Style-Controllable Generalized Person Re-identification
Proceedings of the 31st ACM International Conference on Multimedia(2023)
摘要
Domain generalizable person Re-identification is a challenging and realistic task. It requires a model to train on multi-source domains and then generalizes well on unseen target domains. Existing approaches typically mix images from different domains in a mini-batch for training, but this can increase discrimination within a mini-batch due to the vast style differences among domains. As a result, the model may converge easily by mining domain-related information, while neglecting identity-discriminative information, especially for metric learning. To improve the difficulty of metric learning under multi-source training, we design a Style-aware Hard-negative Sampling (SHS) strategy. SHS effectively improves metric learning but reduces the style diversity within the batch. To enhance style diversity, we devise a Dynamic Style Mixing (DSM) which memorizes single-domain styles and synthesizes novel styles, which largely raises the diversity of source domains. Extensive experiments prove the effectiveness of our method. In both single-source and multi-source settings, our approach significantly outperforms the state-of-the-art (SOTA).
更多查看译文
关键词
Person Re-identification,domain generalization,metric learning
AI 理解论文
溯源树
样例
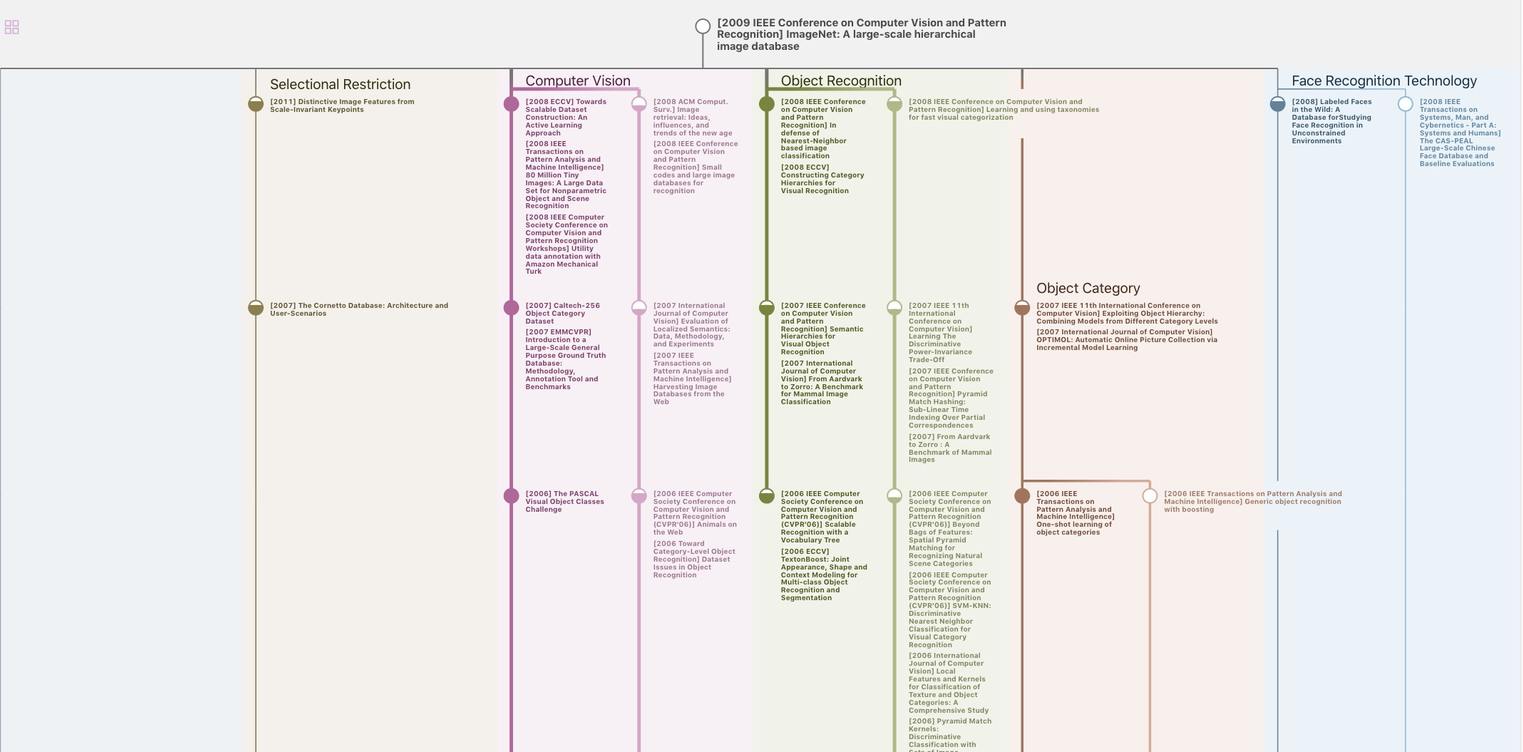
生成溯源树,研究论文发展脉络
Chat Paper
正在生成论文摘要