Identifying Epileptogenic Abnormality by Decomposing Intracranial EEG and MEG Power Spectra
JOURNAL OF NEUROSCIENCE METHODS(2024)
摘要
Background: Accurate identification of abnormal electroencephalographic (EEG) activity is pivotal for diagnosing and treating epilepsy. Recent studies indicate that decomposing brain activity into periodic (oscillatory) and aperiodic (trend across all frequencies) components can illuminate the drivers of spectral activity changes. New methods: We analysed intracranial EEG (iEEG) data from 234 subjects, creating a normative map. This map was compared to a cohort of 63 patients with refractory focal epilepsy under consideration for neurosurgery. The normative map was computed using three approaches: (i) relative complete band power, (ii) relative band power with the aperiodic component removed, and (iii) the aperiodic exponent. Abnormalities were calculated for each approach in the patient cohort. We evaluated the spatial profiles, assessed their ability to localize abnormalities, and replicated the findings using magnetoencephalography (MEG). Results: Normative maps of relative complete band power and relative periodic band power exhibited similar spatial profiles, while the aperiodic normative map revealed higher exponent values in the temporal lobe. Abnormalities estimated through complete band power effectively distinguished between good and bad outcome patients. Combining periodic and aperiodic abnormalities enhanced performance, like the complete band power approach. Comparison with existing methods and conclusions: Sparing cerebral tissue with abnormalities in both periodic and aperiodic activity may result in poor surgical outcomes. Both periodic and aperiodic components do not carry sufficient information in isolation. The relative complete band power solution proved to be the most reliable method for this purpose. Future studies could investigate how cerebral location or pathology influences periodic or aperiodic abnormalities.
更多查看译文
关键词
Normative mapping,IEEG,MEG,Power spectrum decomposition,1/f exponent,Epilepsy
AI 理解论文
溯源树
样例
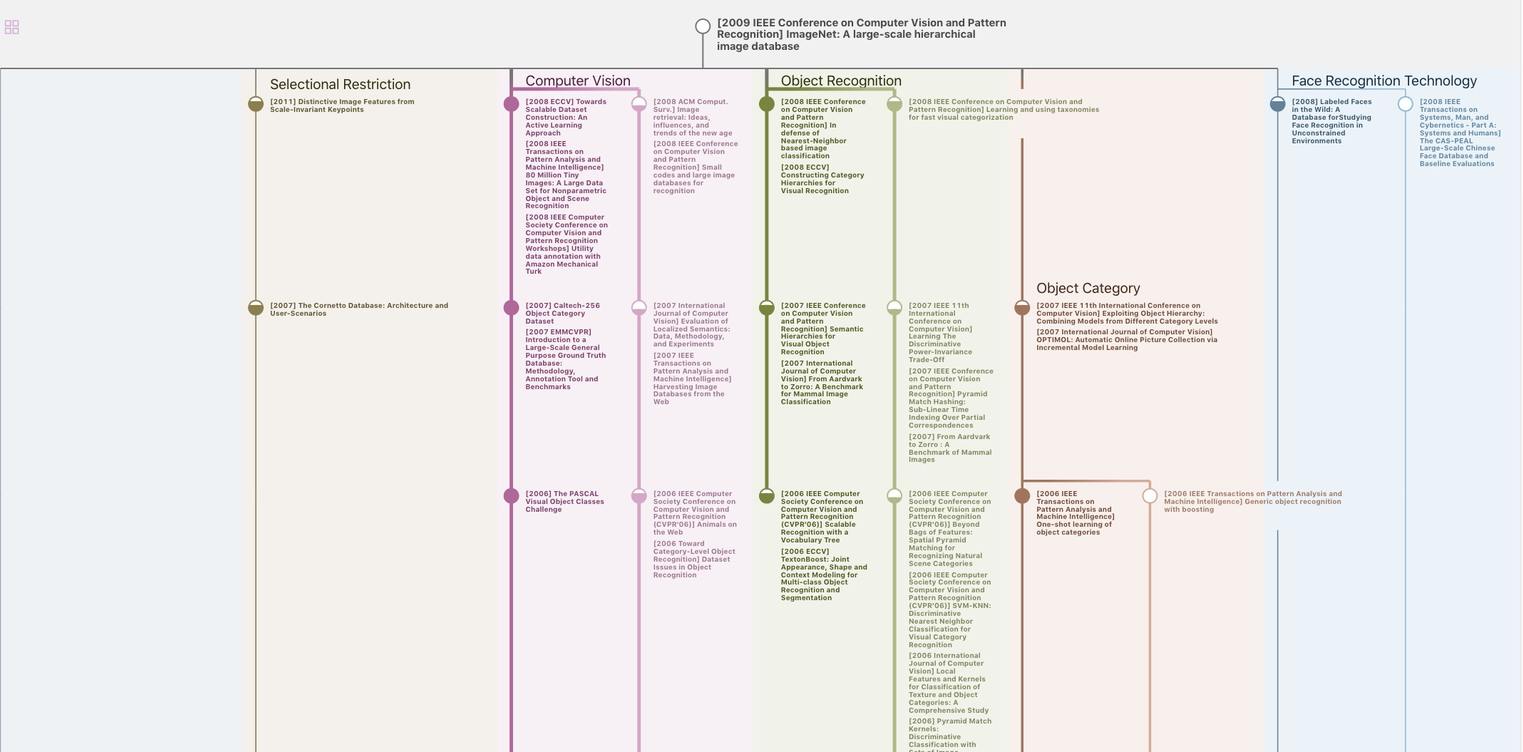
生成溯源树,研究论文发展脉络
Chat Paper
正在生成论文摘要