Using Pre-Trained Language Models for Abstractive DBPEDIA Summarization: A Comparative Study
KNOWLEDGE GRAPHS: SEMANTICS, MACHINE LEARNING, AND LANGUAGES(2023)
摘要
Purpose: This study addresses the limitations of current short abstracts of DBPEDIA entities, which often lack a comprehensive overview due to their creating method (i.e., selecting the first two-three sentences from the full DBPEDIA abstracts). Methodology: We leverage pre-trained language models to generate abstractive summaries of DBPEDIA abstracts in six languages (English, French, German, Italian, Spanish, and Dutch). We performed several experiments to assess the quality of generated summaries by language models. In particular, we evaluated the generated summaries using human judgments and automated metrics (Self-ROUGE and BERTScore). Additionally, we studied the correlation between human judgments and automated metrics in evaluating the generated summaries under different aspects: informativeness, coherence, conciseness, and fluency. Findings: Pre-trained language models generate summaries more concise and informative than existing short abstracts. Specifically, BART-based models effectively overcome the limitations of DBPEDIA short abstracts, especially for longer ones. Moreover, we show that BERTScore and ROUGE-1 are reliable metrics for assessing the informativeness and coherence of the generated summaries with respect to the full DBPEDIA abstracts. We also find a negative correlation between conciseness and human ratings. Furthermore, fluency evaluation remains challenging without human judgment. Value: This study has significant implications for various applications in machine learning and natural language processing that rely on DBPEDIA resources. By providing succinct and comprehensive summaries, our approach enhances the quality of DBPEDIA abstracts and contributes to the semantic web community.
更多查看译文
关键词
Abstractive Summarization,Large Language Models,Knowledge Graphs
AI 理解论文
溯源树
样例
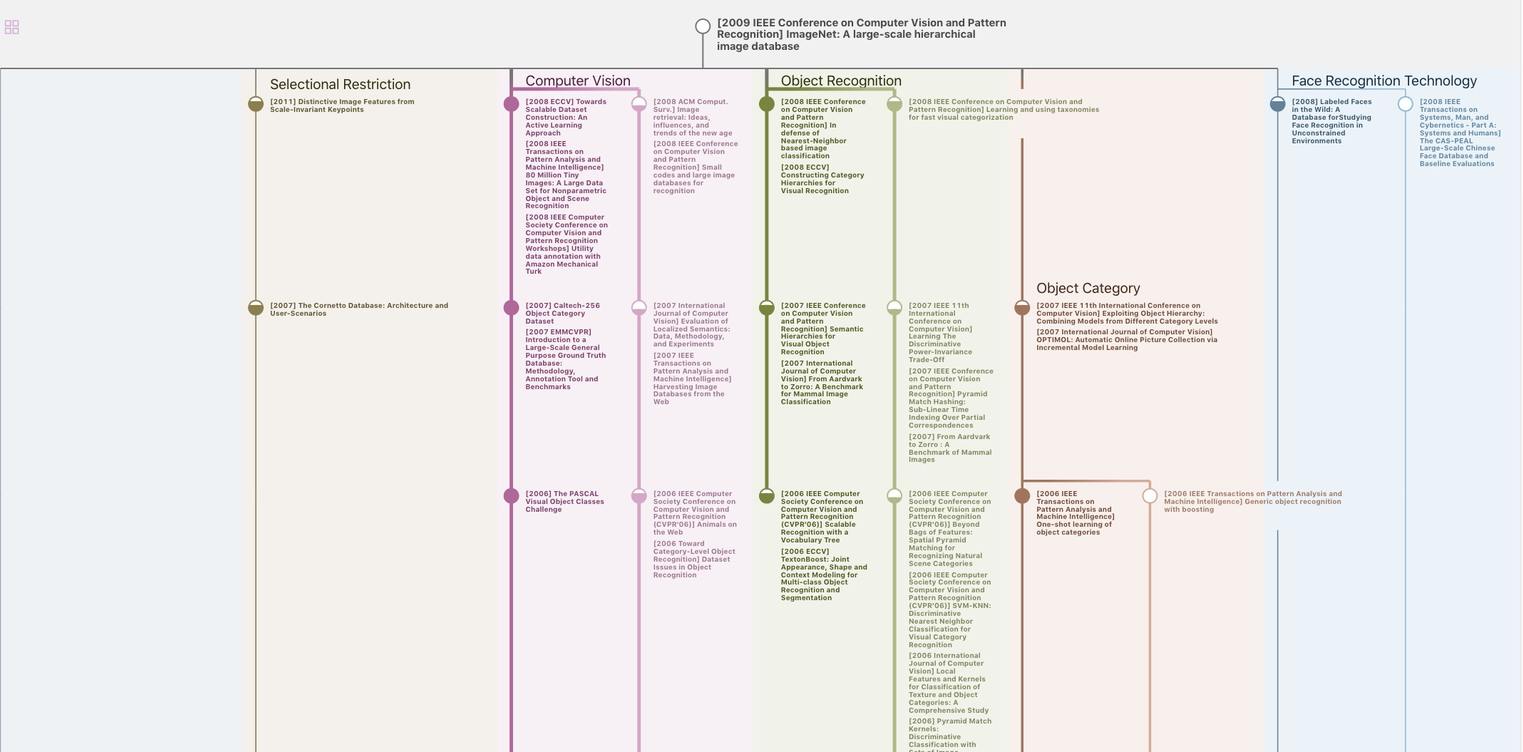
生成溯源树,研究论文发展脉络
Chat Paper
正在生成论文摘要