Pre-test prediction of multiple druggable mutations based on H&E image artificial intelligence (AI) analysis may enable more efficient clinical workflow for treatment decisions in non-small cell lung cancer (NSCLC)
ANNALS OF ONCOLOGY(2023)
摘要
AI may predict driver mutations from H&E slides, and this prediction may be useful as a screening tool to guide the efficient use of molecular testing. Here, we investigate the prediction performance for mutations in six driver oncogenes and explore the benefits to clinical workflow in NSCLC treatment decisions. NSCLC samples from Neogenomics (n=2,161) and TCGA (n=933) were used for model development. Four features were used: two convolutional neural networks trained for cell and tissue, a self-supervised vision transformer, and an AI-based pathology profiling analyzer for semantic contents. A set of classifiers was trained and ensembled for improved robustness. Performance of the model was validated in an independent dataset (n=792), then we simulated real-world impact based on the prevalence of driver mutations from AACR GENIE v13.1 NSCLC (n=19,722). Table 2330P shows the dataset and performance of the models. Given 2.8% prevalence of MET exon skipping mutations (MET-ex), 8.5% positive predictive value (PPV) means that a test-positive patient has a 3x higher chance of being true-MET-ex positive compared to the overall patient population. Moreover, given 99.2% specificity and 49.4% prevalence, PPV for selecting patients without mutations (All-WT) is 95.2%, enabling avoidance of unnecessary tests with potentially acceptable error (<5%).Table: 2330PInternal setExternal setn (%)AUCn (%)AUCSensitivitySpecificityEGFR-mt384 (12.4)0.841224 (28.3)0.72375.5%52.6%KRAS-mt516 (16.7)0.728130 (16.4)0.72186.2%22.5%ALK-tr310 (10.0)0.79546 (5.8)0.73839.1%81.8%ROS1-tr295 (9.5)0.79362 (7.8)0.60914.5%70.6%RET-tr307 (9.9)0.76311 (1.4)0.68318.2%96.4%MET-ex240 (7.8)0.78912 (1.5)0.84983.3%74.4%All-WT1045 (33.8)N/A277 (35.0)N/A15.9%99.2%AUC, area-under-the-curve; ex, exon skipping; mt, mutation; tr, translocation. Open table in a new tab AUC, area-under-the-curve; ex, exon skipping; mt, mutation; tr, translocation. A novel clinical workflow, using AI analysis of H&E to predict genomic profiles and identify which patients would benefit from confirmatory molecular testing, could enable better and more efficient treatment decisions.
更多查看译文
AI 理解论文
溯源树
样例
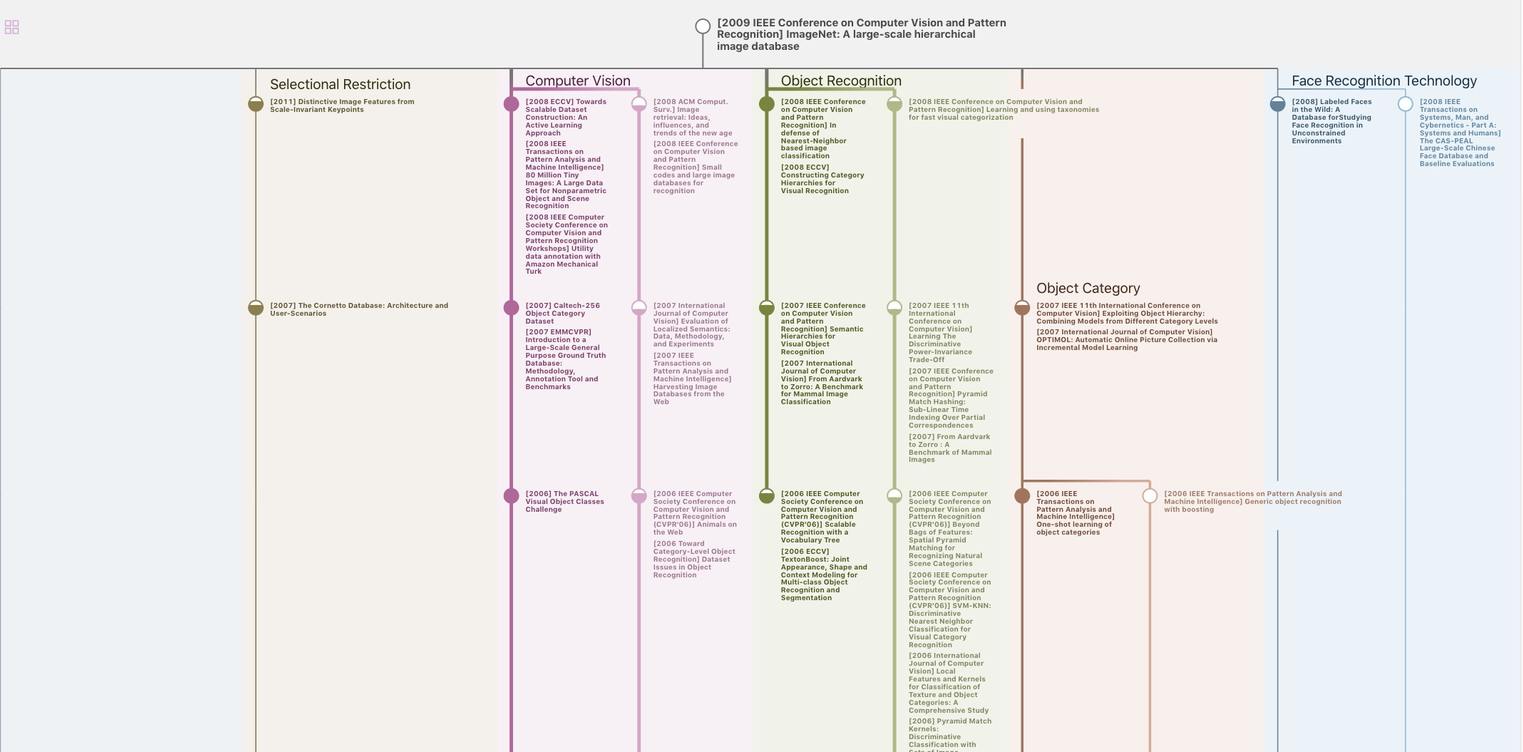
生成溯源树,研究论文发展脉络
Chat Paper
正在生成论文摘要