Fast Contingency Filtering Using Machine Learning for Power System Planning
2023 IEEE 11th International Conference on Smart Energy Grid Engineering (SEGE)(2023)
摘要
Modern power systems assess reliability $N -k$ and $k =\{ 2, 3, 4,\ldots\}$ criteria to guarantee the secure, sustainable and optimal operation of power networks. However, performing these studies with traditional methods is computation-intensive and time-prohibitive for the long-term planning of large networks. In this paper, we present a Machine Learning (ML) model for rapid contingency filtering in order to evaluate problematic $N - k$ contingency scenarios. Our proposed model is trained with data sets stochastically created and labeled with AC load flows considering load forecasting. Their inputs are the time and $N - \quad k$ status of network equipment. The performance of the proposed ML model was evaluated on the IEEE 39-Bus System for a planning period of 10yr with a time resolution of 1h. The performance obtained was an accuracy greater than 95% with a time acceleration of approx. 2500x. This result makes the proposed model suitable for supporting decision making during the planning of power systems.
更多查看译文
关键词
Asset Management,Machine Learning,Probabilistic Reliability Assessment,Transmission Planning
AI 理解论文
溯源树
样例
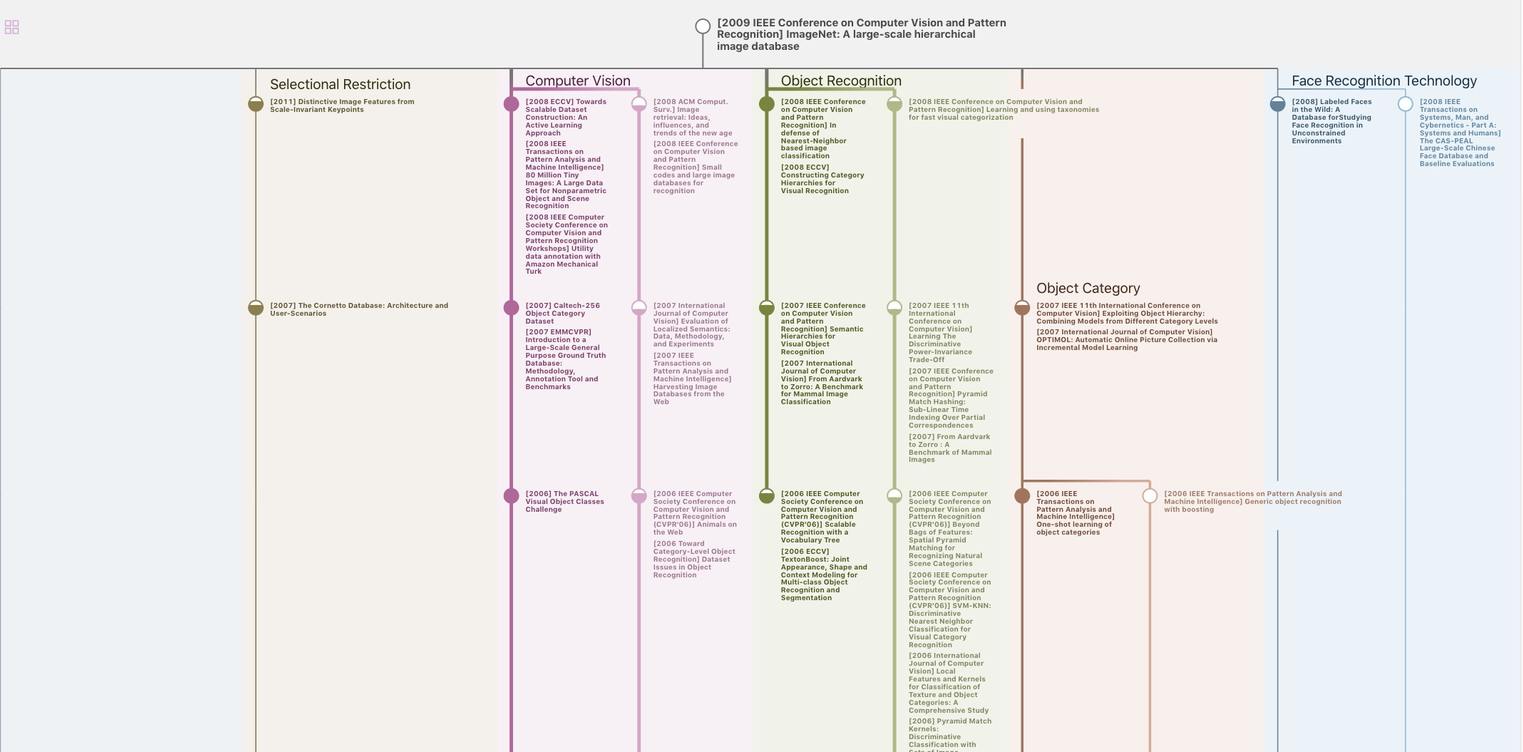
生成溯源树,研究论文发展脉络
Chat Paper
正在生成论文摘要