The Value of Ensemble Learning Model Based on Conventional Non-Contrast MRI in the Pathological Grading of Cervical Cancer
CMMCA@MICCAI(2023)
摘要
Purpose: To investigate the value of an stacking ensemble learning model based on conventional non-enhanced MRI sequences in the pathological grading of cervical cancer. Methods: We retrospectively included 98 patients with cervical cancer (54 well/moderately differentiated and 44 poorly differentiated). Radiomics features were extracted from T2WI Axi and T2WI Sag. Feature selection was performed by intra-class correlation coefficients (ICC), t-test, least absolute shrinkage and selection operator (LASSO). Logistic Regression (LR), Support Vector Machine (SVM), k-Nearest Neighbor (kNN), and Extreme Gradient Boosting (XGB) were used as the first-layer base classifier, and LR as the second-layer meta-classifier in stacking ensemble learning model. The model performance was evaluated by the area under the curve (AUC) and accuracy. Results: In the basic classifiers, the XGB model showed the best performance, the average AUC was 0.74(0.69,0.76) and the accuracy was 0.73. It was followed by SVM, LR and KNN models, and the average AUC were 0.73(0.66,0.80), 0.71(0.62,0.78) and 0.66(0.61,0.72), respectively. The performance of stacking ensemble model showed effective improvement, with an average AUC of 0.77(0.67,0.84), and the accuracy was 0.83. Conclusions: The ensemble learning model based on conventional non-enhanced MRI sequences could identify poorly differentiated cervical cancer from well/moderately differentiated cervical cancer, and can provide more references for preoperative non-invasive assessment of cervical cancer.
更多查看译文
关键词
Cervical cancer,Grade,Radiomics,Ensemble learning,Magnetic resonance imaging
AI 理解论文
溯源树
样例
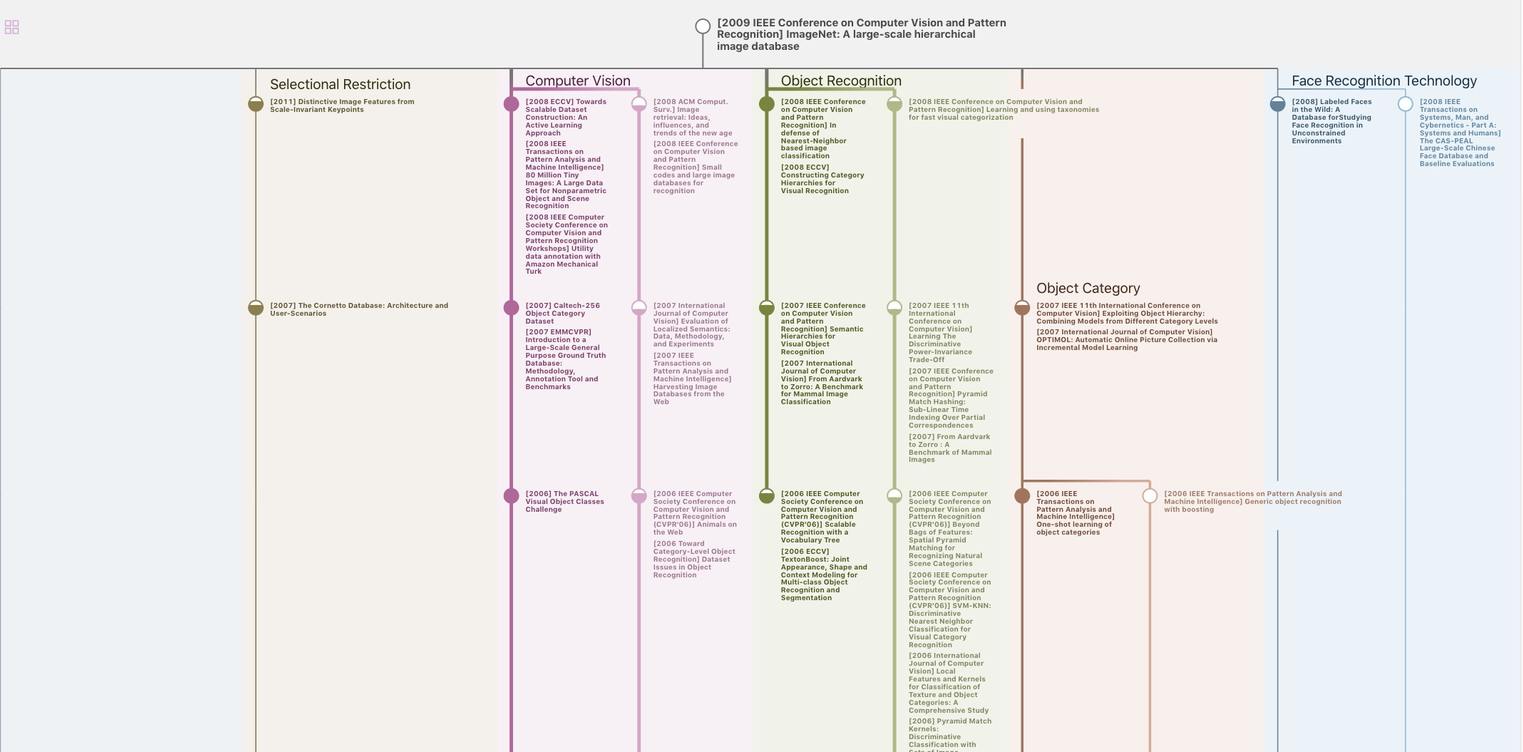
生成溯源树,研究论文发展脉络
Chat Paper
正在生成论文摘要