Learning Many-to-Many Mapping for Unpaired Real-World Image Super-resolution and Downscaling
IEEE Transactions on Pattern Analysis and Machine Intelligence(2024)
摘要
Learning based single image super-resolution (SISR) for real-world images has been an active research topic yet a challenging task, due to the lack of paired low-resolution (LR) and high-resolution (HR) training images. Most of the existing unsupervised real-world SISR methods adopt a two-stage training strategy by synthesizing realistic LR images from their HR counterparts first, then training the super-resolution (SR) models in a supervised manner. However, the training of image degradation and SR models in this strategy are separate, ignoring the inherent mutual dependency between downscaling and its inverse upscaling process. Additionally, the ill-posed nature of image degradation is not fully considered. In this paper, we propose an image downscaling and SR model dubbed as SDFlow, which simultaneously learns a bidirectional many-to-many mapping between real-world LR and HR images unsupervisedly. The main idea of SDFlow is to decouple image content and degradation information in the latent space, where content information distribution of LR and HR images is matched in a common latent space. Degradation information of the LR images and the high-frequency information of the HR images are fitted to an easy-to-sample conditional distribution. Experimental results on real-world image SR datasets indicate that SDFlow can generate diverse realistic LR and SR images both quantitatively and qualitatively.
更多查看译文
关键词
Super-Resolution,Unsupervised Learning,Sparse Representations,Image Processing,Visual Servoing
AI 理解论文
溯源树
样例
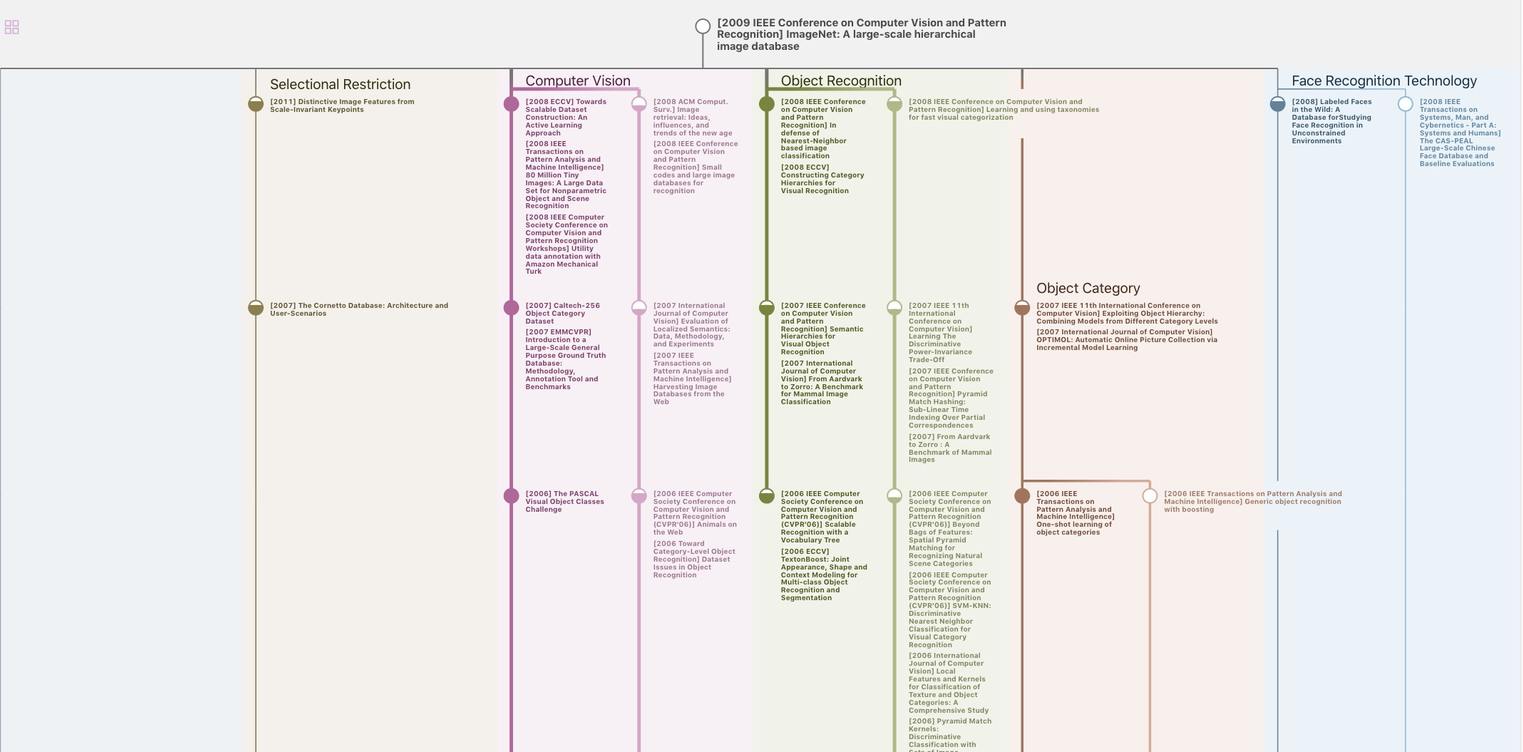
生成溯源树,研究论文发展脉络
Chat Paper
正在生成论文摘要