Graph attention network with Granger causality map for fault detection and root cause diagnosis
COMPUTERS & CHEMICAL ENGINEERING(2024)
Abstract
Unsupervised data-driven methods are widely used for fault detection and diagnosis in modern industrial processes. However, accurately distinguishing faults from normal feedback control system adjustments and promptly identifying their root causes are among unresolved challenges. To address these issues, we propose a neural network model consisting of one-dimensional convolutional neural networks and a graph attention network (CNN-GAT) that uses a causal map derived from fault-free data using conditional Granger causality analysis. The CNN-GAT model produces a monitoring index that accurately reflects the operating conditions of the process and distinguishes faults from normal control adjustments. Using the causal map and prediction results from the CNN-GAT model, the root cause diagnosis can be performed promptly after faults are detected, providing operators with more time to address the fault. We demonstrate the performance of the proposed framework using the benchmark Tennessee Eastman process case studies and through comparison with other fault detection methods.
MoreTranslated text
Key words
Graph attention network,Fault detection,Root cause diagnosis,Conditional Granger causality analysis
AI Read Science
Must-Reading Tree
Example
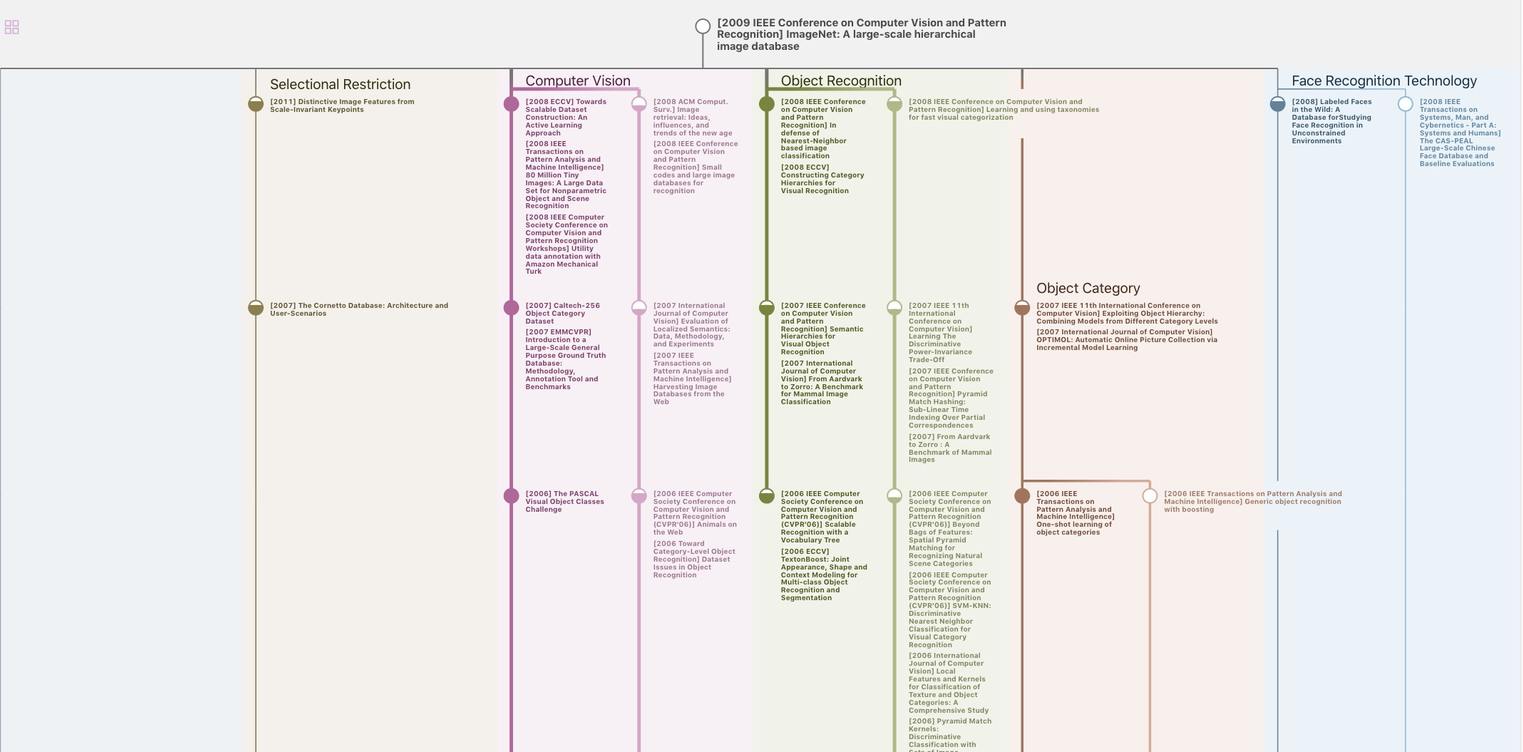
Generate MRT to find the research sequence of this paper
Chat Paper
Summary is being generated by the instructions you defined