Knowledge-informed Variational Bayesian Gaussian mixture regression model for predicting mixed oil length
Energy(2023)
摘要
Precise prediction of mixed oil length is a critical task in the multi-product pipeline. Current predictive models may not achieve desirable performance as they neglected the multimode characteristics of the data, which has been alleviated by using the finite mixture model (FMM) to identify the different modes and develop localized predictive models while it faces the challenge of assigning a suitable number of components. Variational Bayesian Gaussian mixture regression model (VBGMR) may remedy these deficiencies but the linear assumption between variables can result in unsatisfactory performance, and it may also not handle the model selection well since all input variables are forced to participate in the mode identification process, even if some are not important. To address such issues, this article proposes a new modeling approach named as the Knowledgeinformed Variational Bayesian Gaussian mixture regression model (KIVBGMR) by incorporating the conventional VBGMR with the guidance of the domain knowledge. Subsequently, the learning procedure for the KIVBGMR based on the Variational Inference method is developed. The performance of the KIVBGMR modeling method is evaluated based on two case studies including a numerical example and a real-life industrial dataset, which demonstrates the performance superiority of the proposed method.
更多查看译文
关键词
Multi-product pipeline,Mixed oil length,Knowledge-data,Multi -mode
AI 理解论文
溯源树
样例
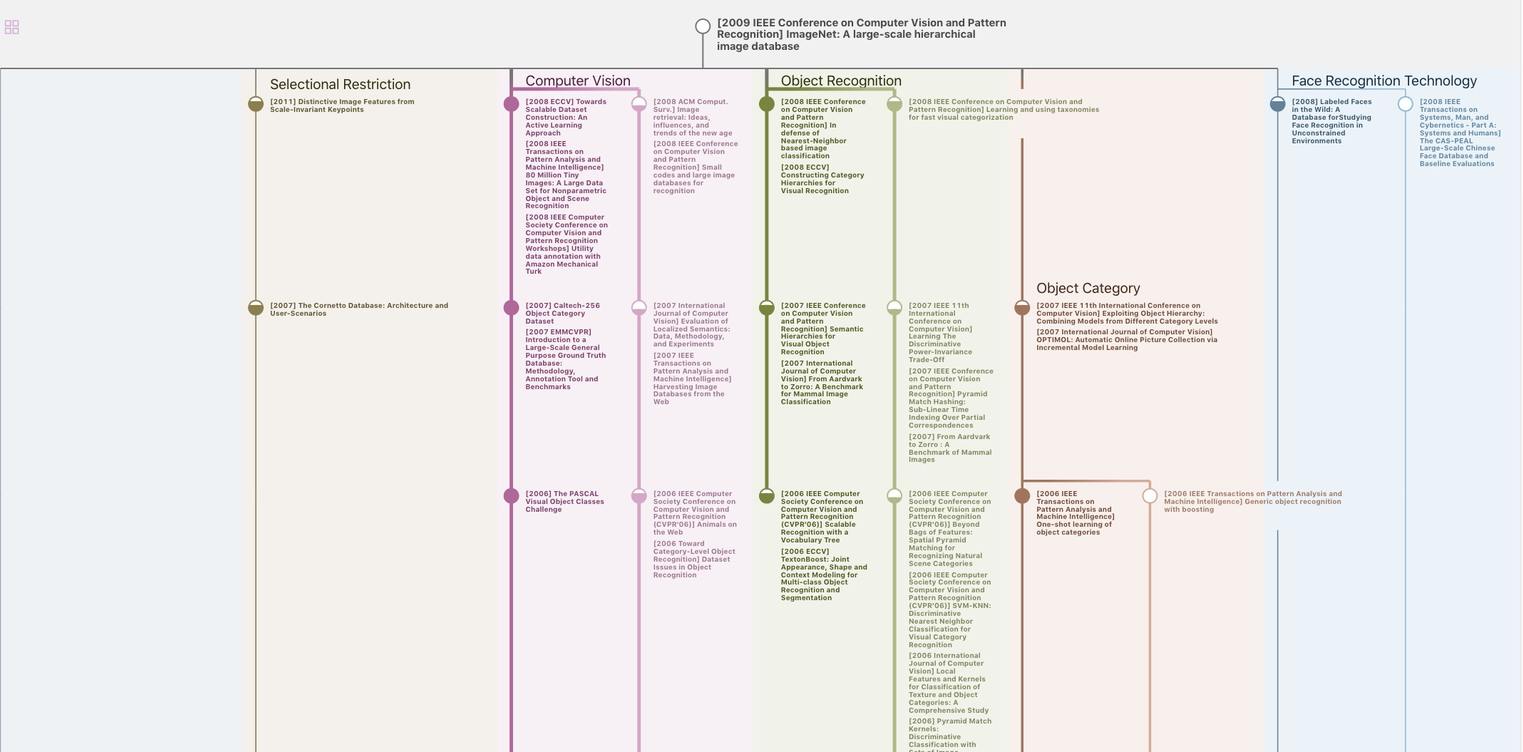
生成溯源树,研究论文发展脉络
Chat Paper
正在生成论文摘要