Mask-Guided Progressive Network for Joint Raindrop and Rain Streak Removal in Videos
MM '23: Proceedings of the 31st ACM International Conference on Multimedia(2023)
摘要
Videos captured in rainy weather are unavoidably corrupted by both rain streaks and raindrops in driving scenarios, and it is desirable and challenging to recover background details obscured by rain streaks and raindrops. However, existing video rain removal methods often address either video rain streak removal or video raindrop removal, thereby suffer from degraded performance when deal with both simultaneously. The bottleneck is a lack of a video dataset, where each video frame contains both rain streaks and raindrops. To address this issue, we in this work generate a synthesized dataset, namely VRDS, with 102 rainy videos from diverse scenarios, and each video frame has the corresponding rain streak map, raindrop mask, and the underlying rain-free clean image (ground truth). Moreover, we devise a mask-guided progressive video deraining network (ViMP-Net) to remove both rain streaks and raindrops of each video frame. Specifically, we develop an intensity-guided alignment block to predict the rain streak intensity map and remove the rain streaks of the input rainy video at the first stage. Then, we predict a raindrop mask and pass it into a devised mask-guided dual transformer block to learn inter-frame and intra-frame transformer features, which are then fed into a decoder for further eliminating raindrops. Experimental results demonstrate that our ViMP-Net outperforms state-of-the-art methods on our synthetic dataset and real-world rainy videos. Our code is available at https://github.com/TonyHongtaoWu/ViMP-Net.
更多查看译文
AI 理解论文
溯源树
样例
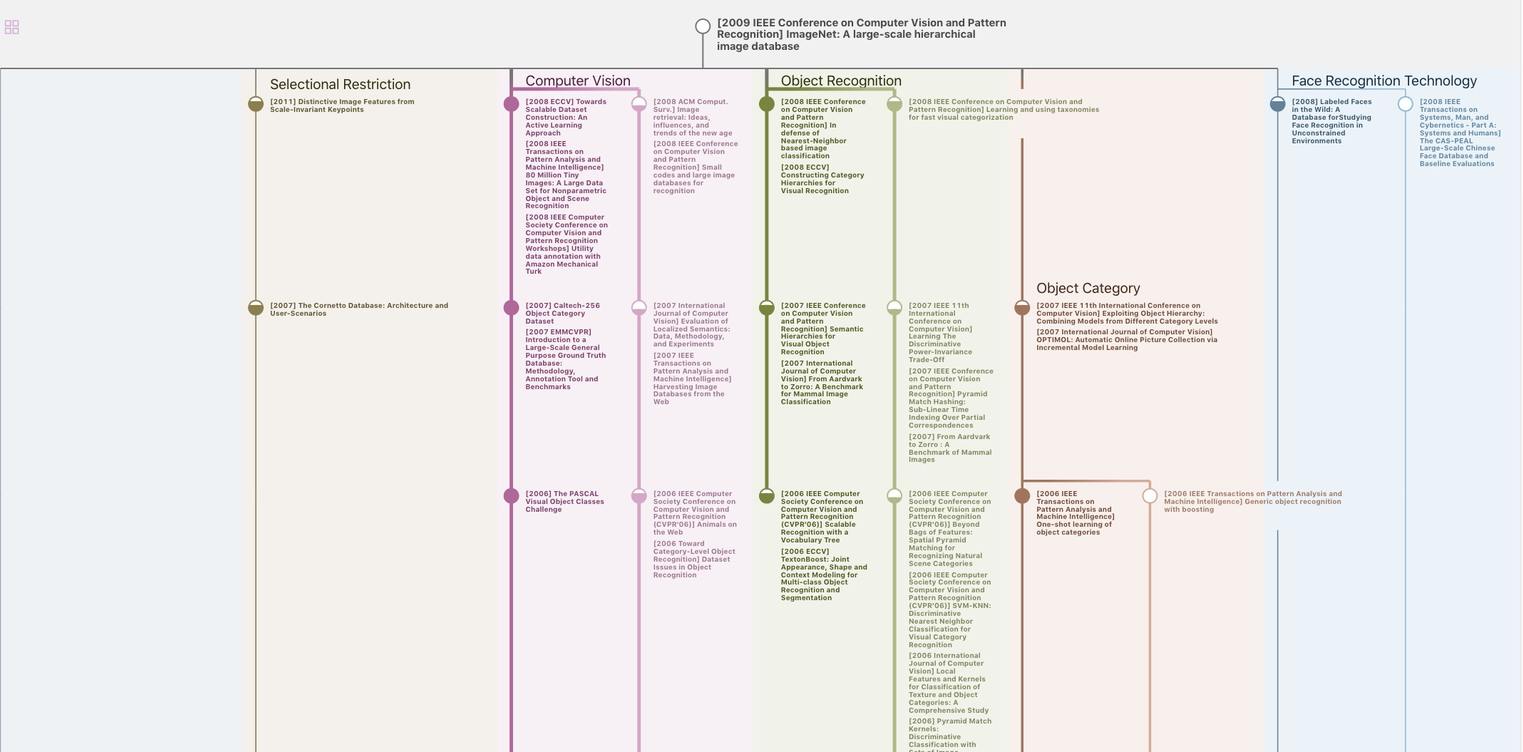
生成溯源树,研究论文发展脉络
Chat Paper
正在生成论文摘要