Enhanced Machine-Learning Flow for Microwave-Sensing Systems to Detect Contaminants in Food
2023 IEEE Conference on AgriFood Electronics (CAFE)(2023)
摘要
The presence of foreign bodies in packaged food is a serious concern for both final consumers (allergies, injuries, choking) and food manufacturers (reputation and economic losses). In particular, low-density plastics, glass and wood splinters are hard to detect even by the most advanced X-ray imagers. One solution is Machine-Learning-based Microwave Sensing (MLMWS): a non-invasive, contactless, and real-time method which uses a machine-learning (ML) classifier to analyze the scattered microwaves from the irradiated target object. In this paper, we want to extend our previous work about contaminant detection in cocoa-hazelnut spread jars by proposing an enhanced ML flow to increase the accuracy of the ML classifier. For the first time in this case study, we use a multi-class classifier, we train it with scattering parameters measured at multiple microwave frequencies, with a new pre-processing scaler, data augmentation, quantization-aware training and a pruning schedule. The results show a contaminant detection multi-class accuracy of 94.167% with a latency of
$26 \mu s$
when targeting an AMD/Xilinx Kria K26 FPGA. Finally, we released our datasets publicly to OpenML.
1
1
Fermilab report number: FERMILAB-PUB-23-313-PPD
更多查看译文
关键词
foreign body detection in food,machine learning,microwave sensing,neural networks,fpga acceleration
AI 理解论文
溯源树
样例
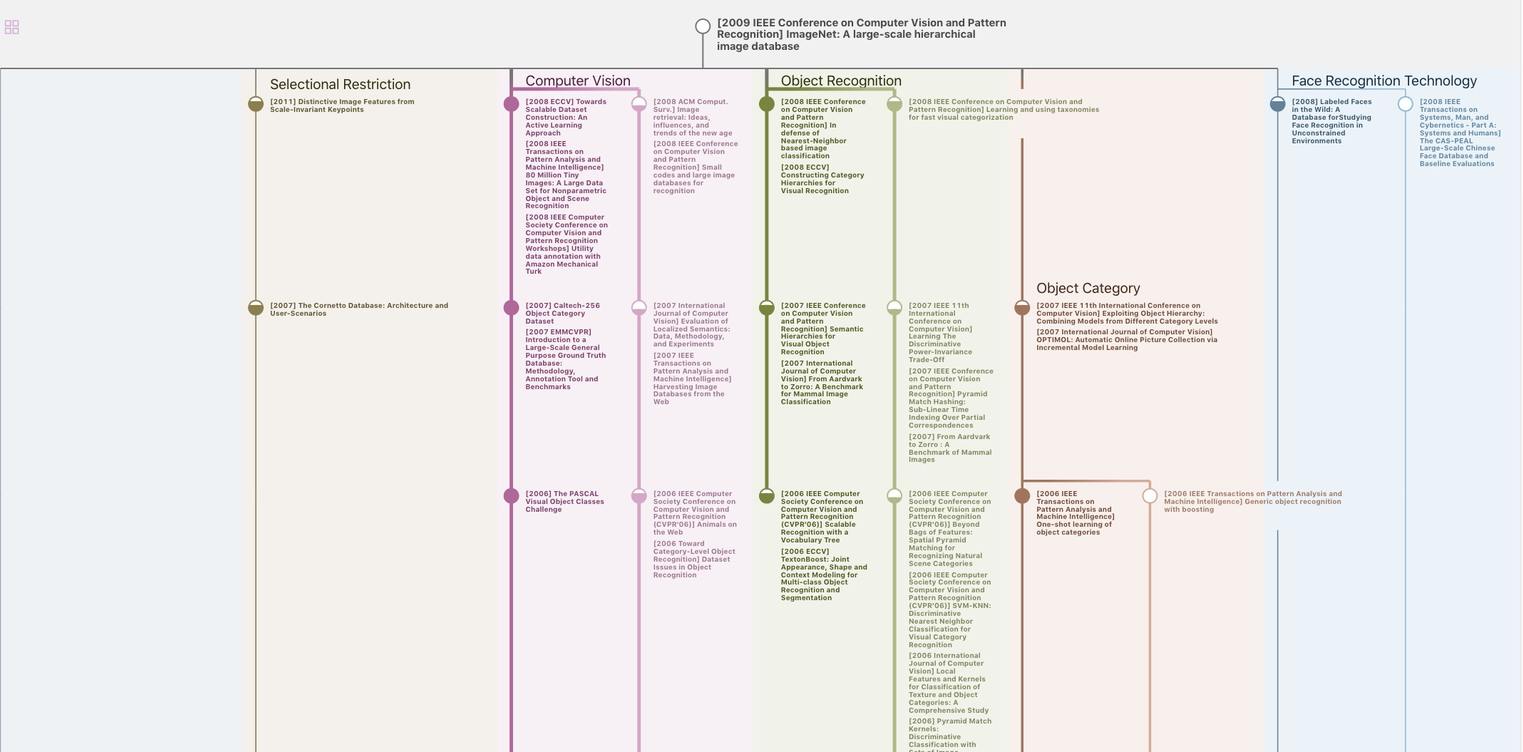
生成溯源树,研究论文发展脉络
Chat Paper
正在生成论文摘要