Boosting Domain-Specific Question Answering Through Weakly Supervised Self-Training
ISI(2023)
摘要
Question answering systems have emerged as an important research area in the field of natural language processing, enabling the provision of accurate answers to user queries in a more efficient and user-friendly manner. These systems have much practical significance especially in security-related applications, where intelligence analysts can easily access pertinent information from diverse sources to accelerate the decision-making process. In the context of security-related scenarios, users tend to interact with a question answering system with specific domain-oriented purposes. However, most existing question answering systems focus on open-domain situations with abundant labeled data as well as structured data, and domain-specific question answering methods are in urgent need. Domain-specific question answering faces the challenge of the low-resource issue caused by data scarcity. To address this challenge, in this paper, we propose a weakly supervised self-training method for domain-specific question answering based on the Retriever-Reader framework. For the retriever module, during the self-training process, we develop two strategies for generating pseudo-labels to augment the labeled dataset, including high confidence sampling and random negative sampling. For the reader module, we adopt the pre-trained language model and fine-tune the generative reader using limited labeled datasets. To evaluate our proposed method, we construct the first Chinese financial question answering dataset of textual document. Experimental results demonstrate that our proposed method can significantly improve the performances of the baseline method through the self-training process.
更多查看译文
关键词
question answering system,Retriever-Reader framework,weakly supervised learning,self-training
AI 理解论文
溯源树
样例
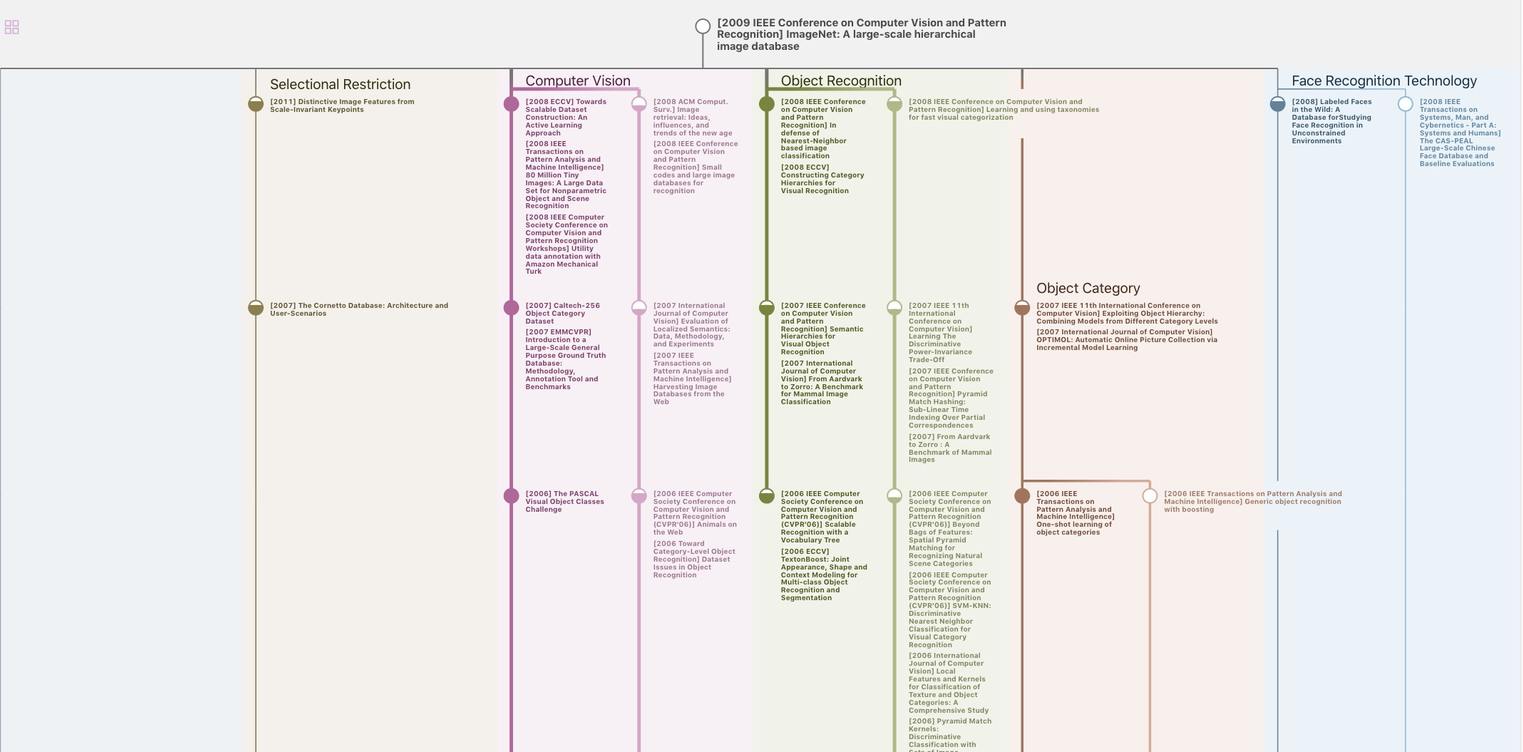
生成溯源树,研究论文发展脉络
Chat Paper
正在生成论文摘要