Raw Mill Engine Failure Detection from Transformer Gated Convolutional Unit Networks
2023 IEEE International Conference on Data and Software Engineering (ICoDSE)(2023)
摘要
From the era of Industry 4.0, Internet-of-Things (IoT) technology has developed an industrial maintenance strategy, namely Predictive Maintenance (PdM), to boost massive production processes. One task in PdM is to estimate the engine failure time to help the decision-making on maintenance strategies, called diagnostic task. Besides that, diagnostic tasks play a dominant role as they can ensure uninterrupted production steps in almost all industry fields, including a sand-cement factory. In this work, we focus on raw mill as the first major engine in sand-cement production. Further, we attempt to estimate the failure time of the raw mill engine as early as possible by applying Transformer-Gated Convolutional Unit Networks (T-GCUN). In brief, the Gated Convolutional Unit (GCU) captures the local features of the multi-sensor data. The transformer estimates the failure time of raw mill engine by learning the short and long-term dependencies and local features from GCU. In the experiments, we studied multi-sensor data from the raw mill engine in one of the sand-cement factories in Indonesia around 2015, as most failures occurred. Within various time cycles, T-GCUN can predict the failure time of raw engine earlier than the ground truth.
更多查看译文
关键词
Deep Learning,Transformer Model,Predictive maintenance,Engine Time Failure,Raw Mill Machine
AI 理解论文
溯源树
样例
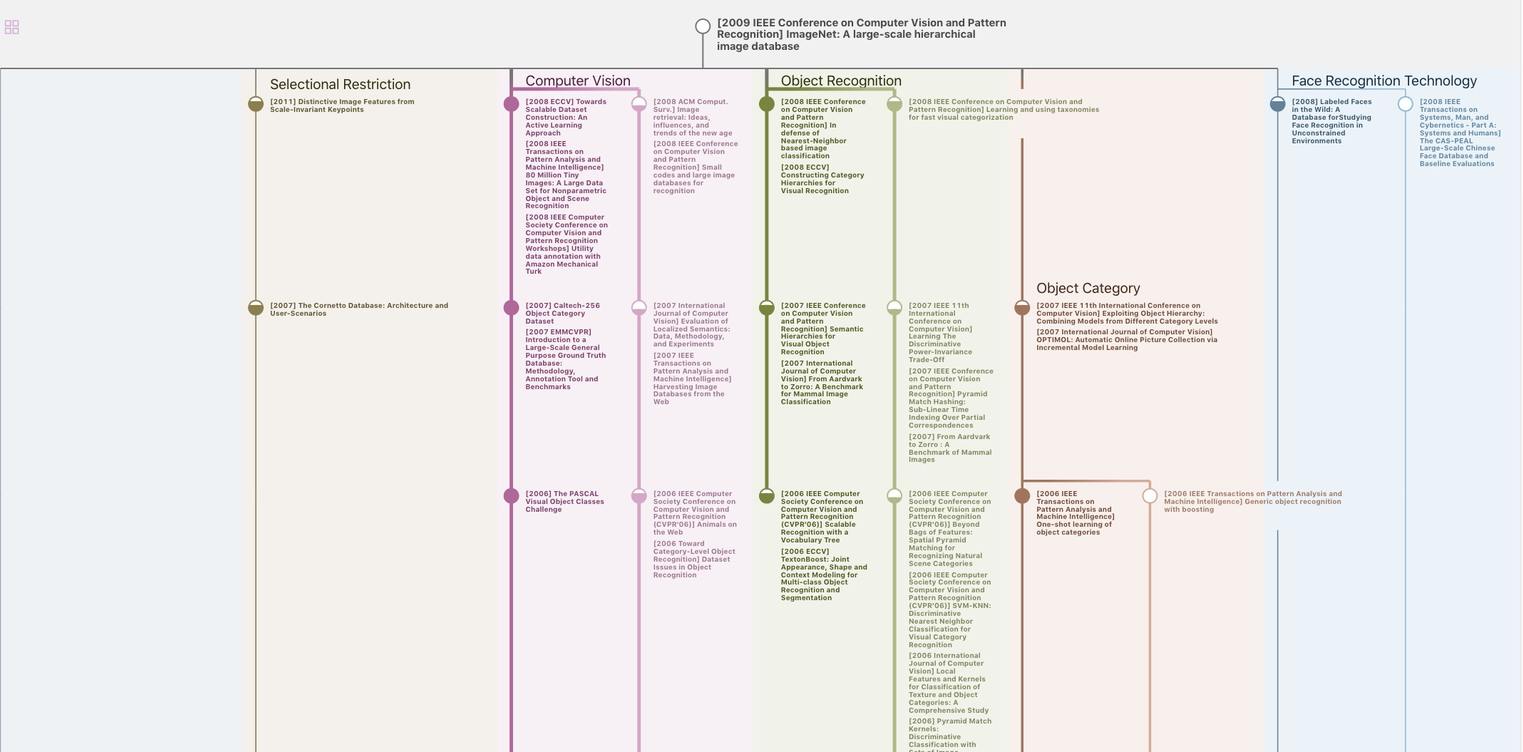
生成溯源树,研究论文发展脉络
Chat Paper
正在生成论文摘要