Analyzing Disparity and Temporal Progression of Internet Quality through Crowdsourced Measurements with Bias-Correction
CoRR(2023)
摘要
Crowdsourced speedtest measurements are an important tool for studying internet performance from the end user perspective. Nevertheless, despite the accuracy of individual measurements, simplistic aggregation of these data points is problematic due to their intrinsic sampling bias. In this work, we utilize a dataset of nearly 1 million individual Ookla Speedtest measurements, correlate each datapoint with 2019 Census demographic data, and develop new methods to present a novel analysis to quantify regional sampling bias and the relationship of internet performance to demographic profile. We find that the crowdsourced Ookla Speedtest data points contain significant sampling bias across different census block groups based on a statistical test of homogeneity. We introduce two methods to correct the regional bias by the population of each census block group. Whereas the sampling bias leads to a small discrepancy in the overall cumulative distribution function of internet speed in a city between estimation from original samples and bias-corrected estimation, the discrepancy is much smaller compared to the size of the sampling heterogeneity across regions. Further, we show that the sampling bias is strongly associated with a few demographic variables, such as income, education level, age, and ethnic distribution. Through regression analysis, we find that regions with higher income, younger populations, and lower representation of Hispanic residents tend to measure faster internet speeds along with substantial collinearity amongst socioeconomic attributes and ethnic composition. Finally, we find that average internet speed increases over time based on both linear and nonlinear analysis from state space models, though the regional sampling bias may result in a small overestimation of the temporal increase of internet speed.
更多查看译文
关键词
internet quality,crowdsourced measurements,bias-correction bias-correction,disparity
AI 理解论文
溯源树
样例
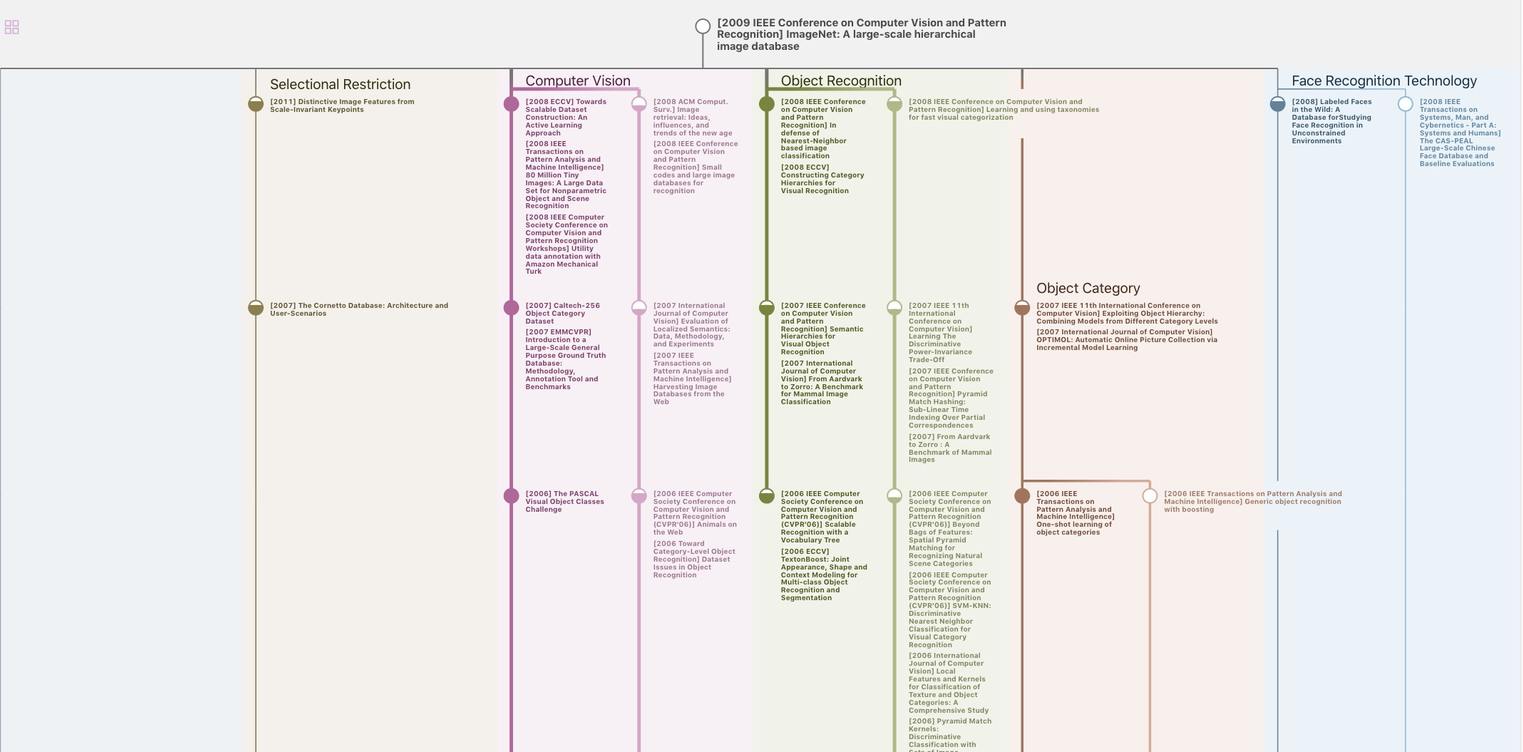
生成溯源树,研究论文发展脉络
Chat Paper
正在生成论文摘要