Predicting the Performance of Green Stormwater Infrastructure Using Multivariate Long Short-Term Memory (LSTM) Neural Network
JOURNAL OF HYDROLOGY(2023)
摘要
The expected performance of Green Stormwater Infrastructure (GSI) is typically quantified through numerical models based on hydrologic parameters and physics-based equations. With numerical models, the choice of a spatio-temporal discretization scheme for the computational domain is a strenuous task that requires extensive calibration and potentially lab-based parameters and experimentation. The performance of GSI has high temporal dynamics due to natural, anthropogenic, and climatic processes that are not well represented by the traditional physics-based hydrologic models, which are calibrated against only a few historical observations and have a user-defined and constrained set of computational outcomes. Deep learning-based predictive models, such as Long Short-Term Memory (LSTM) neural networks, offer an exciting opportunity to quantify GSI performance, accounting for its highly dynamic and constantly evolving nature by leveraging advancements in observational data. A LSTM regression can overcome some of the limitations associated with traditional hydrological models to aid the development of a fully data-informed GSI performance predictor. To demonstrate the LSTM and traditional model outcomes, both methods were applied to a rain garden in Villanova, PA, USA. Specifically, an LSTM model was used to predict the recession of ponded water depth in the rain garden using five years of observed data. A set of eight predictors (i.e., precipitation, air temperature, soil temperature, soil moisture content at a depth of 10 cm, 35 cm, 65 cm and 91 cm, water depth) and a target variable (i.e., recession rate) were considered for training/testing the LSTM model. A comparative study with the USEPA Storm Water Management Model (SWMM) was performed to observe the performance of a physics-based model and a LSTM model for continuous recession rate time series and specific storms. The LSTM model had a performance score, Root Mean Square Error (RMSE), of 0.081 for the continuous time series, outperforming the SWMM performance with a score of 2.173 when compared to observed data. In the case of storm-specific prediction, LSTM also outperformed SWMM simulation for four storms with lower RMSE values when compared to the observed data. The application of the LSTM model in predicting GSI performance is a crucial stride towards efficient real-time forecasting.
更多查看译文
关键词
Green Stormwater Infrastructure (GSI),Recession rates,Storm Water Management Model (SWMM),Deep learning,LSTM
AI 理解论文
溯源树
样例
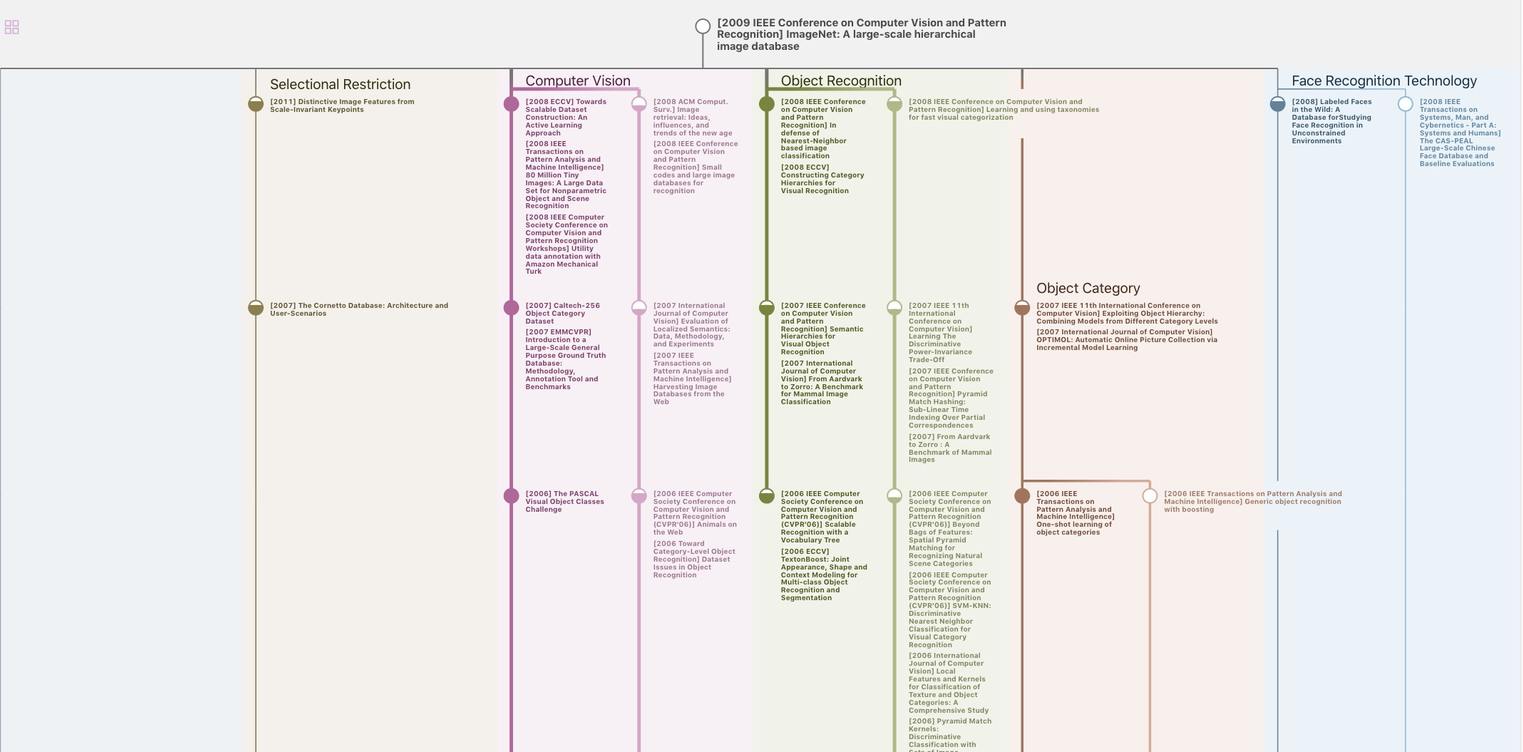
生成溯源树,研究论文发展脉络
Chat Paper
正在生成论文摘要