Examining User Heterogeneity in Digital Experiments.
ACM Trans. Inf. Syst.(2023)
摘要
Digital experiments are routinely used to test the value of a treatment relative to a status-quo control setting-for instance, a new search relevance algorithm for a website or a new results layout for a mobile app. As digital experiments have become increasingly pervasive in organizations and a wide variety of research areas, their growth has prompted a new set of challenges for experimentation platforms. One challenge is that experiments often focus on the average treatment effect (ATE) without explicitly considering differences across major sub-groups: heterogeneous treatment effect (HTE). This is especially problematic, because ATEs have decreased in many organizations as the more obvious benefits have already been realized. However, questions abound regarding the pervasiveness of user HTEs and how best to detect them. We propose a framework for detecting and analyzing user HTEs in digital experiments. Our framework combines an array of user characteristics with double machine learning. Analysis of 27 real-world experiments spanning 1.76 billion sessions and simulated data demonstrates the effectiveness of our detection method relative to existing techniques. We also find that transaction, demographic, engagement, satisfaction, and lifecycle characteristics exhibit statistically significant HTEs in 10% to 20% of our real-world experiments, underscoring the importance of considering user heterogeneity when analyzing experiment results; otherwise, personalized features and experiences cannot happen, thus reducing effectiveness. In terms of the number of experiments and user sessions, we are not aware of any study that has examined user HTEs at this scale. Our findings have important implications for information retrieval, user modeling, platforms, and digital experience contexts, in which online experiments are often used to evaluate the effectiveness of design artifacts.
更多查看译文
关键词
user heterogeneity,experiments
AI 理解论文
溯源树
样例
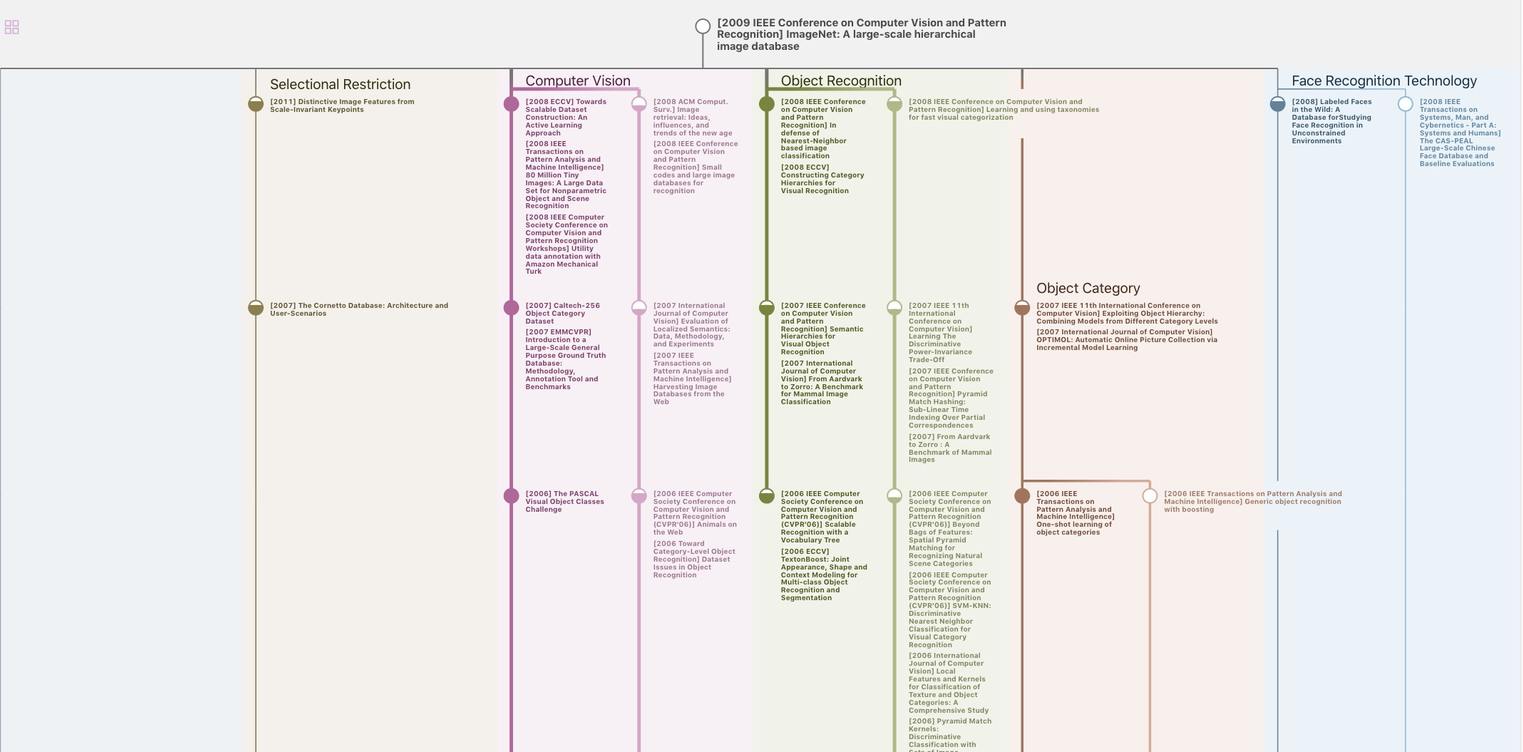
生成溯源树,研究论文发展脉络
Chat Paper
正在生成论文摘要