EslaXDET: A new X-ray baggage security detection framework based on self-supervised vision transformers
ENGINEERING APPLICATIONS OF ARTIFICIAL INTELLIGENCE(2024)
Abstract
Deep learning-based X-ray detection of hazardous materials is crucial for public safety, as it can automatically detect them in baggage. However, most existing algorithms for X-ray detection rely on supervised learning, necessitating large amounts of labeled data for training. Obtaining such data is challenging due to the unique nature of X-ray safety images, requiring significant time and money investment from trained staff for accurate labeling. In this paper, we propose a new X-ray dangerous goods detection framework called EslaXDET, whose backbone is trained by a hybrid Self-Supervised Learning (SSL) strategy and whose detection head is designed for a non-multistage backbone. First, the hybrid strategy named ESLA is an abbreviation for Enhanced Self-supervised Learning with masked Autoencoders, which does not require the involvement of labels as it is derived from a hybrid architecture of Contrastive Learning (CL) and Masked Image Model (MIM). Then, the detection head, called Head-Tail Feature Pyramid (HTFP), creates multi-level feature maps comparable to that produced by a multi-level backbone structure by downsampling the output feature of the last stage of the plain Vision Transformers (ViT) multiple times. Finally, the experimental results show that with the backbone of ViT-B, the top-1 accuracy of ESLA on the ImageNet-1K dataset is 77.2%, which is 3% higher than BYOL. Moreover, the average precision (AP, the same evaluation metrics as COCO) of EslaXDET on the PIDray dataset is 69.2%, nearly 8% higher than the original method SDANet, providing a new idea for the subsequent SSL-based X-ray baggage security detection method.
MoreTranslated text
Key words
X-ray security,Self-supervised learning,MAE,Object detection,Contrastive learning
AI Read Science
Must-Reading Tree
Example
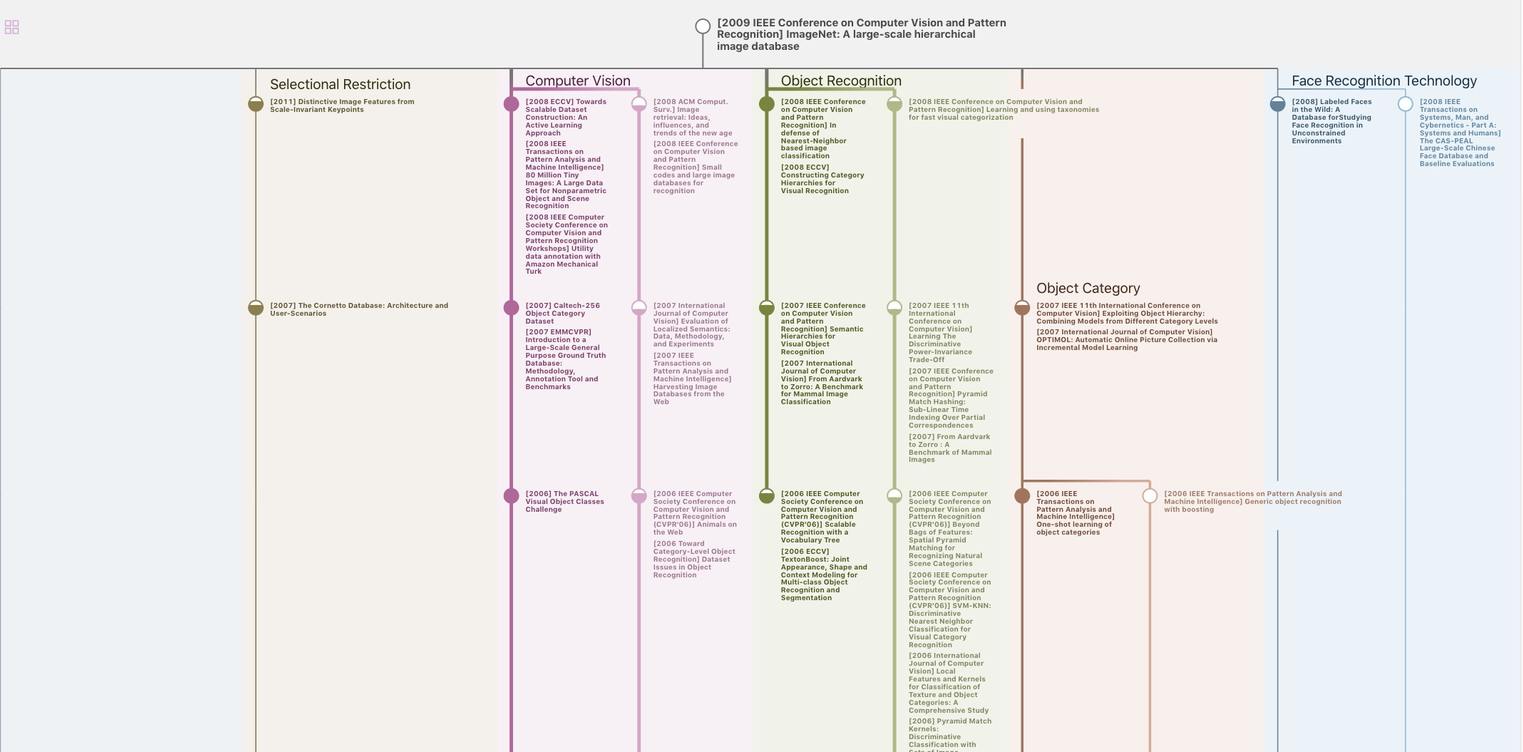
Generate MRT to find the research sequence of this paper
Chat Paper
Summary is being generated by the instructions you defined