Distributed Indexing Schemes for k-Dominant Skyline Analytics on Uncertain Edge-IoT Data
CoRR(2023)
摘要
Skyline queries typically search a Pareto-optimal set from a given data set to solve the corresponding multiobjective optimization problem. As the number of criteria increases, the skyline presumes excessive data items, which yield a meaningless result. To address this curse of dimensionality, we proposed a k-dominant skyline in which the number of skyline members was reduced by relaxing the restriction on the number of dimensions, considering the uncertainty of data. Specifically, each data item was associated with a probability of appearance, which represented the probability of becoming a member of the k-dominant skyline. As data items appear continuously in data streams, the corresponding k-dominant skyline may vary with time. Therefore, an effective and rapid mechanism of updating the k-dominant skyline becomes crucial. Herein, we proposed two time-efficient schemes, Middle Indexing (MI) and All Indexing (AI), for k-dominant skyline in distributed edge-computing environments, where irrelevant data items can be effectively excluded from the compute to reduce the processing duration. Furthermore, the proposed schemes were validated with extensive experimental simulations. The experimental results demonstrated that the proposed MI and AI schemes reduced the computation time by approximately 13% and 56%, respectively, compared with the existing method.
更多查看译文
关键词
Pareto-optimal,$k$ -dominant skyline,skyline,uncertain data,data streams
AI 理解论文
溯源树
样例
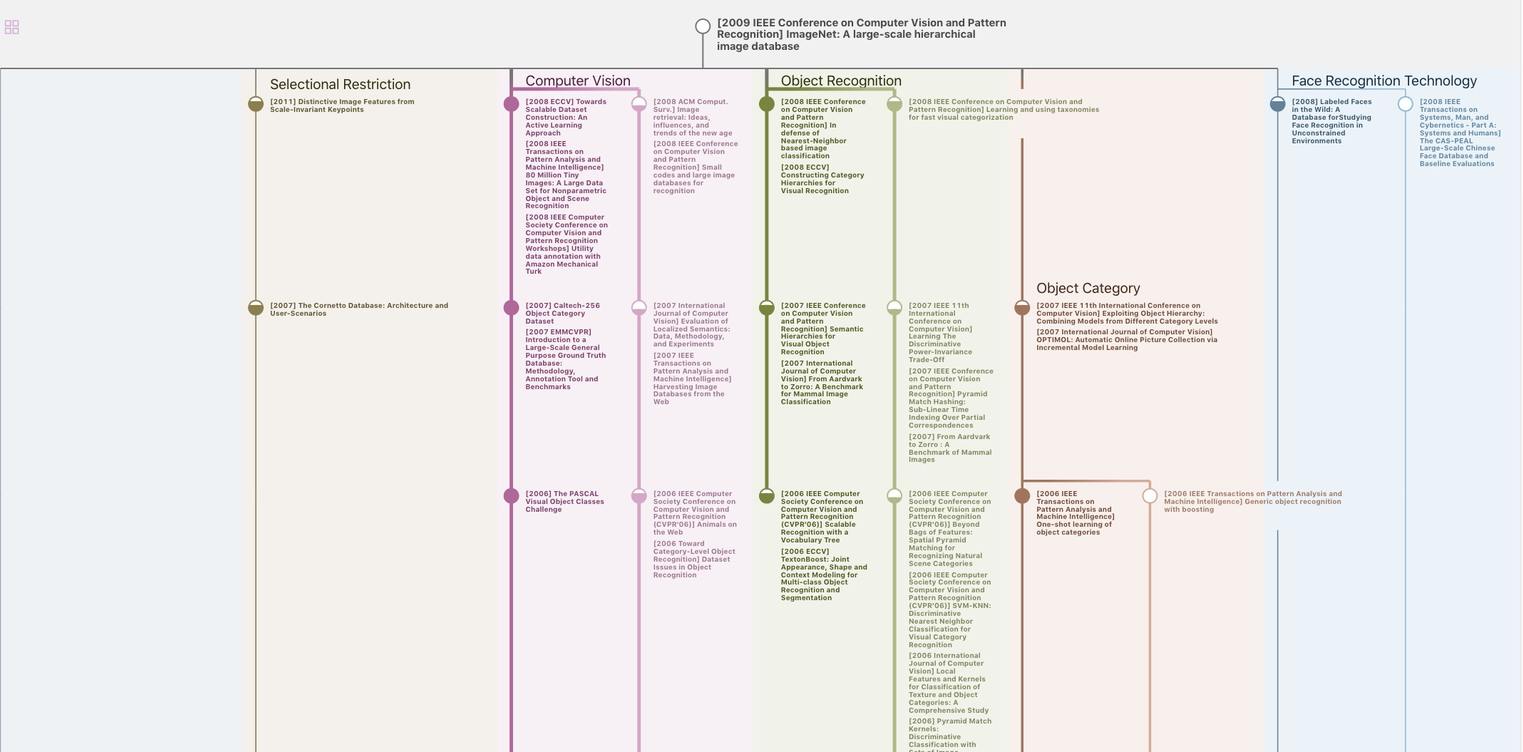
生成溯源树,研究论文发展脉络
Chat Paper
正在生成论文摘要