Deep Learning Enables Large Depth-of-Field Images for Sub-Diffraction-Limit Scanning Superlens Microscopy
CoRR(2023)
摘要
Scanning electron microscopy (SEM) is indispensable in diverse applications ranging from microelectronics to food processing because it provides large depth-of-field images with a resolution beyond the optical diffraction limit. However, the technology requires coating conductive films on insulator samples and a vacuum environment. We use deep learning to obtain the mapping relationship between optical super-resolution (OSR) images and SEM domain images, which enables the transformation of OSR images into SEM-like large depth-of-field images. Our custom-built scanning superlens microscopy (SSUM) system, which requires neither coating samples by conductive films nor a vacuum environment, is used to acquire the OSR images with features down to ~80 nm. The peak signal-to-noise ratio (PSNR) and structural similarity index measure values indicate that the deep learning method performs excellently in image-to-image translation, with a PSNR improvement of about 0.74 dB over the optical super-resolution images. The proposed method provides a high level of detail in the reconstructed results, indicating that it has broad applicability to chip-level defect detection, biological sample analysis, forensics, and various other fields.
更多查看译文
关键词
deep learning,depth-of-field,sub-diffraction-limit
AI 理解论文
溯源树
样例
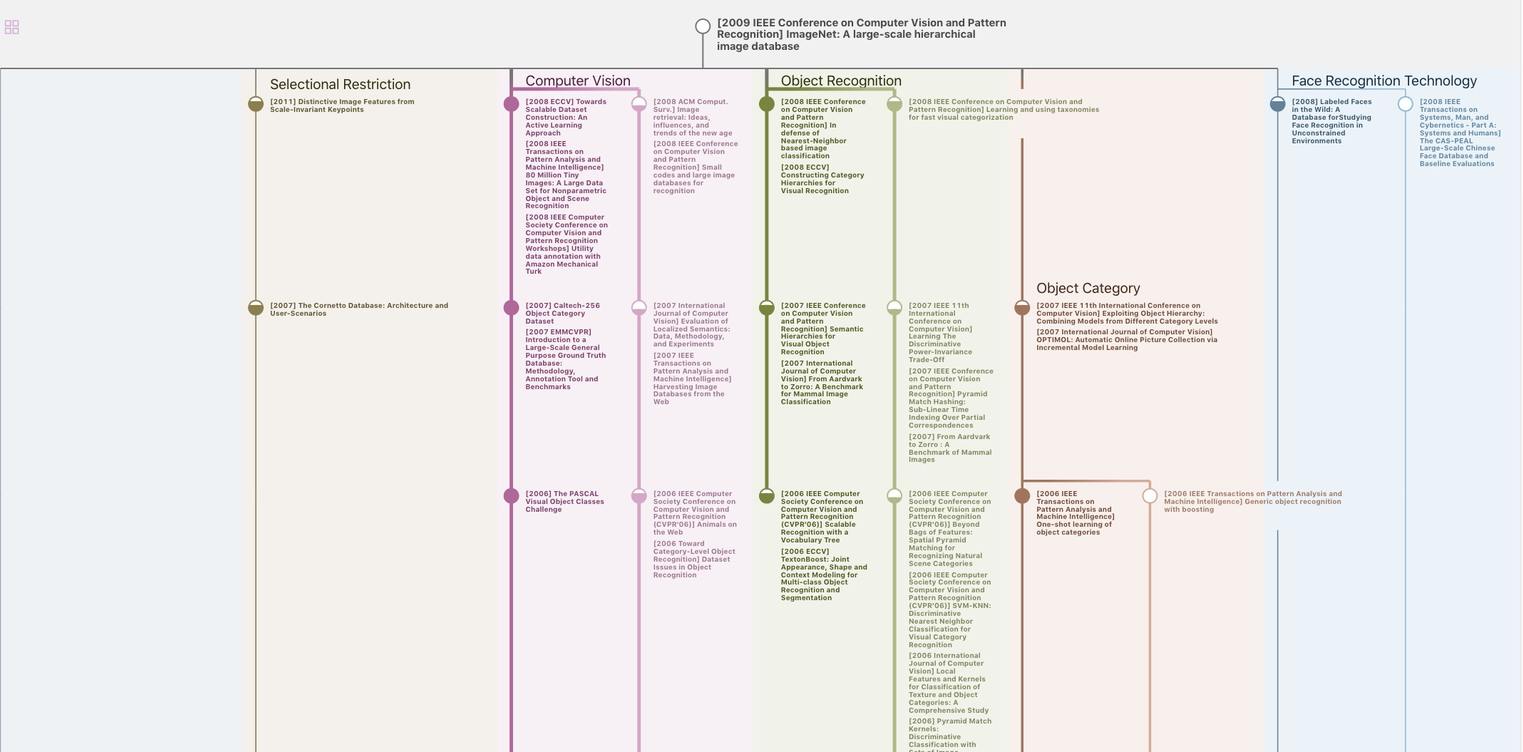
生成溯源树,研究论文发展脉络
Chat Paper
正在生成论文摘要