Topology-Preserving Computed Tomography Super-Resolution Based on Dual-Stream Diffusion Model
MEDICAL IMAGE COMPUTING AND COMPUTER ASSISTED INTERVENTION, MICCAI 2023, PT X(2023)
摘要
X-ray computed tomography (CT) is indispensable for modern medical diagnosis, but the degradation of spatial resolution and image quality can adversely affect analysis and diagnosis. Although super-resolution (SR) techniques can help restore lost spatial information and improve imaging resolution for low-resolution CT (LRCT), they are always criticized for topology distortions and secondary artifacts. To address this challenge, we propose a dual-stream diffusion model for super-resolution with topology preservation and structure fidelity. The diffusion model employs a dual-stream structure-preserving network and an imaging enhancement operator in the denoising process for image information and structural feature recovery. The imaging enhancement operator can achieve simultaneous enhancement of vascular and blob structures in CT scans, providing the structure priors in the super-resolution process. The final super-resolved CT is optimized in both the convolutional imaging domain and the proposed vascular structure domain. Furthermore, for the first time, we constructed an ultra-high resolution CT scan dataset with a spatial resolution of 0.34 x 0.34 mm(2) and an image size of 1024x1024 as a super-resolution training set. Quantitative and qualitative evaluations show that our proposed model can achieve comparable information recovery and much better structure fidelity compared to the other state-of-the-art methods. The performance of high-level tasks, including vascular segmentation and lesion detection on super-resolved CT scans, is comparable to or even better than that of raw HRCT. The source code is publicly available at https://github.com/Arturia-Pendragon-Iris/UHRCT_SR.
更多查看译文
关键词
Computed tomography,Super resolution,Diffusion model,Image enhancement
AI 理解论文
溯源树
样例
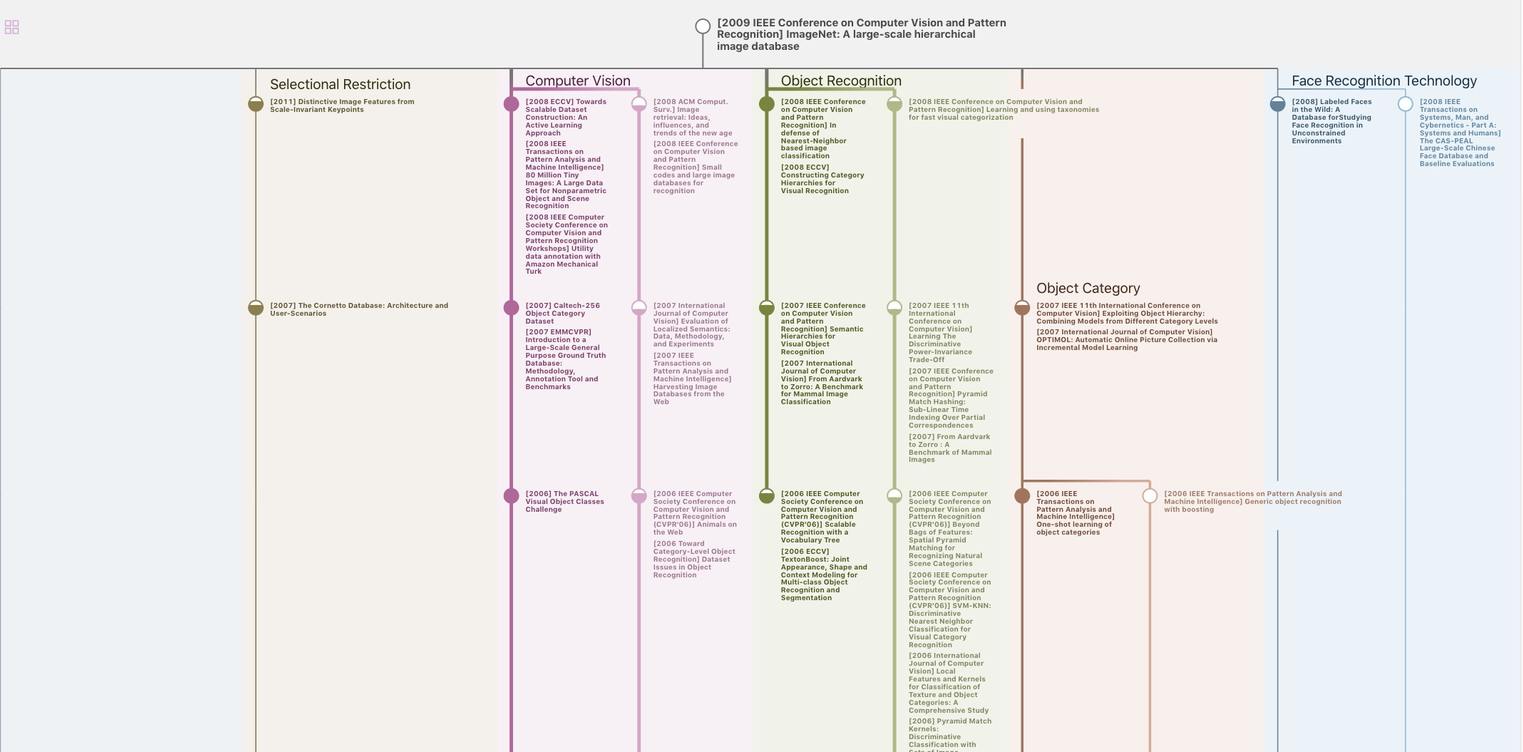
生成溯源树,研究论文发展脉络
Chat Paper
正在生成论文摘要