Research on Modeling Weighted Average Temperature Based on the Machine Learning Algorithms
ATMOSPHERE(2023)
摘要
In response to the nonlinear fitting difficulty of the traditional weighted average temperature (T-m) modeling, this paper proposed four machine learning (ML)-based T-m models. Based on the seven radiosondes in the Yangtze River Delta region from 2014 to 2019, four forecasting ML-based T-m models were constructed using Light Gradient Boosting Machine (LightGBM), Support Vector Machine (SVM), Random Forest (RF), and Classification and Regression Tree (CART) algorithms. The surface temperature (Ts), water vapor pressure (Es), and atmospheric pressure (Ps) were identified as crucial influencing factors after analyzing their correlations to the T-m. The ML-based T-m models were trained using seven radiosondes from 2014 to 2018. Then, the mean bias and root mean square error (RMSE) of the 2019 dataset were used to evaluate the accuracy of the ML-based T-m models. Experimental results show that the overall accuracy of the LightGBM-based T-m model is superior to the SVM, CART, and RF-based Tm models under different temporal variations. The mean RMSE of the daily LightGBM-based T-m model is reduced by 0.07 K, 0.04 K, and 0.13 K compared to the other three ML-based models, respectively. The mean RMSE of the monthly LightGBM-based T-m model is reduced by 0.09 K, 0.04 K, and 0.11 K, respectively. The mean RMSE of the quarterly LightGBM-based T-m model is reduced by 0.09 K, 0.04 K, and 0.11 K, respectively. The mean bias of the LightGBM-based T-m model is also smaller than that of the other ML-based T-m models. Therefore, the LightGBM-based T-m model can provide more accurate T-m and is more suitable for obtaining GNSS precipitable water vapor in the Yangtze River Delta region.
更多查看译文
关键词
average temperature,machine learning algorithms,modeling weighted,machine learning
AI 理解论文
溯源树
样例
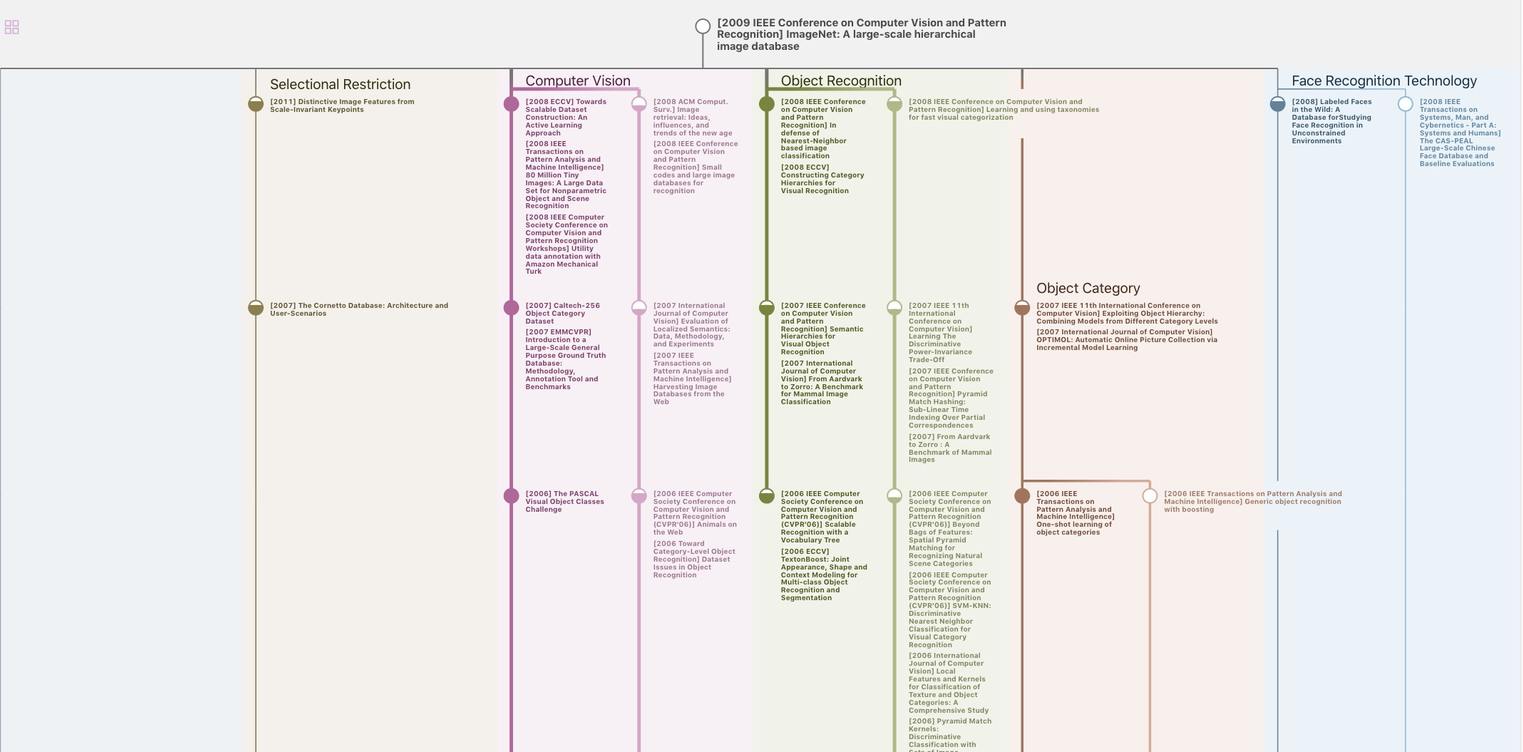
生成溯源树,研究论文发展脉络
Chat Paper
正在生成论文摘要