Beating Gradient Boosting: Target-Guided Binning for Massively Scalable Classification in Real-Time.
Annals of Computer Science and Information Systems(2023)
摘要
Gradient Boosting (GB) consistently outperforms other ML predictors especially in the context of binary classification based on multi-modal data of different forms and types. Its newest efficient implementations, including XGBoost, LGBM and CATBoost, push GB even further ahead with fast GPU-accelerated compute engine and optimized handling of categorical features. In an attempt to beat GB in both the performance and processing speed we propose a new simple yet fast and robust classification model based on predictive binning. At first all features undergo massively parallelized binning into a unified ordinally compressed risk representation, independently optimized to maximize the AUC score against the target. The resultant array of summarized micro-predictors, resembling 0-depth decision trees, directly expressing oridnally represented target risk, are then passed through the greedy feature selection to compose a robust wide-margin voting classifier, whose performance can beat GB while the extreme build and execution speed along with highly compressed representation welcomes extreme data sizes and realtime applicability. The model has been applied to detect cyber-security attacks on IoT devices within FedCSIS‘2023 Challenge and scored 2 nd place with the AUC ≈ 1, leaving behind all the latest GB variants in performance and speed.
更多查看译文
AI 理解论文
溯源树
样例
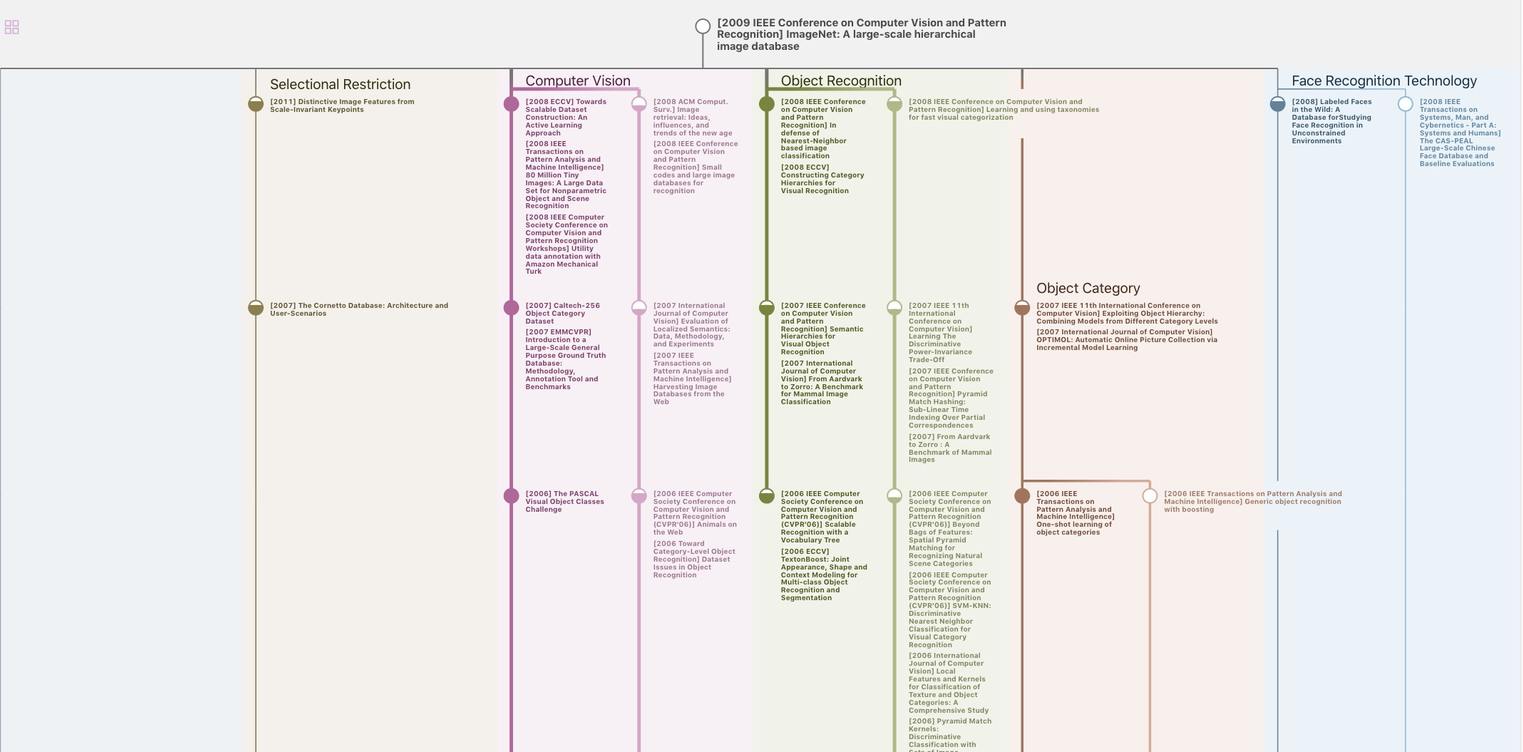
生成溯源树,研究论文发展脉络
Chat Paper
正在生成论文摘要