Improving Cross-lingual Transfer through Subtree-aware Word Reordering.
CoRR(2023)
摘要
Despite the impressive growth of the abilities of multilingual language models, such as XLM-R and mT5, it has been shown that they still face difficulties when tackling typologically-distant languages, particularly in the low-resource setting. One obstacle for effective cross-lingual transfer is variability in word-order patterns. It can be potentially mitigated via source- or target-side word reordering, and numerous approaches to reordering have been proposed. However, they rely on language-specific rules, work on the level of POS tags, or only target the main clause, leaving subordinate clauses intact. To address these limitations, we present a new powerful reordering method, defined in terms of Universal Dependencies, that is able to learn fine-grained word-order patterns conditioned on the syntactic context from a small amount of annotated data and can be applied at all levels of the syntactic tree. We conduct experiments on a diverse set of tasks and show that our method consistently outperforms strong baselines over different language pairs and model architectures. This performance advantage holds true in both zero-shot and few-shot scenarios.
更多查看译文
关键词
transfer,cross-lingual,subtree-aware
AI 理解论文
溯源树
样例
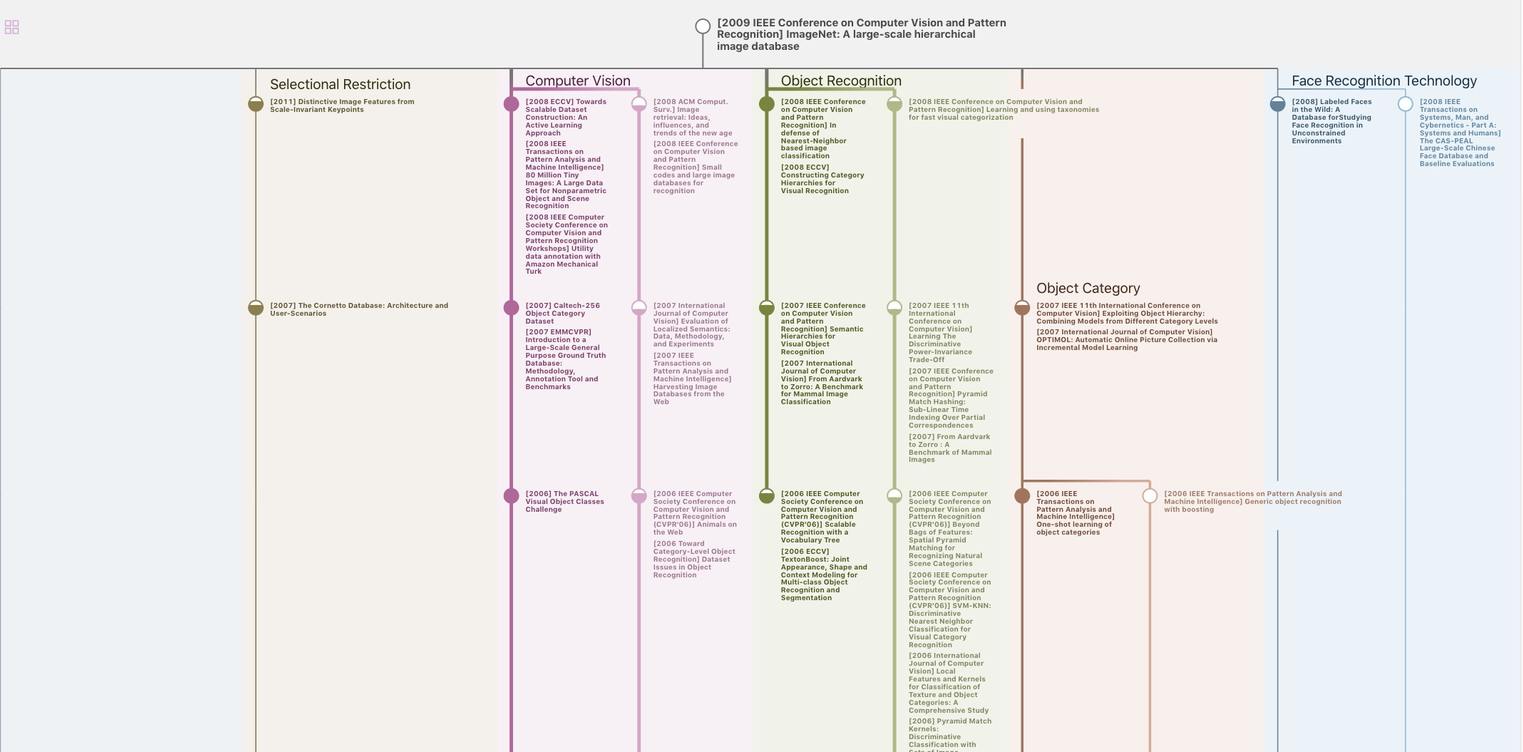
生成溯源树,研究论文发展脉络
Chat Paper
正在生成论文摘要