A Graph Deep Learning-Based Framework for Drug-Disease Association Identification with Chemical Structure Similarities
JOURNAL OF COMPUTATIONAL BIOPHYSICS AND CHEMISTRY(2023)
摘要
Traditional drug development requires a lot of time and effort, while computational drug repositioning enables to discover the underlying mechanisms of drugs, thereby reducing the cost of drug discovery and development. However, existing computational models utilize only low-order biological information at the level of individual drugs, diseases and their associations, making it difficult to dig deeper and fuse higher-order structural information. In this paper, we develop a novel prediction model, namely DRGDL, based on graph deep learning to infer potential drug-disease associations (DDAs) by coming chemical structural similarity information, which can more comprehensively make use of the biological features of drugs and diseases. First, the lower-order and higher-order representations of drugs and diseases are captured by two different graph deep learning strategies. Then, suitable negative samples are select by use of k-means algorithm. At last, the Random Forest classifier is applied to complete the prediction task of DDAs by integrating two representations of drugs and diseases. Experiment results indicate that DRGDL achieves excellent performance under ten-fold cross-validation on two benchmark datasets.
更多查看译文
关键词
Graph deep learning,computational drug repositioning,drug-disease association prediction,DDAs
AI 理解论文
溯源树
样例
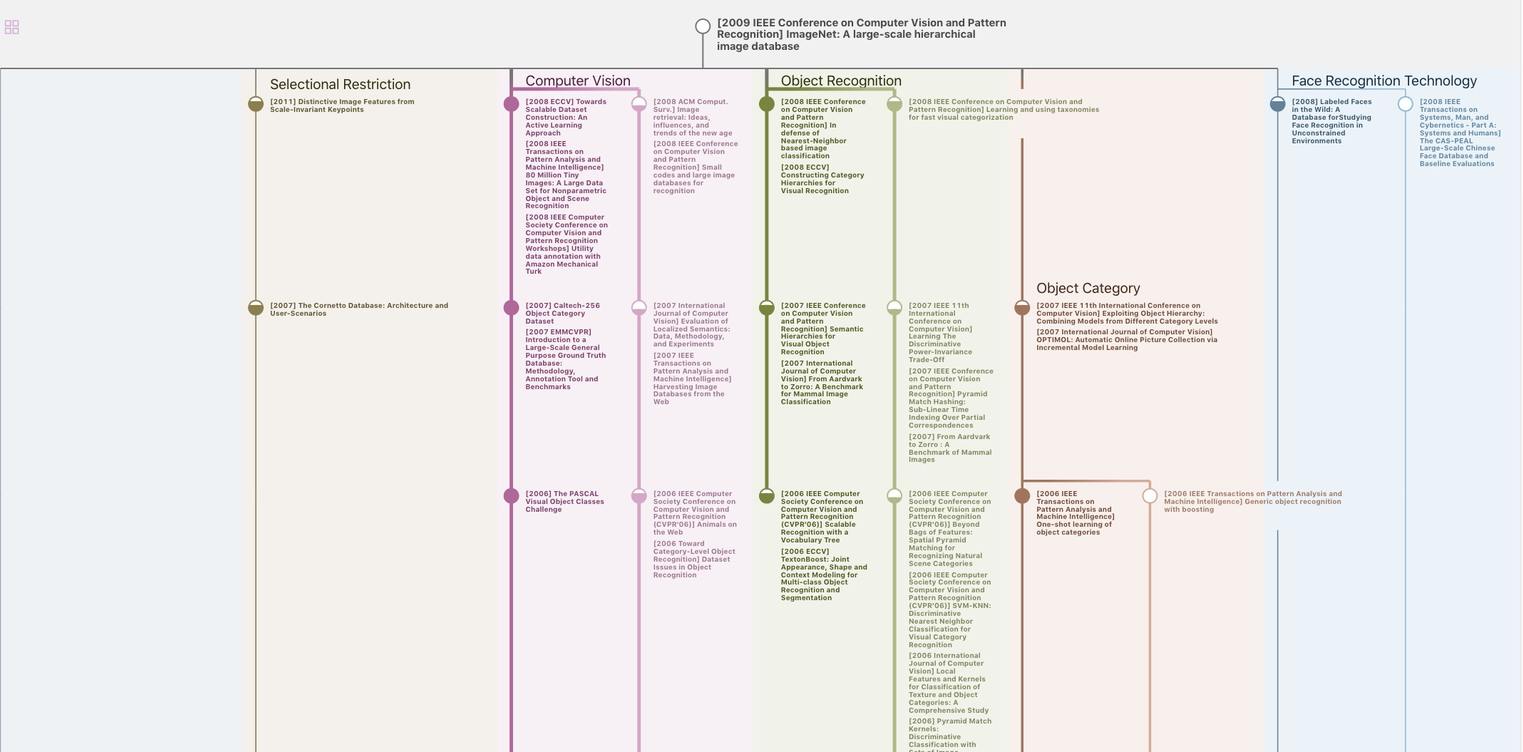
生成溯源树,研究论文发展脉络
Chat Paper
正在生成论文摘要