FedVQA: Personalized Federated Visual Question Answering over Heterogeneous Scenes
MM '23: Proceedings of the 31st ACM International Conference on Multimedia(2023)
摘要
This paper presents a new setting for visual question answering (VQA) called personalized federated VQA (FedVQA) that addresses the growing need for decentralization and data privacy protection. FedVQA is both practical and challenging, requiring clients to learn well-personalized models on scene-specific datasets with severe feature/label distribution skews. These models then collaborate to optimize a generic global model on a central server, which is desired to generalize well on both seen and unseen scenes without sharing raw data with the server and other clients. The primary challenge of FedVQA is that, client models tend to forget the global knowledge initialized from central server during the personalized training, which impairs their personalized capacity due to the potential overfitting issue on local data. This further leads to divergence issues when aggregating distinct personalized knowledge at the central server, resulting in an inferior generalization ability on unseen scenes. To address the challenge, we propose a novel federated pairwise preference preserving (FedP3) framework to improve personalized learning via preserving generic knowledge under FedVQA constraints. Specifically, we first design a differentiable pairwise preference (DPP) to improve knowledge preserving by formulating a flexible yet effective global knowledge. Then, we introduce a forgotten-knowledge filter (FKF) to encourage the client models to selectively consolidate easily-forgotten knowledge. By aggregating the DPP and the FKF, FedP3 coordinates the generic and the personalized knowledge to enhance the personalized ability of clients and generalizability of the server. Extensive experiments show that FedP3 consistently surpasses the state-of-the-art in FedVQA task.
更多查看译文
AI 理解论文
溯源树
样例
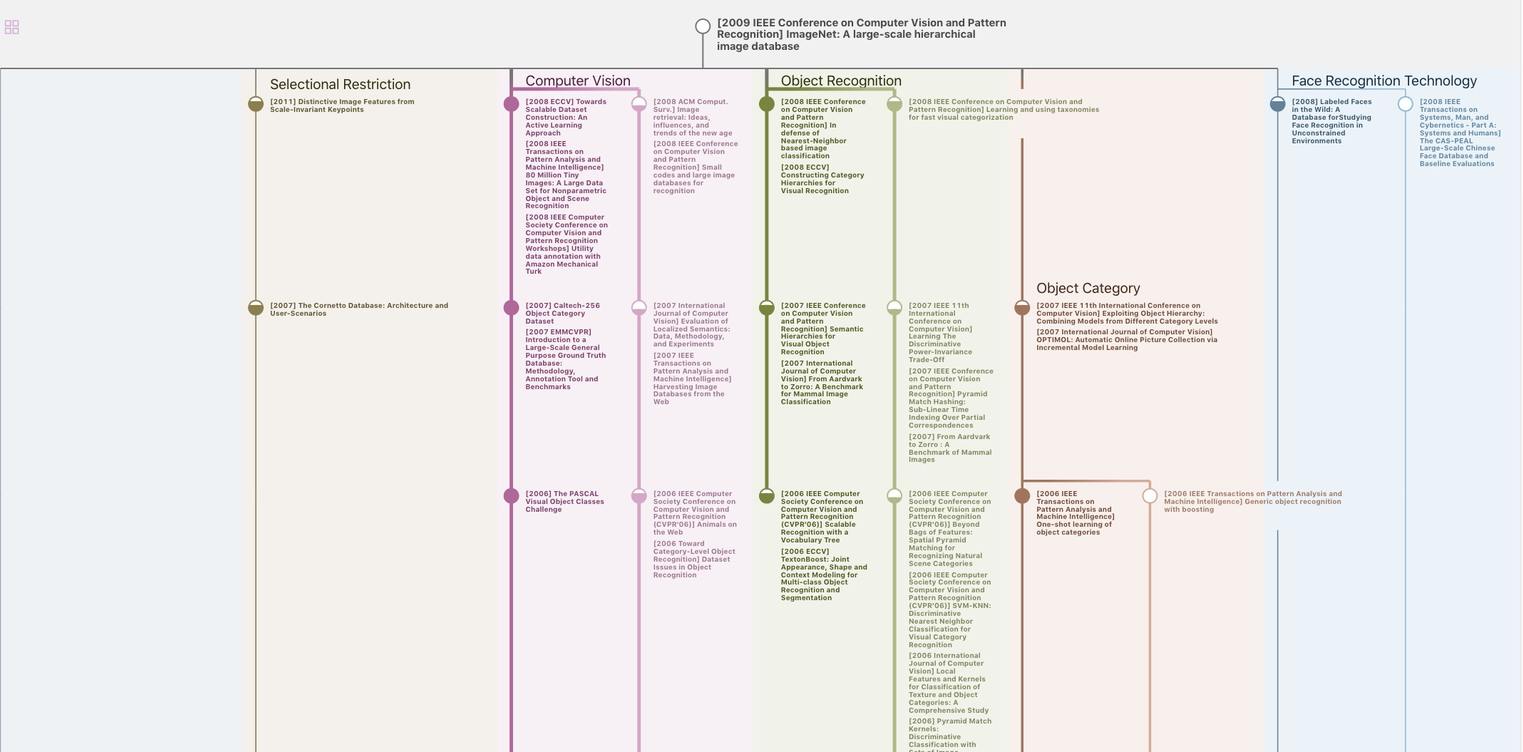
生成溯源树,研究论文发展脉络
Chat Paper
正在生成论文摘要