Concentration for Multiplier Empirical Processes with Dependent Weights
AIMS MATHEMATICS(2023)
摘要
A novel concentration inequality for the sum of independent sub-Gaussian variables with random dependent weights is introduced in statistical settings for high-dimensional data. The random dependent weights are functions of some regularized estimators. We applied the proposed concentration inequality to obtain a high probability bound for the stochastic Lipschitz constant for negative binomial loss functions involved in Lasso-penalized negative binomial regressions. We used this bound to study oracle inequalities for Lasso estimators. Additionally, a similar concentration inequality was derived for a randomly weighted sum of independent centred exponential family variables.
更多查看译文
关键词
high-dimensional data,multiplier empirical processes,sub-gaussian distributions,statistical inference
AI 理解论文
溯源树
样例
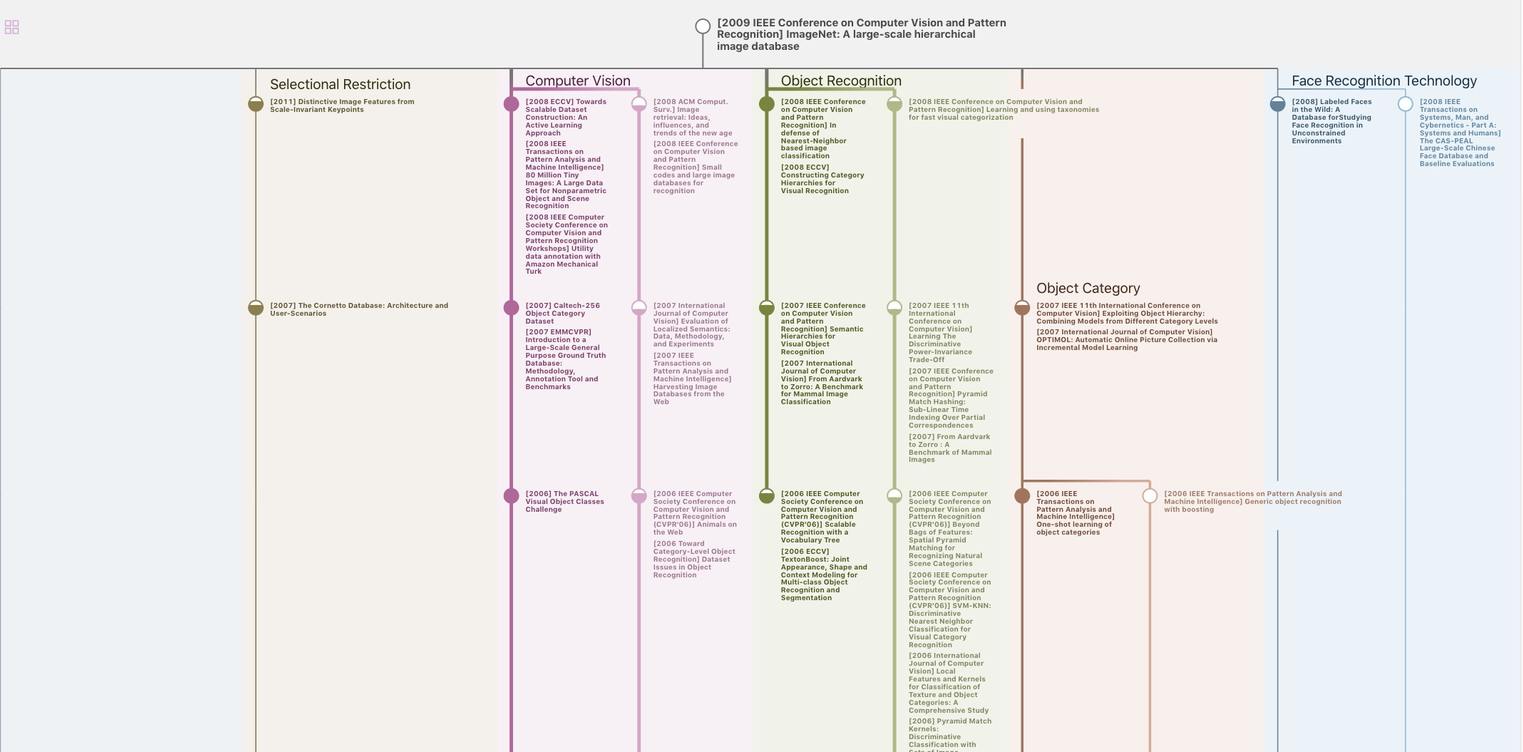
生成溯源树,研究论文发展脉络
Chat Paper
正在生成论文摘要