SmoothHess: ReLU Network Feature Interactions via Stein's Lemma
arXiv (Cornell University)(2023)
摘要
Several recent methods for interpretability model feature interactions by
looking at the Hessian of a neural network. This poses a challenge for ReLU
networks, which are piecewise-linear and thus have a zero Hessian almost
everywhere. We propose SmoothHess, a method of estimating second-order
interactions through Stein's Lemma. In particular, we estimate the Hessian of
the network convolved with a Gaussian through an efficient sampling algorithm,
requiring only network gradient calls. SmoothHess is applied post-hoc, requires
no modifications to the ReLU network architecture, and the extent of smoothing
can be controlled explicitly. We provide a non-asymptotic bound on the sample
complexity of our estimation procedure. We validate the superior ability of
SmoothHess to capture interactions on benchmark datasets and a real-world
medical spirometry dataset.
更多查看译文
关键词
relu network feature interactions,stein
AI 理解论文
溯源树
样例
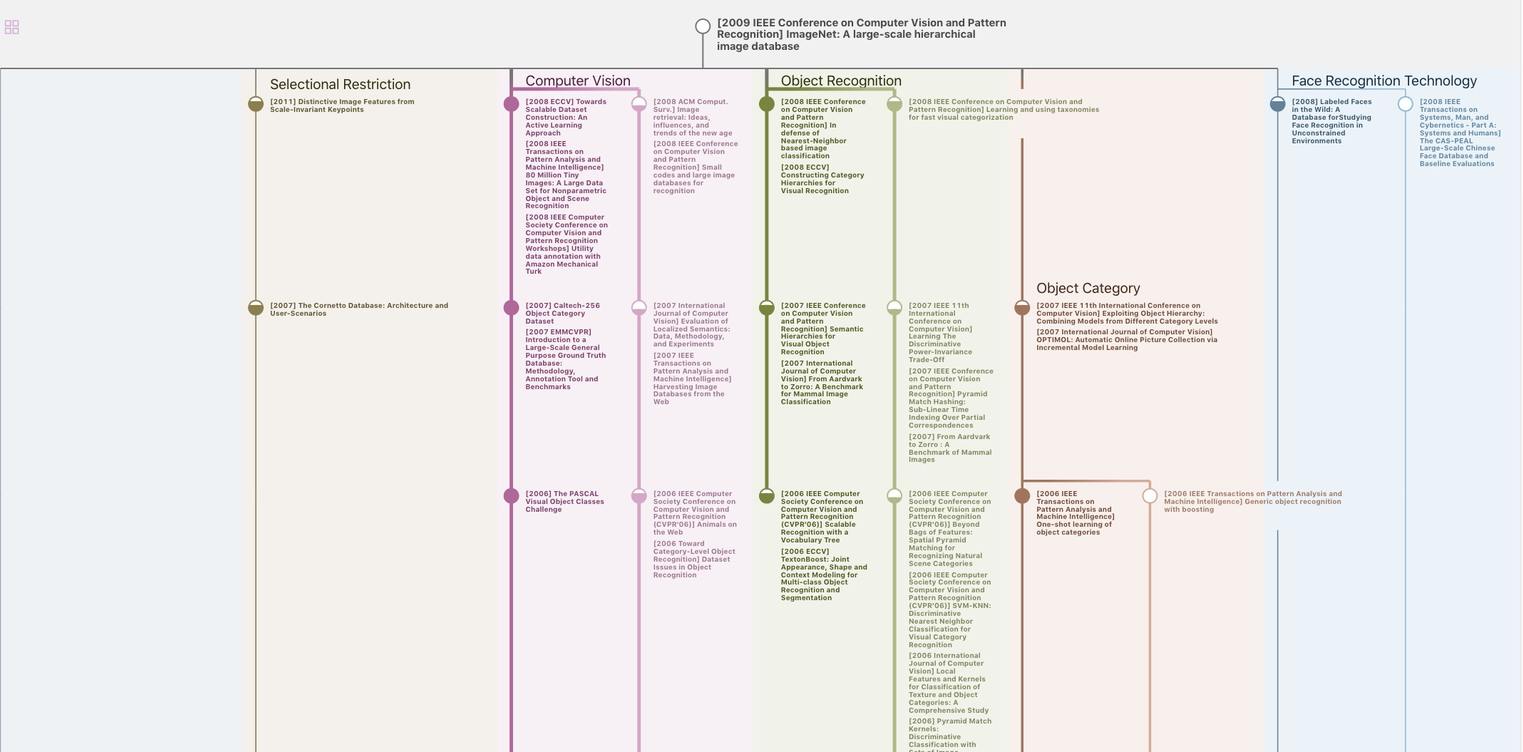
生成溯源树,研究论文发展脉络
Chat Paper
正在生成论文摘要