Personalized recommendation via inductive spatiotemporal graph neural network
PATTERN RECOGNITION(2024)
摘要
Graph neural network-based collaborative filtering methods have achieved excellent performance in recommender systems. However, previous works have primarily focused on representation using the entire graph or a single sampled subgraph, which fails to address the issue of noise introduced by prolonged ineffective interactions. Moreover, these approaches lack the capacity to model temporal information in an inductive manner, thereby limiting their ability to fully capture the evolving dynamic interests of users over time in a real world scenario. To address the aforementioned challenges, we propose a novel personalized inductive spatiotemporal graph neural network-based framework PistGNN. PistGNN extracts multiple temporal-aware subgraphs from user-item pairs to avoid long-time gap meaningless interactions, which are then fed into a spatiotemporal graph neural network module. It is worth noting that PistGNN trains the model only by relying on subgraphs extracted from bipartite graphs, without depending on global information, and is therefore capable of predicting for new users or items. Finally, we propose an attention-based meta-learning method to personalize the aggregation of subgraphs' embeddings. Extensive experiments conducted on four real-world datasets demonstrate the superiority of PistGNN over both inductive and transductive baseline methods.
更多查看译文
关键词
Recommendation system,Inductive learning,Spatiotemporal graph neural network
AI 理解论文
溯源树
样例
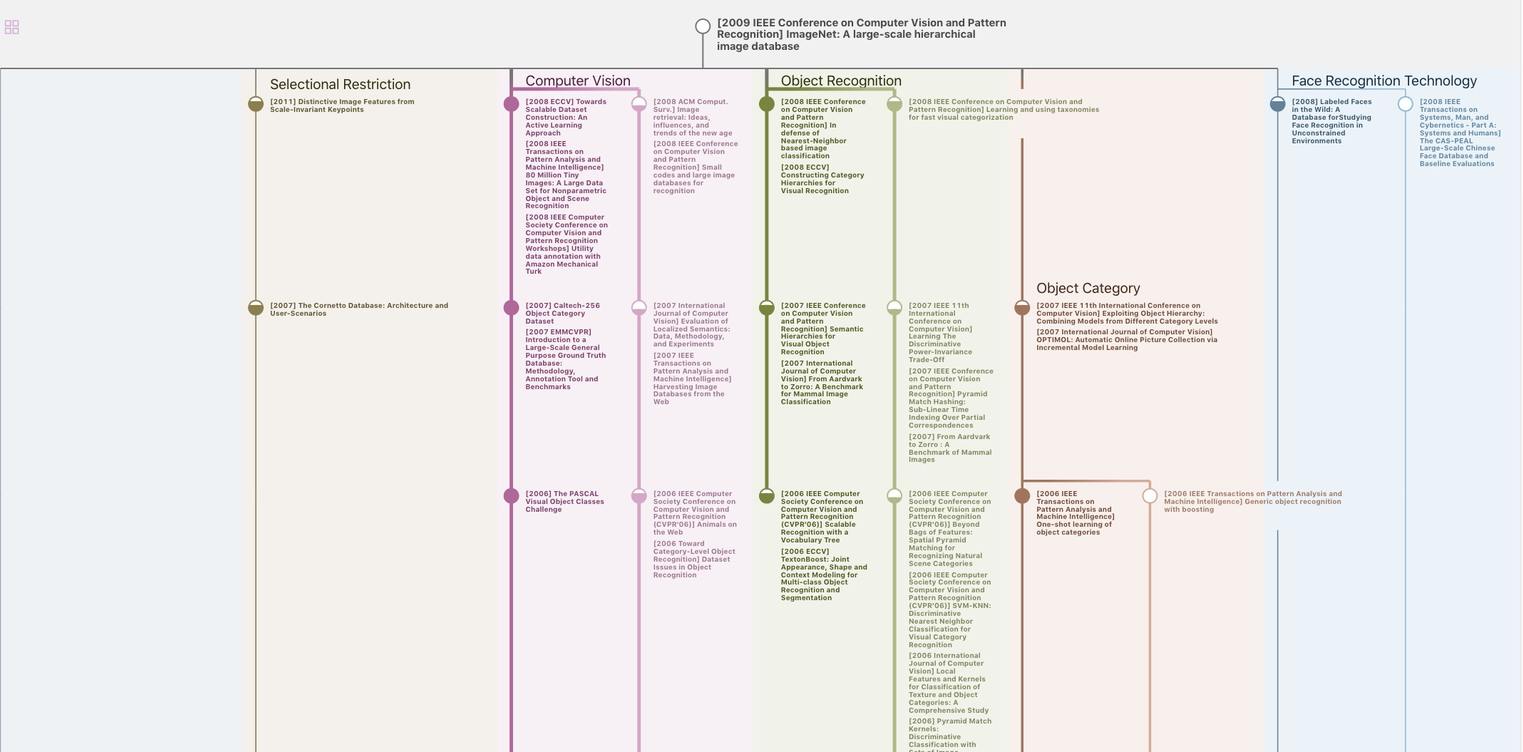
生成溯源树,研究论文发展脉络
Chat Paper
正在生成论文摘要