Interpretable, Verifiable, and Robust Reinforcement Learning Via Program Synthesis
XXAI - BEYOND EXPLAINABLE AI International Workshop, Held in Conjunction with ICML 2020, July 18, 2020, Vienna, Austria, Revised and Extended Papers(2022)
摘要
Reinforcement learning is a promising strategy for automatically training policies for challenging control tasks. However, state-of-the-art deep reinforcement learning algorithms focus on training deep neural network (DNN) policies, which are black box models that are hard to interpret and reason about. In this chapter, we describe recent progress towards learning policies in the form of programs. Compared to DNNs, such programmatic policies are significantly more interpretable, easier to formally verify, and more robust. We give an overview of algorithms designed to learn programmatic policies, and describe several case studies demonstrating their various advantages.
更多查看译文
关键词
Interpretable reinforcement learning,Program synthesis
AI 理解论文
溯源树
样例
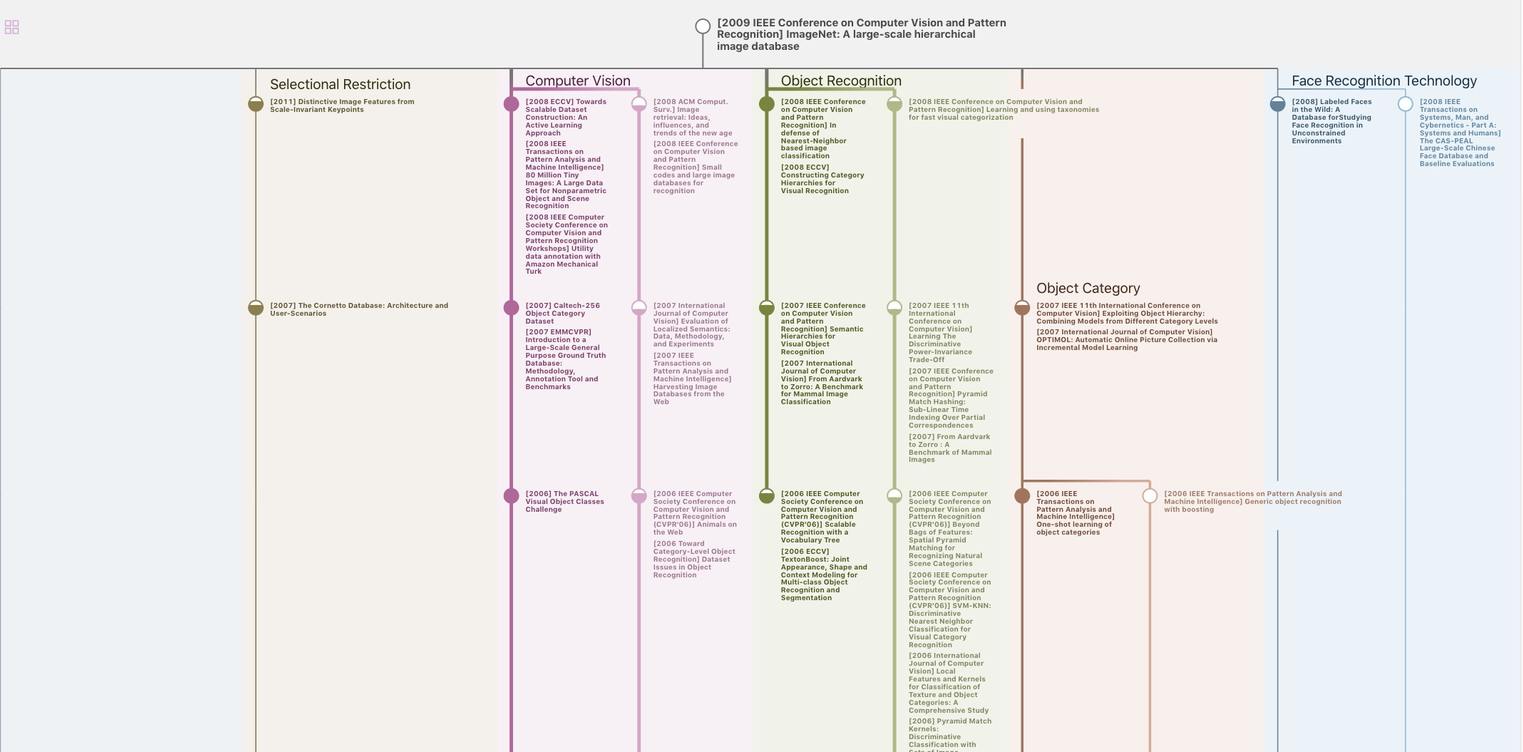
生成溯源树,研究论文发展脉络
Chat Paper
正在生成论文摘要