Multimodal Sensor Data Fusion and Ensemble Modeling for Human Locomotion Activity Recognition
Adjunct Proceedings of the 2023 ACM International Joint Conference on Pervasive and Ubiquitous Computing & the 2023 ACM International Symposium on Wearable Computing(2023)
摘要
The primary research objective of this study is to develop an algorithm pipeline for recognizing human locomotion activities using multimodal sensor data from smartphones, while minimizing prediction errors due to data differences between individuals. The multimodal sensor data provided for the 2023 SHL recognition challenge comprises three types of motion data and two types of radio sensor data. Our team, ‘HELP,’ presents an approach that aligns all the multimodal data to derive a form of vector composed of 106 features, and then blends predictions from multiple learning models which are trained using different number of feature vectors. The proposed neural network models, trained solely on data from a specific individual, yield F1 scores of up to 0.8 in recognizing the locomotion activities of other users. Through post-processing operations, including the ensemble of multiple learning models, it is expected to achieve a performance improvement of 10% or greater in terms of F1 score.
更多查看译文
关键词
Activity Recognition,Accelerometer Data,Cross-View Recognition,Human Identification,Ambient Intelligence
AI 理解论文
溯源树
样例
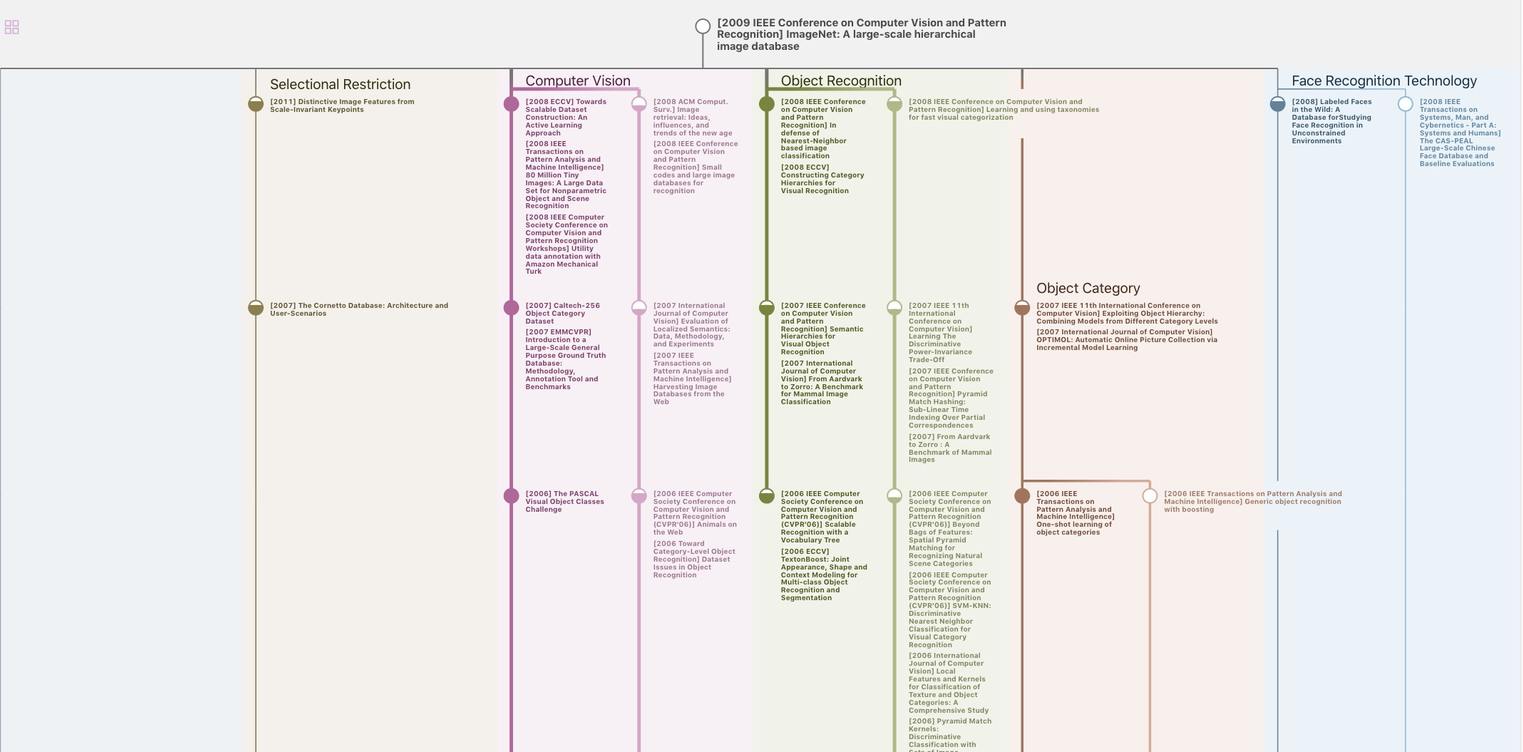
生成溯源树,研究论文发展脉络
Chat Paper
正在生成论文摘要