MuLMS: A Multi-Layer Annotated Text Corpus for Information Extraction in the Materials Science Domain
Proceedings of the Second Workshop on Information Extraction from Scientific Publications(2023)
摘要
Keeping track of all relevant recent publications and experimental results for a research area is a challenging task. Prior work has demonstrated the efficacy of information extraction models in various scientific areas. Recently, several datasets have been released for the yet understudied materials science domain. However, these datasets focus on sub-problems such as parsing synthesis procedures or on sub-domains, e.g., solid oxide fuel cells. In this resource paper, we present MuLMS, a new dataset of 50 open-access articles, spanning seven sub-domains of materials science. The corpus has been annotated by domain experts with several layers ranging from named entities over relations to frame structures. We present competitive neural models for all tasks and demonstrate that multi-task training with existing related resources leads to benefits.
更多查看译文
关键词
information extraction,corpus,materials,text,multi-layer
AI 理解论文
溯源树
样例
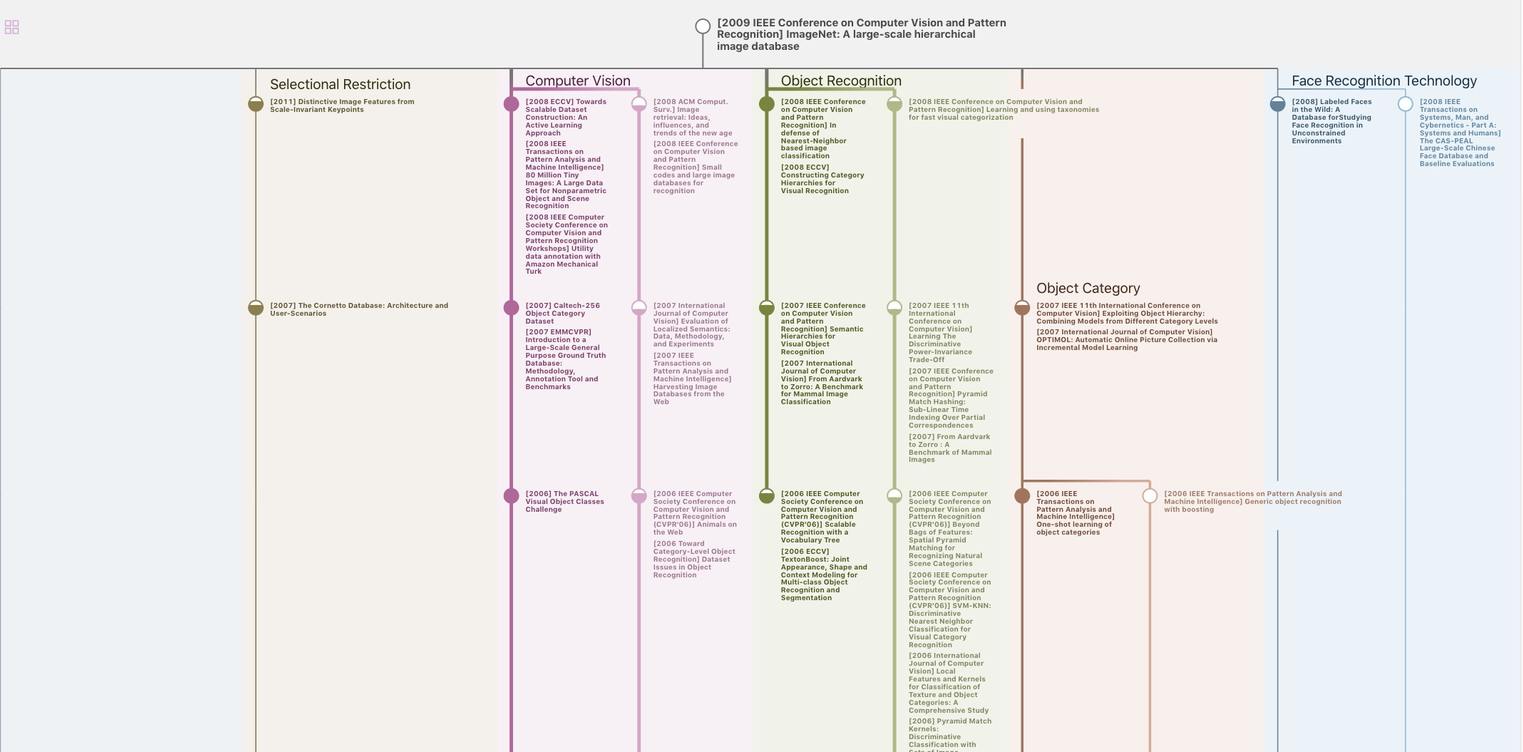
生成溯源树,研究论文发展脉络
Chat Paper
正在生成论文摘要