Large-Scale Deep Learning Medical Image Methodology and Applications Using Multiple GPUs
2023 Seventh International Conference on Advances in Biomedical Engineering (ICABME)(2023)
摘要
Digitalization of medical images has become firmly established in research labs and clinical settings worldwide, and its numerous benefits have helped drive its rapid expansion, which is predicted to continue momentum over the coming years. Its advantages of cutting costs, improving analysis, productivity and patient outcomes, reducing errors, and enhancing imaging and innovation will likely propel its widespread adoption. In recent years digitalization of medical images especially relied on high performance computing. This work illustrates how a large volume of digital radiology data can be analysed in a parallel heterogeneous architecture. We have used TensorFlow (including Keras) and the Horovod API to speed up the learning of Deep Learning models, particularly using GPUs in single and multiple compute nodes. We conclude that using Horovod API with data parallelism shows tremendous speed gains compared to traditional CPU when training the 2D UNet model.
更多查看译文
关键词
Deep Learning,Digital Radiology,UNet,Keras,Horovod,GPU,HPC
AI 理解论文
溯源树
样例
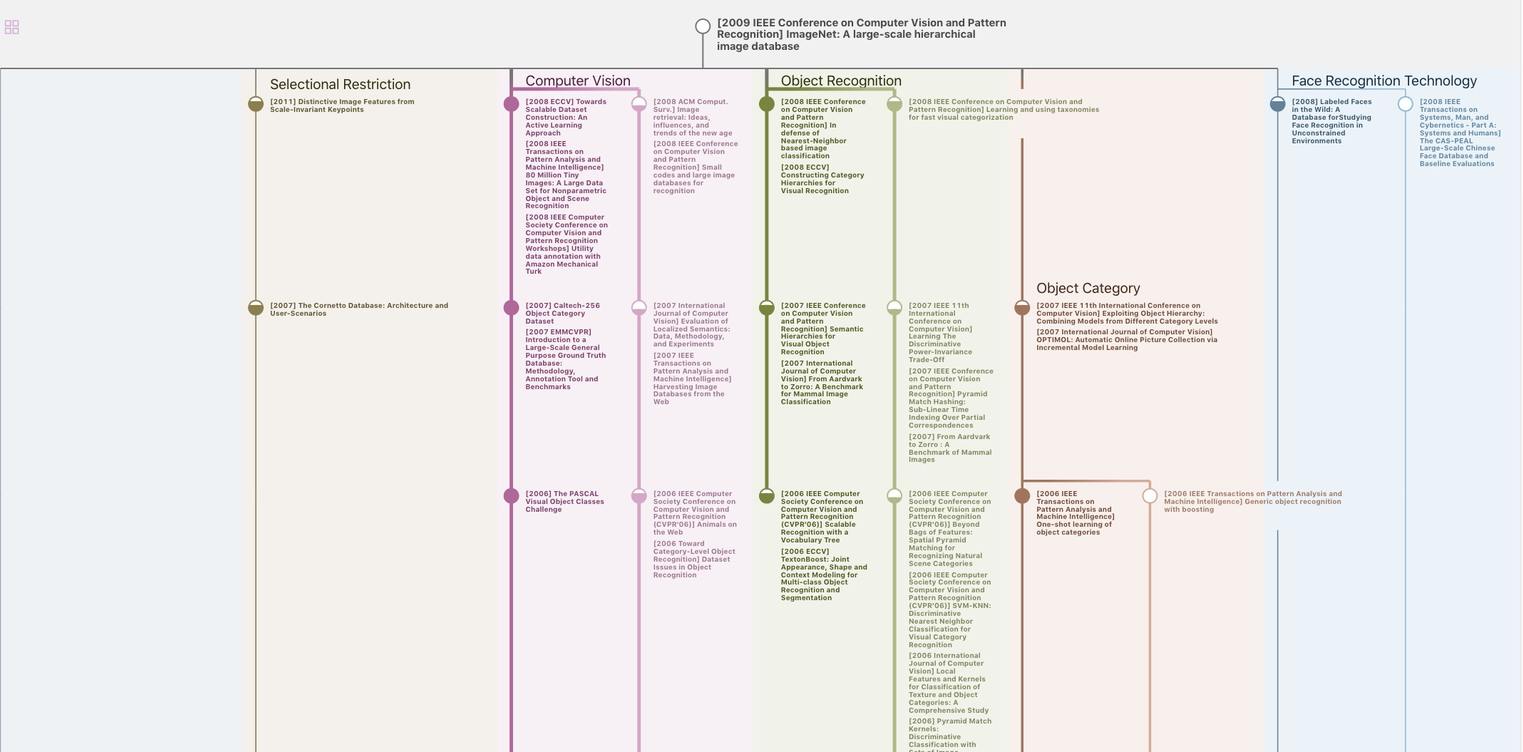
生成溯源树,研究论文发展脉络
Chat Paper
正在生成论文摘要