Explainable Claim Verification via Knowledge-Grounded Reasoning with Large Language Models.
CoRR(2023)
摘要
Claim verification plays a crucial role in combating misinformation. While existing works on claim verification have shown promising results, a crucial piece of the puzzle that remains unsolved is to understand how to verify claims without relying on human-annotated data, which is expensive to create at a large scale. Additionally, it is important for models to provide comprehensive explanations that can justify their decisions and assist human fact-checkers. This paper presents First-Order-Logic-Guided Knowledge-Grounded (FOLK) Reasoning that can verify complex claims and generate explanations without the need for annotated evidence using Large Language Models (LLMs). FOLK leverages the in-context learning ability of LLMs to translate the claim into a First-Order-Logic (FOL) clause consisting of predicates, each corresponding to a sub-claim that needs to be verified. Then, FOLK performs FOL-Guided reasoning over a set of knowledge-grounded question-and-answer pairs to make veracity predictions and generate explanations to justify its decision-making process. This process makes our model highly explanatory, providing clear explanations of its reasoning process in human-readable form. Our experiment results indicate that FOLK outperforms strong baselines on three datasets encompassing various claim verification challenges. Our code and data are available.
更多查看译文
关键词
explainable claim verification,large language models,reasoning,knowledge-grounded
AI 理解论文
溯源树
样例
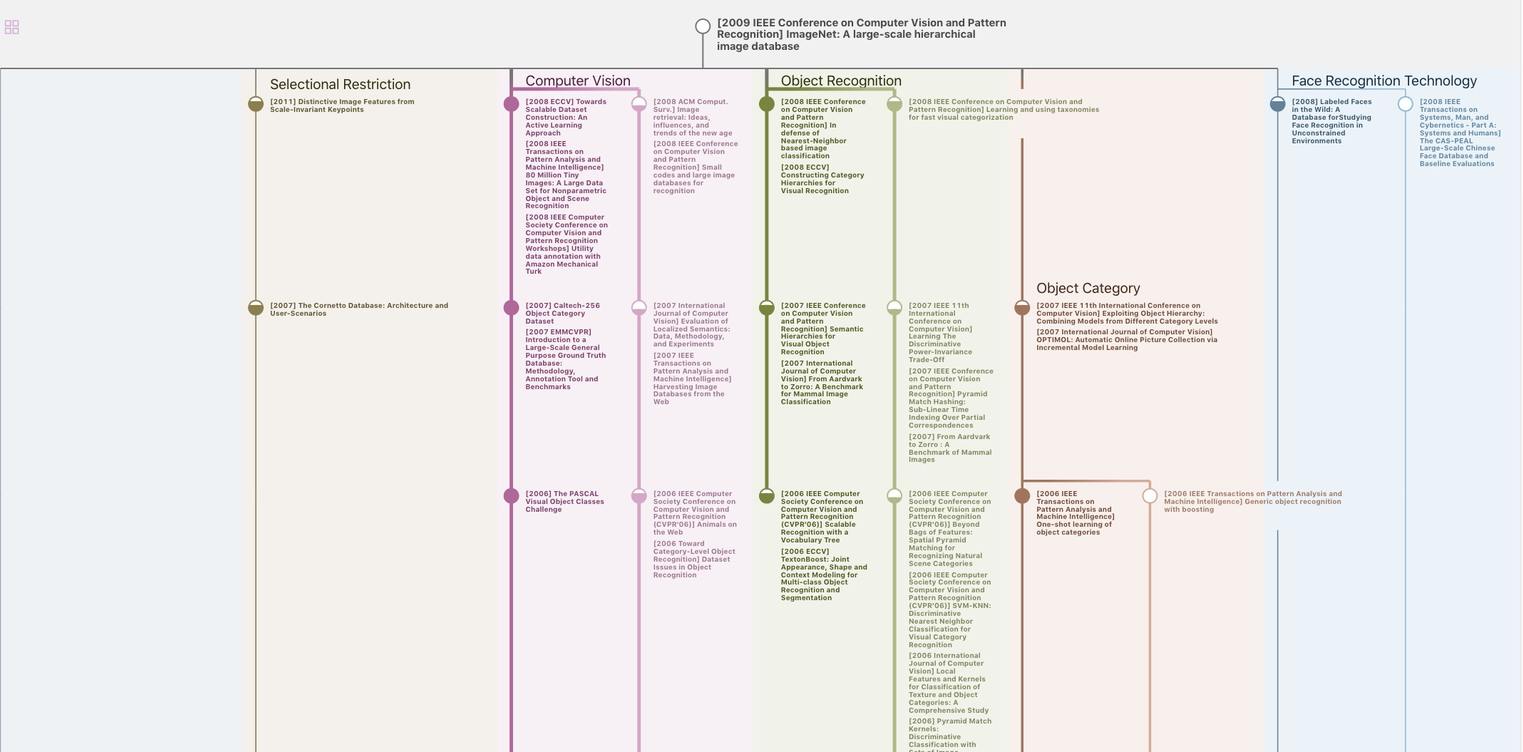
生成溯源树,研究论文发展脉络
Chat Paper
正在生成论文摘要