Attention or Convolution: Transformer Encoders in Audio Language Models for Inference Efficiency
CoRR(2023)
摘要
In this paper, we show that a simple self-supervised pre-trained audio model
can achieve comparable inference efficiency to more complicated pre-trained
models with speech transformer encoders. These speech transformers rely on
mixing convolutional modules with self-attention modules. They achieve
state-of-the-art performance on ASR with top efficiency. We first show that
employing these speech transformers as an encoder significantly improves the
efficiency of pre-trained audio models as well. However, our study shows that
we can achieve comparable efficiency with advanced self-attention solely. We
demonstrate that this simpler approach is particularly beneficial with a
low-bit weight quantization technique of a neural network to improve
efficiency. We hypothesize that it prevents propagating the errors between
different quantized modules compared to recent speech transformers mixing
quantized convolution and the quantized self-attention modules.
更多查看译文
关键词
audio language models,transformer encoders,language models,attention
AI 理解论文
溯源树
样例
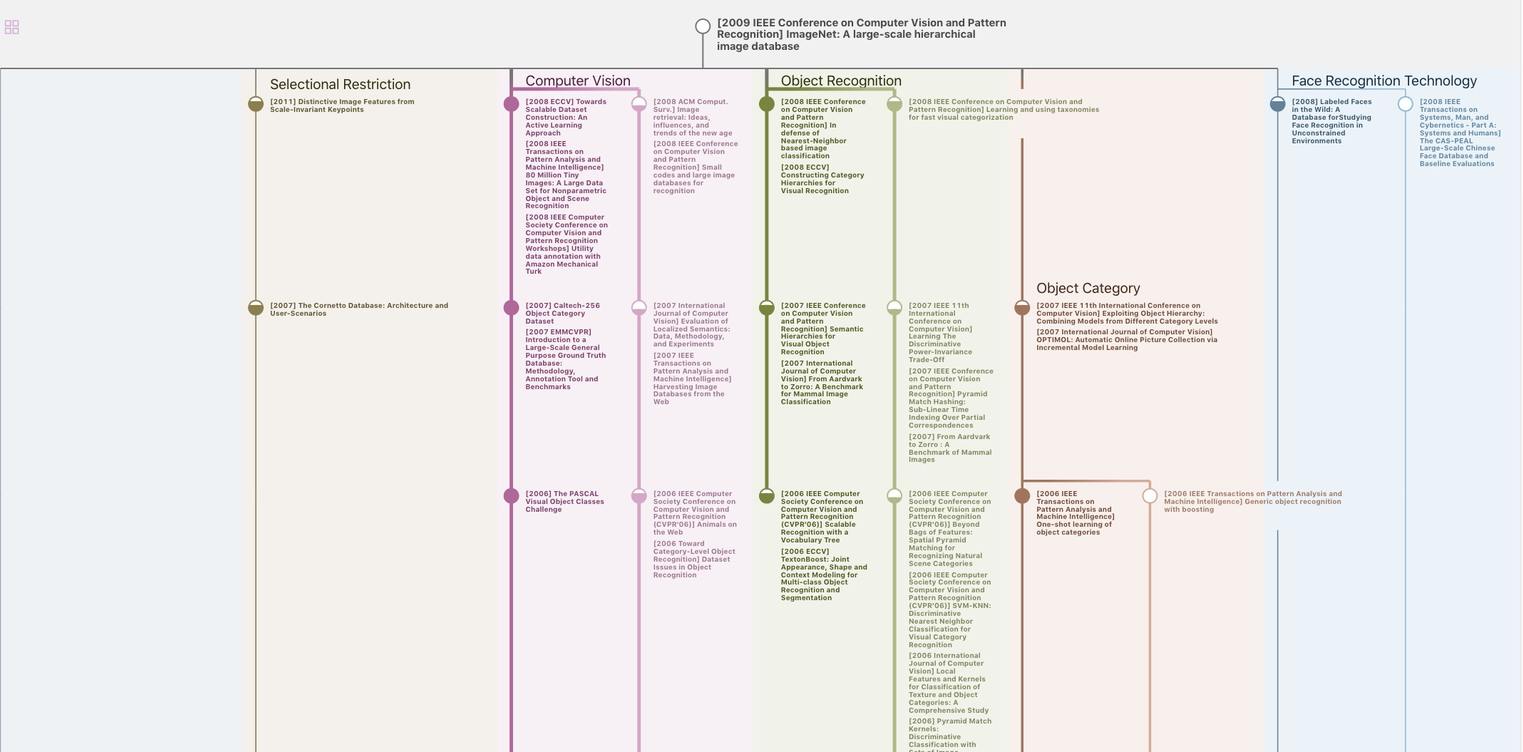
生成溯源树,研究论文发展脉络
Chat Paper
正在生成论文摘要