COMET: Cross-space Optimization-based Mutual Learning Network for Super-Resolution of CEST-MRI.
IEEE JOURNAL OF BIOMEDICAL AND HEALTH INFORMATICS(2024)
摘要
Chemical Exchange Saturation Transfer Magn-etic Resonance Imaging (CEST-MRI) is a promising approach for detecting tissue metabolic changes. However, due to the constraints of scan time and contrast-noise-ratio, CEST-MRI always exhibits low spatial resolution, hindering the clinical applications especially for detection of small lesions. Many super-resolution (SR) methods have shown good performance in medical images. However, when applied to CEST-MRI, these methods have two shortcomings that may limit their performance. Firstly, CEST-MRI has an additional frequency dimension, but the information along this dimension is not fully utilized. The second is that these SR methods mainly focus on improving the quality of the CEST-weighted images, while the accuracy of the quantitative maps is the most concerned aspect for CEST-MRI. To address these shortcomings, we propose a Cross-space Optimization-based Mutual learning nETwork (COMET) for SR of CEST-MRI. COMET incorporates novel spatio-frequency extraction modules and a mutual learning module to leverage and combine information from both spatial and frequency spaces, thereby enhancing the SR performance. Furthermore, we propose a novel CEST-based normalization loss to address the normalization-induced distribution problem and preserve the sharpness of quantitative maps, enabling more accurate CEST-MRI quantification. COMET is evaluated on an ischemia rat brain dataset and a human brain dataset. The results demonstrate COMET achieves 8-fold SR, providing accurate quantitative maps. Moreover, COMET outperforms all other state-of-the-art SR methods. Additionally, COMET exhibits its potential in prospective study.
更多查看译文
关键词
Chemical exchange saturation transfer,deep-learning,optimization-based network,super-resolution
AI 理解论文
溯源树
样例
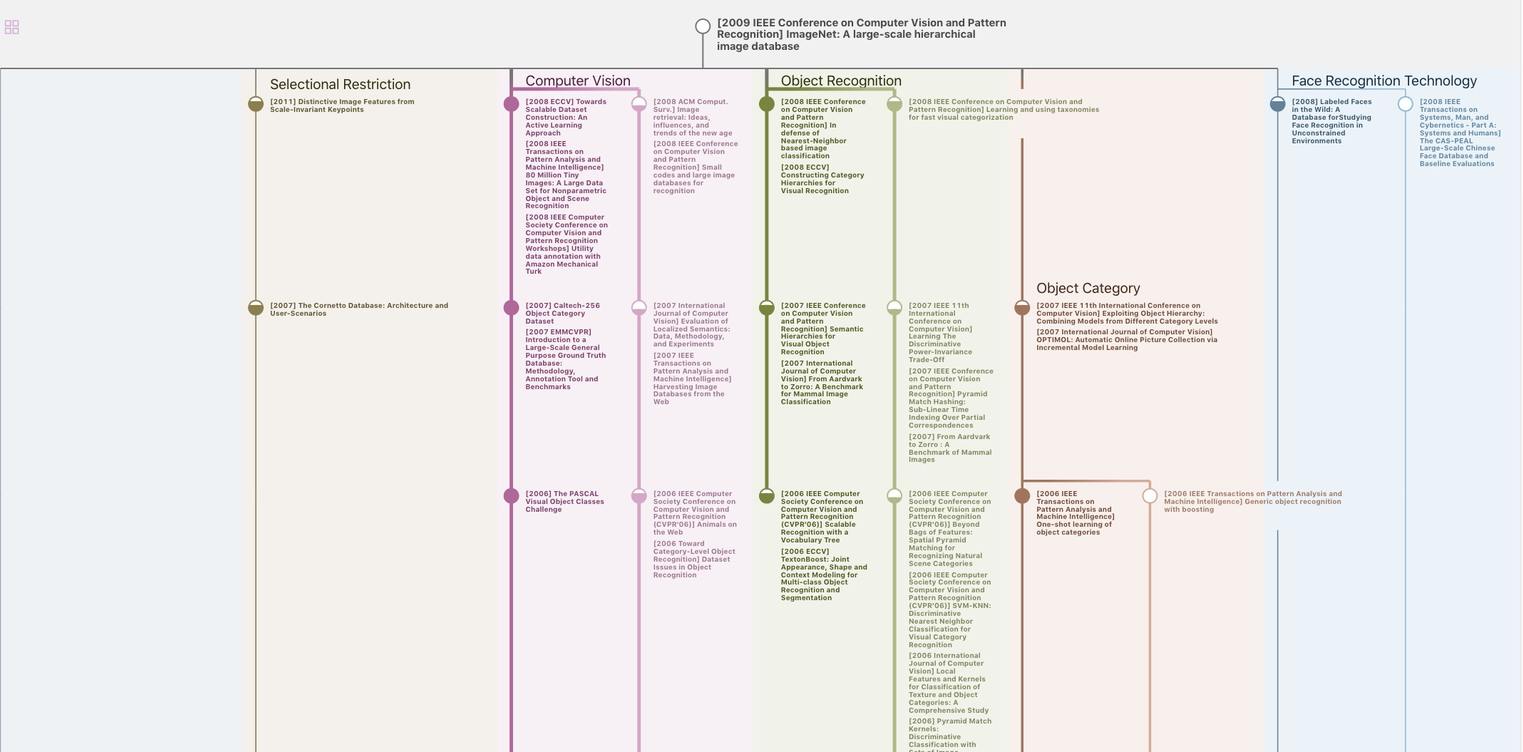
生成溯源树,研究论文发展脉络
Chat Paper
正在生成论文摘要