Learning Long-Range Information with Dual-Scale Transformers for Indoor Scene Completion
Proceedings of the IEEE/CVF International Conference on Computer Vision (ICCV)(2023)
摘要
Due to the limited resolution of 3D sensors and the inevitable mutual occlusion between objects, 3D scans of real scenes are commonly incomplete. Previous scene completion methods struggle to capture long-range spatial context, resulting in unsatisfactory completion results. To alleviate the problem, we propose a novel Dual-Scale Transformer Network (DST-Net) that efficiently utilizes both long-range and short-range spatial context information to improve the quality of 3D scene completion. To reduce the heavy computation cost of extracting long-range features via transformers, DST-Net adopts a self-supervised two-stage completion strategy. In the first stage, we split the input scene into blocks and perform completion on individual blocks. In the second stage, the blocks are merged together as a whole and then further refined to improve completeness. More importantly, we propose a contrastive attention training strategy to encourage the transformers to learn distinguishable features for better scene completion. Experiments on datasets of Matterport3D, ScanNet, and ICL-NUIM demonstrate that our method can generate better completion results, and our method outperforms the state-of-the-art methods quantitatively and qualitatively.
更多查看译文
关键词
Scene Reconstruction,Depth Estimation,3D Mapping,3D Reconstruction
AI 理解论文
溯源树
样例
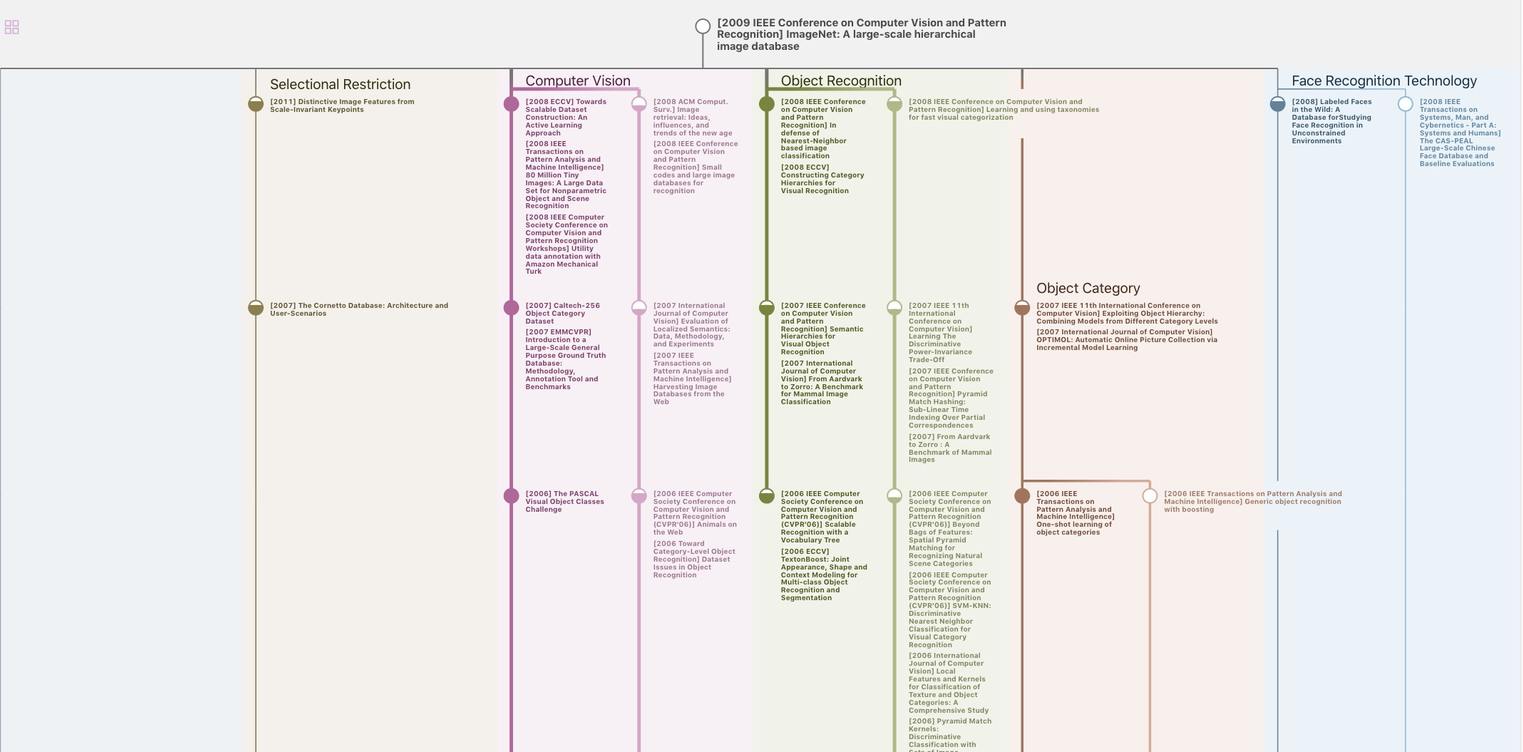
生成溯源树,研究论文发展脉络
Chat Paper
正在生成论文摘要