Efficient Integrators for Diffusion Generative Models.
ICLR(2024)
摘要
Diffusion models suffer from slow sample generation at inference time. Therefore, developing a principled framework for fast deterministic/stochastic sampling for a broader class of diffusion models is a promising direction. We propose two complementary frameworks for accelerating sample generation in pre-trained models: Conjugate Integrators and Splitting Integrators. Conjugate integrators generalize DDIM, mapping the reverse diffusion dynamics to a more amenable space for sampling. In contrast, splitting-based integrators, commonly used in molecular dynamics, reduce the numerical simulation error by cleverly alternating between numerical updates involving the data and auxiliary variables. After extensively studying these methods empirically and theoretically, we present a hybrid method that leads to the best-reported performance for diffusion models in augmented spaces. Applied to Phase Space Langevin Diffusion [Pandey & Mandt, 2023] on CIFAR-10, our deterministic and stochastic samplers achieve FID scores of 2.11 and 2.36 in only 100 network function evaluations (NFE) as compared to 2.57 and 2.63 for the best-performing baselines, respectively. Our code and model checkpoints will be made publicly available at \url{https://github.com/mandt-lab/PSLD}.
更多查看译文
关键词
Diffusion models,Deep Generative Models,Efficient Sampling
AI 理解论文
溯源树
样例
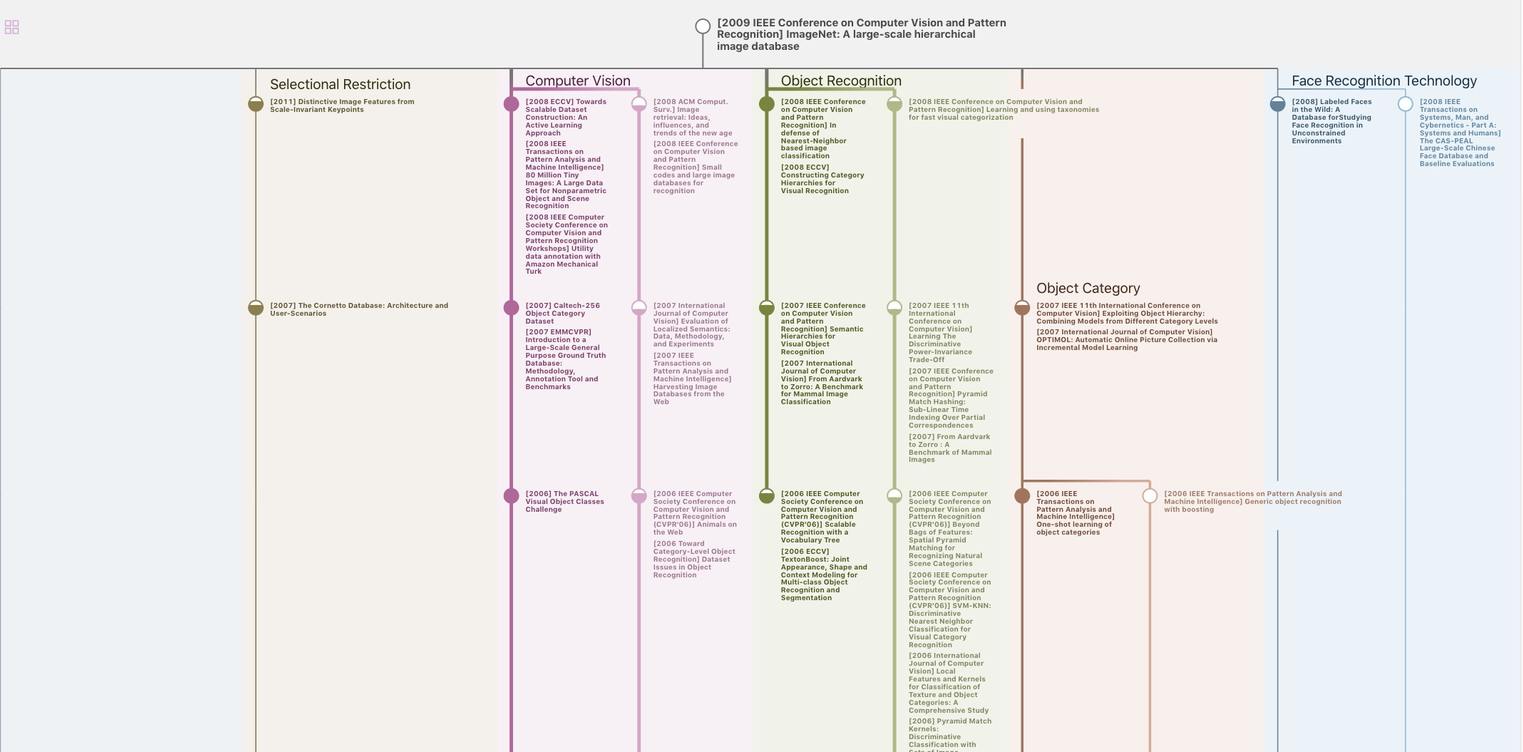
生成溯源树,研究论文发展脉络
Chat Paper
正在生成论文摘要