Yes
The data that supports the findings of this study is available for research purposes at the National Informatics Ecosystem under its Health chapter (ENI Salud), which can be freely accessed at:
MoreTranslated text
Key words
diabetes,machine learning models,learning models,machine learning
AI Read Science
Must-Reading Tree
Example
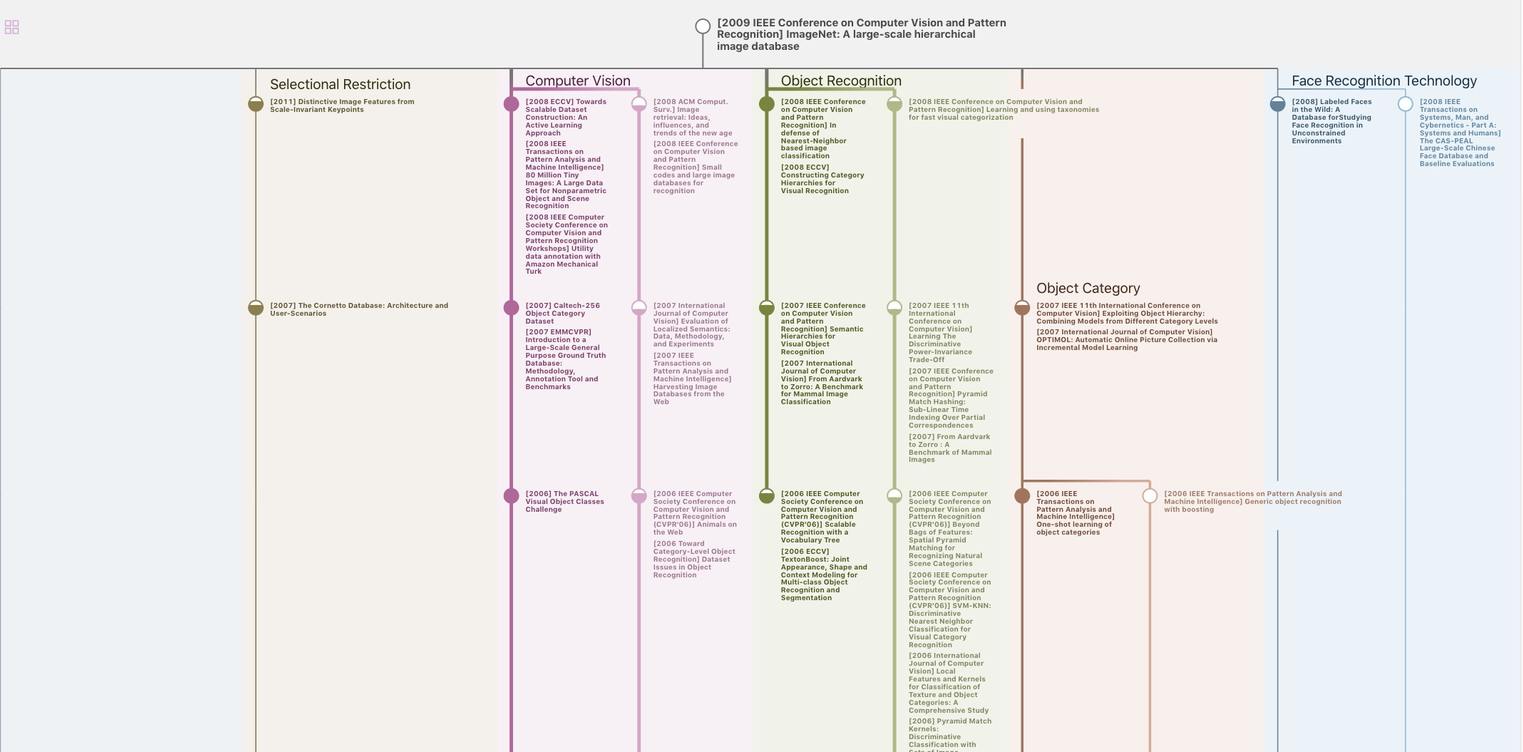
Generate MRT to find the research sequence of this paper
Chat Paper
Summary is being generated by the instructions you defined