Field-level Simulation-Based Inference with Galaxy Catalogs: the Impact of Systematic Effects
JOURNAL OF COSMOLOGY AND ASTROPARTICLE PHYSICS(2025)
Flatiron Inst | Univ Sao Paulo | INAF | Harvard Smithsonian Ctr Astrophys | Univ Portsmouth | Ludwig Maximilians Univ Munchen | Univ Bologna | MIT
Abstract
It has been recently shown that a powerful way to constrain cosmological parameters from galaxy redshift surveys is to train graph neural networks to perform field-level likelihood-free inference without imposing cuts on scale. In particular, de Santi et al. (2023) developed models that could accurately infer the value of $\Omega_{\rm m}$ from catalogs that only contain the positions and radial velocities of galaxies that are robust to uncertainties in astrophysics and subgrid models. However, observations are affected by many effects, including 1) masking, 2) uncertainties in peculiar velocities and radial distances, and 3) different galaxy selections. Moreover, observations only allow us to measure redshift, intertwining galaxies' radial positions and velocities. In this paper we train and test our models on galaxy catalogs, created from thousands of state-of-the-art hydrodynamic simulations run with different codes from the CAMELS project, that incorporate these observational effects. We find that, although the presence of these effects degrades the precision and accuracy of the models, and increases the fraction of catalogs where the model breaks down, the fraction of galaxy catalogs where the model performs well is over 90 %, demonstrating the potential of these models to constrain cosmological parameters even when applied to real data.
MoreTranslated text
Key words
cosmological parameters from LSS,Machine learning,hydrodynamical simulations
PDF
View via Publisher
AI Read Science
AI Summary
AI Summary is the key point extracted automatically understanding the full text of the paper, including the background, methods, results, conclusions, icons and other key content, so that you can get the outline of the paper at a glance.
Example
Background
Key content
Introduction
Methods
Results
Related work
Fund
Key content
- Pretraining has recently greatly promoted the development of natural language processing (NLP)
- We show that M6 outperforms the baselines in multimodal downstream tasks, and the large M6 with 10 parameters can reach a better performance
- We propose a method called M6 that is able to process information of multiple modalities and perform both single-modal and cross-modal understanding and generation
- The model is scaled to large model with 10 billion parameters with sophisticated deployment, and the 10 -parameter M6-large is the largest pretrained model in Chinese
- Experimental results show that our proposed M6 outperforms the baseline in a number of downstream tasks concerning both single modality and multiple modalities We will continue the pretraining of extremely large models by increasing data to explore the limit of its performance
Try using models to generate summary,it takes about 60s
Must-Reading Tree
Example
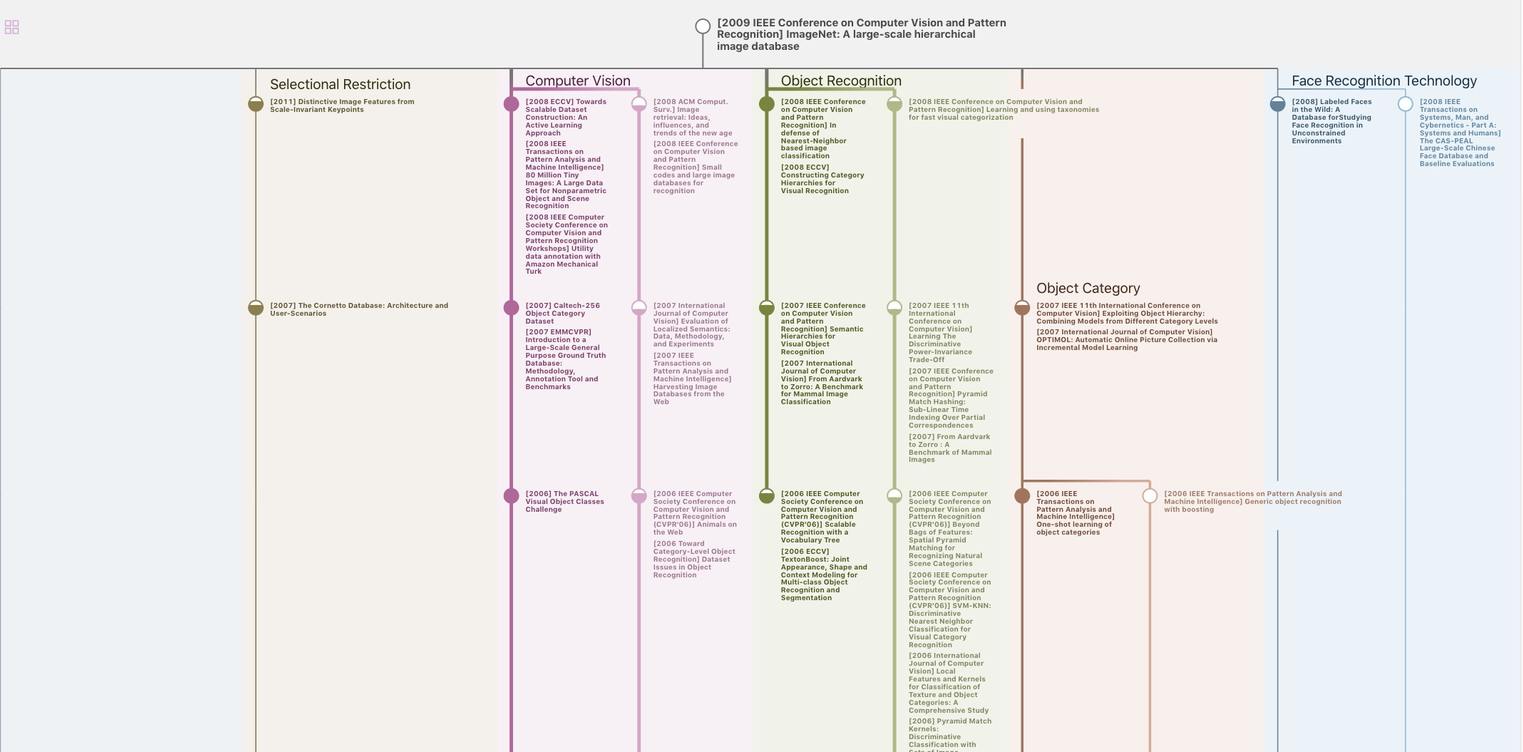
Generate MRT to find the research sequence of this paper
Related Papers
Data Disclaimer
The page data are from open Internet sources, cooperative publishers and automatic analysis results through AI technology. We do not make any commitments and guarantees for the validity, accuracy, correctness, reliability, completeness and timeliness of the page data. If you have any questions, please contact us by email: report@aminer.cn
Chat Paper
去 AI 文献库 对话