Incentivizing Exploration in Linear Contextual Bandits under Information Gap
PROCEEDINGS OF THE 17TH ACM CONFERENCE ON RECOMMENDER SYSTEMS, RECSYS 2023(2023)
摘要
Contextual bandit algorithms have been popularly used to address interactive recommendation, where the users are assumed to be cooperative to explore all recommendations from a system. In this paper, we relax this strong assumption and study the problem of incentivized exploration with myopic users, where the users are only interested in recommendations with their currently highest estimated reward. As a result, in order to obtain long-term optimality, the system needs to offer compensation to incentivize the users to take the exploratory recommendations. We consider a new and practically motivated setting where the context features employed by the user are more informative than those used by the system: for example, features based on users' private information are not accessible by the system. We develop an effective solution for incentivized exploration under such an information gap, and prove that the method achieves a sublinear rate in both regret and compensation. We theoretically and empirically analyze the added compensation due to the information gap, compared with the case where the system has access to the same context features as the user does, i.e., without information gap. Moreover, we also provide a compensation lower bound of this problem.
更多查看译文
关键词
Incentivized exploration,linear bandits,information gap
AI 理解论文
溯源树
样例
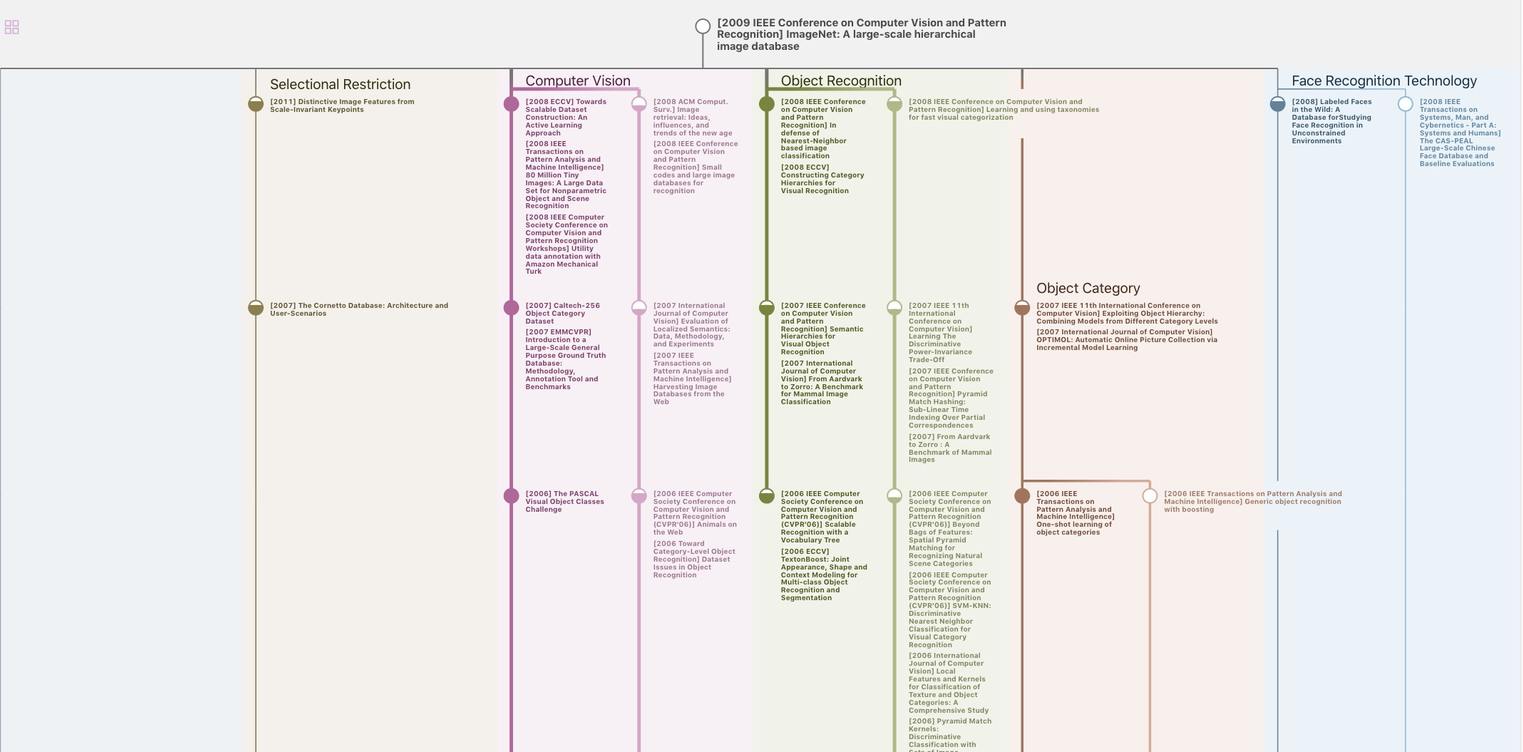
生成溯源树,研究论文发展脉络
Chat Paper
正在生成论文摘要