SemanticRT: A Large-Scale Dataset and Method for Robust Semantic Segmentation in Multispectral Images
MM '23: Proceedings of the 31st ACM International Conference on Multimedia(2023)
摘要
Growing interests in multispectral semantic segmentation (MSS) have been witnessed in recent years, thanks to the unique advantages of combining RGB and thermal infrared images to tackle challenging scenarios with adverse conditions. However, unlike traditional RGB-only semantic segmentation, the lack of a large-scale MSS dataset has become a hindrance to the progress of this field. To address this issue, we introduce a SemanticRT dataset - the largest MSS dataset to date, comprising 11,371 high-quality, pixel-level annotated RGB-thermal image pairs. It is 7 times larger than the existing MFNet dataset, and covers a wide variety of challenging scenarios in adverse lighting conditions such as low-light and pitch black. Further, a novel Explicit Complement Modeling (ECM) framework is developed to extract modality-specific information, which is propagated through a robust cross-modal feature encoding and fusion process. Extensive experiments demonstrate the advantages of our approach and dataset over the existing counterparts. Our new dataset may also facilitate further development and evaluation of existing and new MSS algorithms.
更多查看译文
AI 理解论文
溯源树
样例
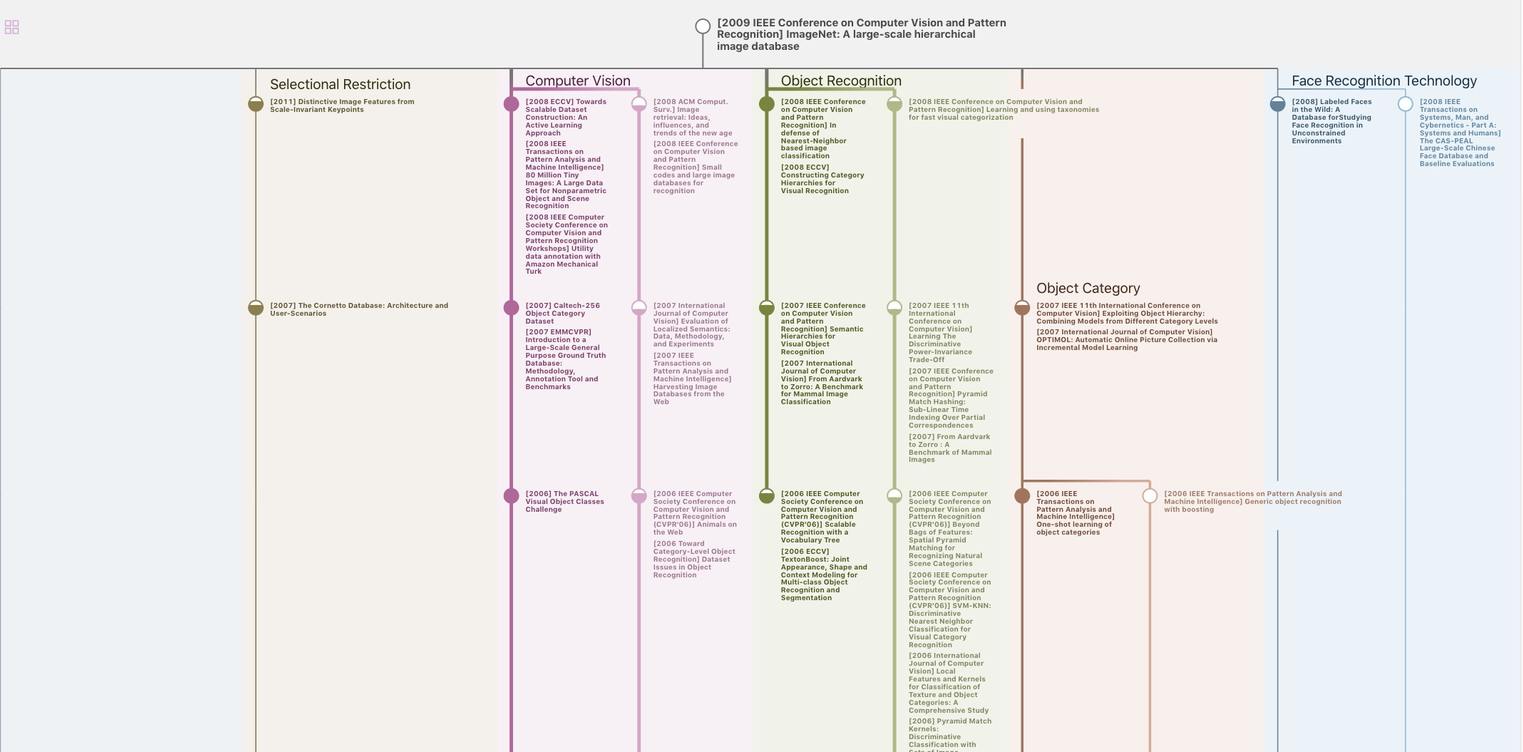
生成溯源树,研究论文发展脉络
Chat Paper
正在生成论文摘要