Low-Dose CT Image Enhancement Using Deep Learning
CoRR(2023)
摘要
The application of ionizing radiation for diagnostic imaging is common around the globe. However, the process of imaging, itself, remains to be a relatively hazardous operation. Therefore, it is preferable to use as low a dose of ionizing radiation as possible, particularly in computed tomography (CT) imaging systems, where multiple x-ray operations are performed for the reconstruction of slices of body tissues. A popular method for radiation dose reduction in CT imaging is known as the quarter-dose technique, which reduces the x-ray dose but can cause a loss of image sharpness. Since CT image reconstruction from directional x-rays is a nonlinear process, it is analytically difficult to correct the effect of dose reduction on image quality. Recent and popular deep-learning approaches provide an intriguing possibility of image enhancement for low-dose artifacts. Some recent works propose combinations of multiple deep-learning and classical methods for this purpose, which over-complicate the process. However, it is observed here that the straight utilization of the well-known U-NET provides very successful results for the correction of low-dose artifacts. Blind tests with actual radiologists reveal that the U-NET enhanced quarter-dose CT images not only provide an immense visual improvement over the low-dose versions, but also become diagnostically preferable images, even when compared to their full-dose CT versions.
更多查看译文
关键词
enhancement,deep learning,ct,low-dose
AI 理解论文
溯源树
样例
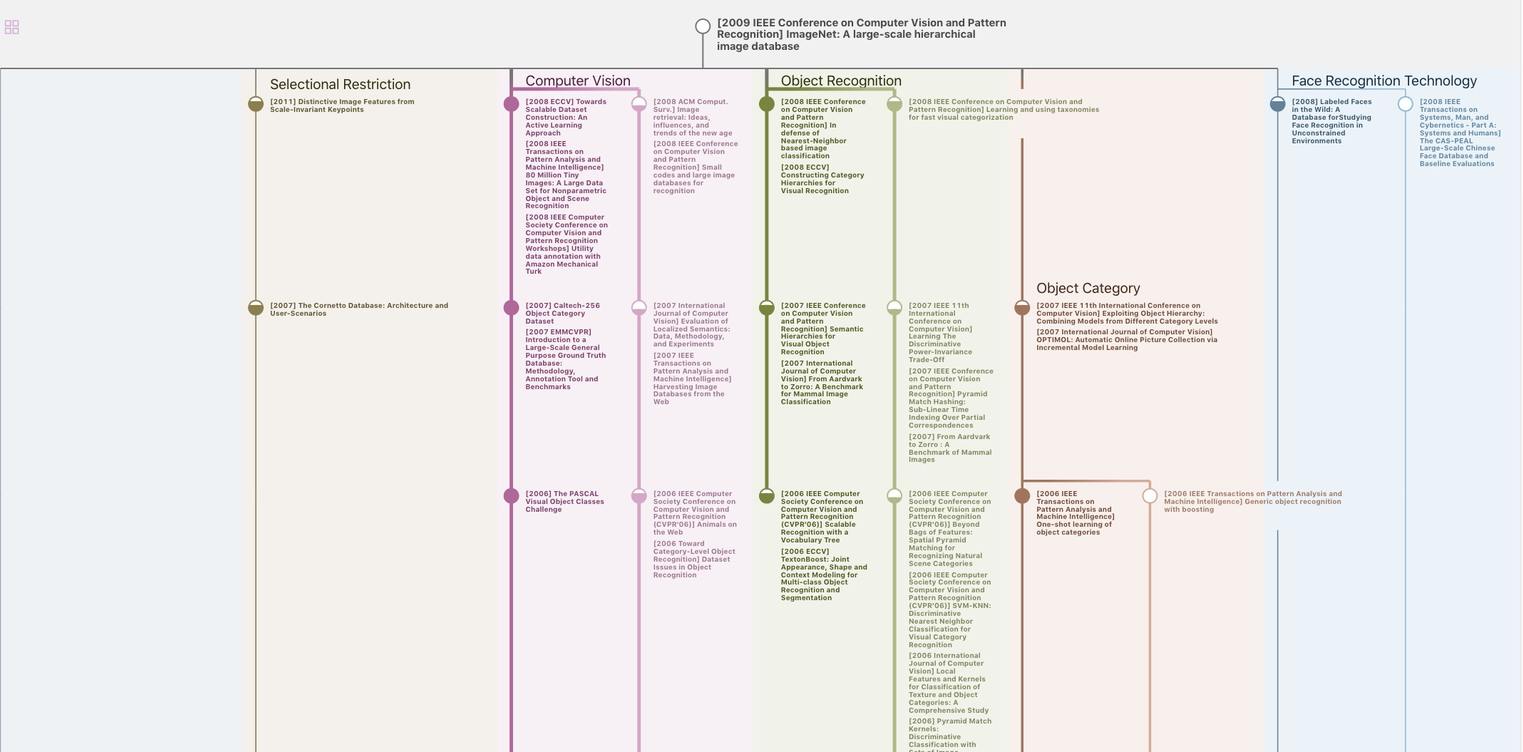
生成溯源树,研究论文发展脉络
Chat Paper
正在生成论文摘要