On Context Utilization in Summarization with Large Language Models
CoRR(2023)
摘要
Large language models (LLMs) excel in abstractive summarization tasks,
delivering fluent and pertinent summaries. Recent advancements have extended
their capabilities to handle long-input contexts, exceeding 100k tokens.
However, in question answering, language models exhibit uneven utilization of
their input context. They tend to favor the initial and final segments,
resulting in a U-shaped performance pattern concerning where the answer is
located within the input. This bias raises concerns, particularly in
summarization where crucial content may be dispersed throughout the source
document(s). Besides, in summarization, mapping facts from the source to the
summary is not trivial as salient content is usually re-phrased. In this paper,
we conduct the first comprehensive study on context utilization and position
bias in summarization. Our analysis encompasses 5 LLMs, 10 datasets, and 5
evaluation metrics. We introduce a new evaluation benchmark called MiddleSum on
the which we benchmark two alternative inference methods to alleviate position
bias: hierarchical summarization and incremental summarization.
更多查看译文
关键词
large language models,summarization,position bias
AI 理解论文
溯源树
样例
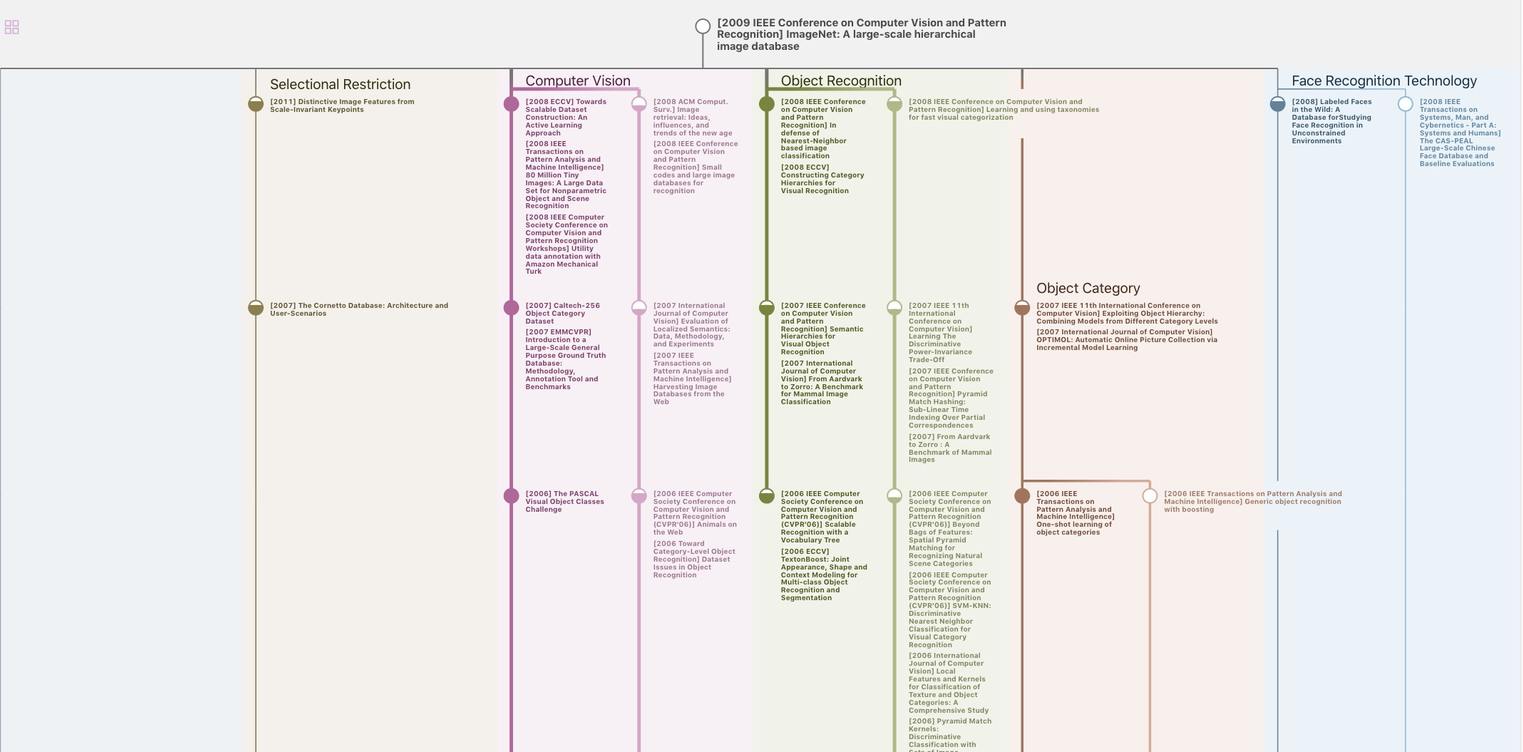
生成溯源树,研究论文发展脉络
Chat Paper
正在生成论文摘要