Exploration and design of Mg alloys for hydrogen storage with supervised machine learning
International Journal of Hydrogen Energy(2023)
摘要
Hydrogen storage is an essential technology for the development of a sustainable energy system. Magnesium (Mg) and its alloys have been identified as promising materials for hydrogen storage due to their high hydrogen storage capacity, low cost, and abundance. However, the use of Mg alloys in hydrogen storage materials requires a thorough understanding of the material properties and their behavior under different conditions. In this study, we established a database of Mg alloys properties and their hydrogen storage performance, which was then used to train various machine learning (ML) regression models with maximum hydrogen storage (Ab_max) and maximum hydrogen release (De_max) as outputs. These models possess excellent prediction accuracy. The GBR model for Ab_max and MLP model for De_max present highest accuracy with the R2 of the test set reaching 0.947 and 0.922, respectively. The Shapley additive explanations (SHAP) algorithm was then used to interpret the best ML models, and the critical values of important descriptors were obtained to guide the design of hydrogen storage materials. Finally, based on the best ML models, the Ab_max and De_max were predicted for the Mg-based binary alloys with other 16 metal elements and Mg–Ni-based ternary alloys with other 15 metal elements, respectively. Among them, 96Mg-4Sm and 95Mg–1Ni-4Sm have higher Ab_max and De_max of 6.31 wt% and 5.69 wt%, 6.64 wt% and 5.63 wt%, respectively, meeting the requirement of high Ab_max/De_max at the operating temperature below 300 °C.
更多查看译文
关键词
Mg-based hydrogen storage, materials, Machine learning, Hydrogen storage property, Feature selection, Interpretable SHAP method
AI 理解论文
溯源树
样例
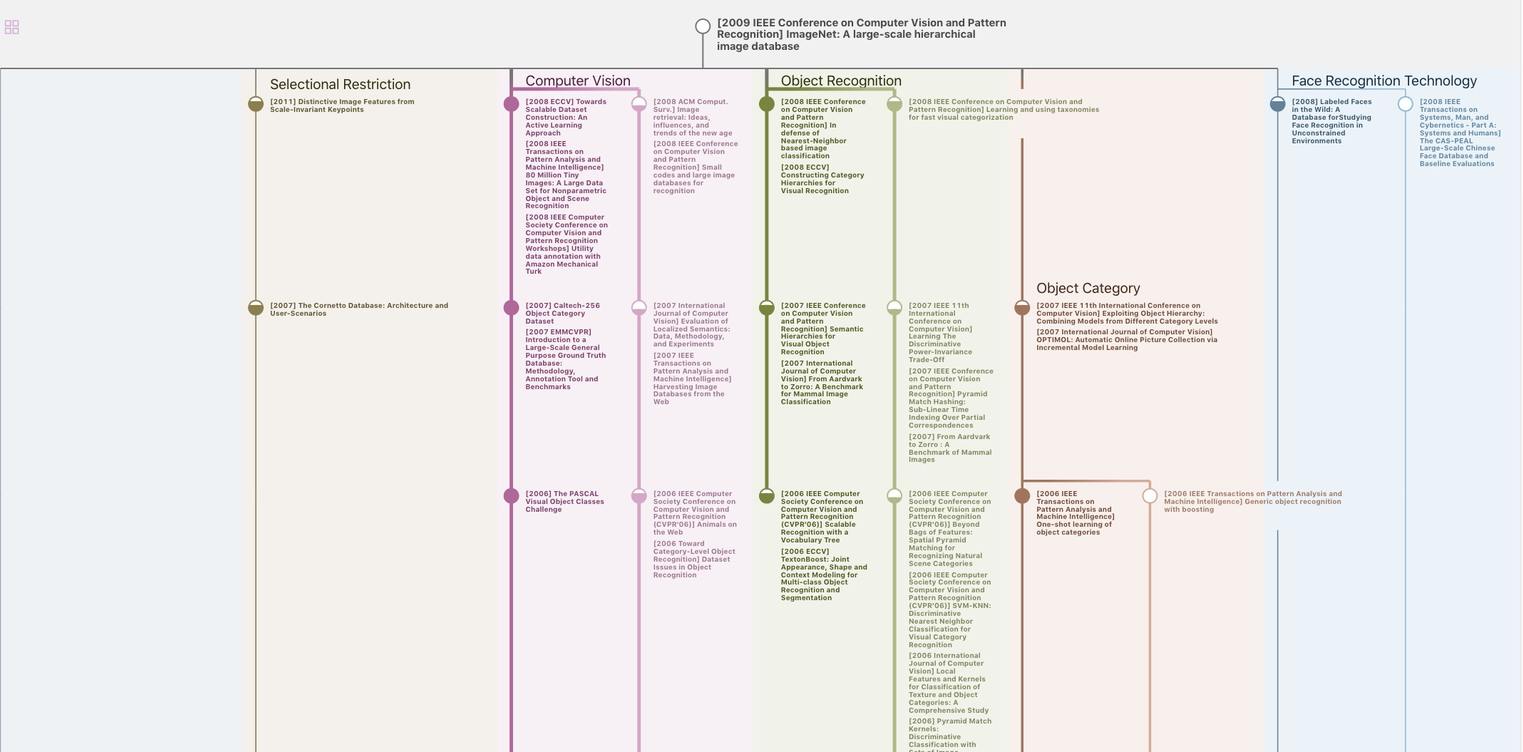
生成溯源树,研究论文发展脉络
Chat Paper
正在生成论文摘要