TapFinger: Task Placement and Fine-Grained Resource Allocation for Edge Machine Learning.
INFOCOM(2023)
摘要
Machine learning (ML) tasks are one of the major workloads in today's edge computing networks. Existing edge-cloud schedulers allocate the requested amounts of resources to each task, falling short of best utilizing the limited edge resources flexibly for ML task performance optimization. This paper proposes TapFinger, a distributed scheduler that minimizes the total completion time of ML tasks in a multi-cluster edge network, through co-optimizing task placement and fine-grained multi-resource allocation. To learn the tasks' uncertain resource sensitivity and enable distributed online scheduling, we adopt multi-agent reinforcement learning (MARL), and propose several techniques to make it efficient for our ML-task resource allocation. First, TapFinger uses a heterogeneous graph attention network as the MARL backbone to abstract inter-related state features into more learnable environmental patterns. Second, the actor network is augmented through a tailored task selection phase, which decomposes the actions and encodes the optimization constraints. Third, to mitigate decision conflicts among agents, we novelly combine Bayes' theorem and masking schemes to facilitate our MARL model training. Extensive experiments using synthetic and test-bed ML task traces show that TapFinger can achieve up to 28.6% reduction in the average task completion time and improve resource efficiency as compared to state-of-the- art resource schedulers.
更多查看译文
关键词
actor network,art resource schedulers,average task completion time,co-optimizing task placement,distributed scheduler,edge computing networks,edge machine learning,edge resources,existing edge-cloud schedulers,fine-grained multiresource allocation,fine-grained resource allocation,heterogeneous graph attention network,machine learning tasks,ML task performance optimization,ML tasks,ML-task resource allocation,multiagent reinforcement learning,multicluster edge network,optimization constraints,resource efficiency,tailored task selection phase,TapFinger,test-bed ML task traces,total completion time
AI 理解论文
溯源树
样例
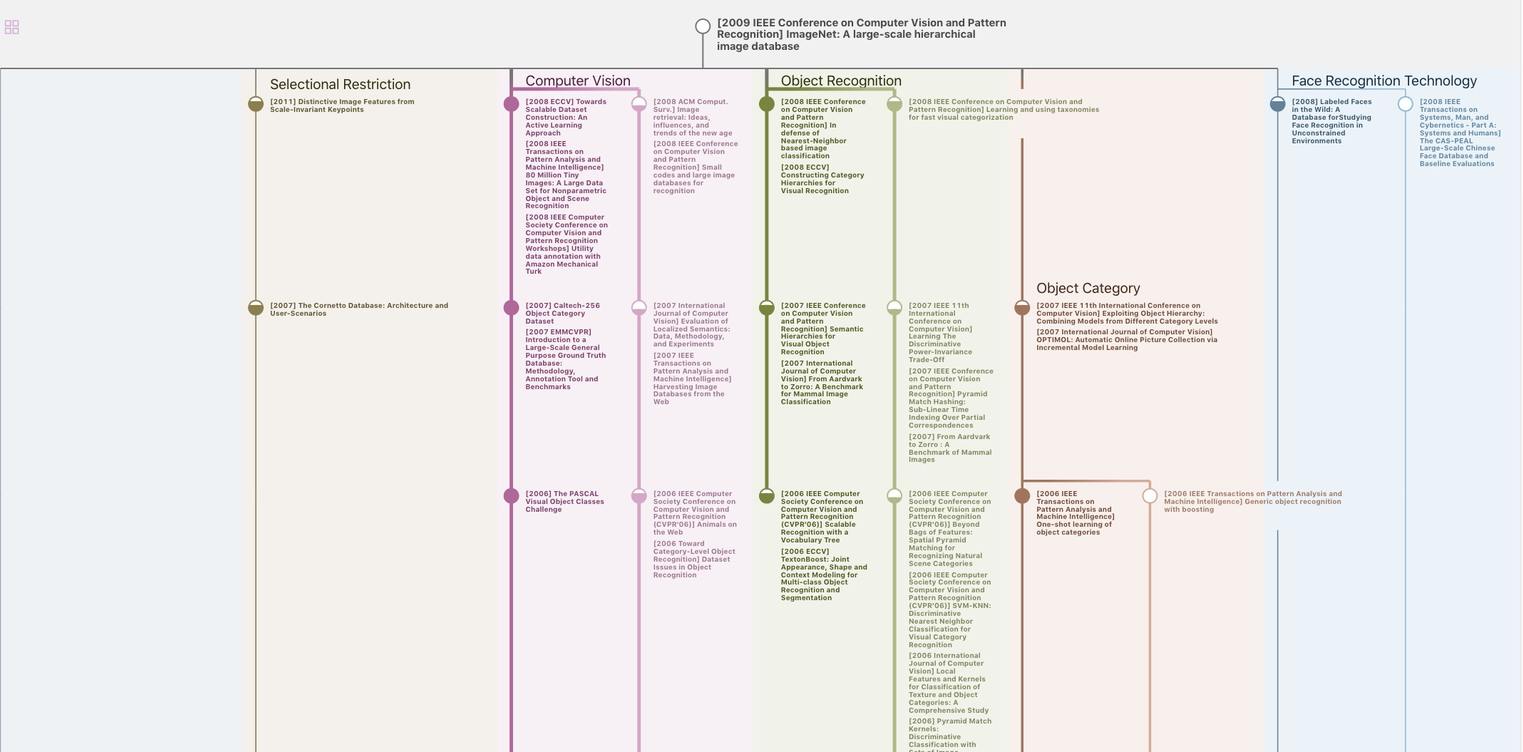
生成溯源树,研究论文发展脉络
Chat Paper
正在生成论文摘要