Generative hyperelasticity with physics-informed probabilistic diffusion fields
Engineering with Computers(2024)
Abstract
Many natural materials exhibit highly complex, nonlinear, anisotropic, and heterogeneous mechanical properties. Recently, it has been demonstrated that data-driven strain energy functions possess the flexibility to capture the behavior of these complex materials with high accuracy while satisfying physics-based constraints. However, most of these approaches disregard the uncertainty in the estimates and the spatial heterogeneity of these materials. In this work, we leverage recent advances in generative models to address these issues. We use as building block neural ordinary equations (NODE) that—by construction—create polyconvex strain energy functions, a key property of realistic hyperelastic material models. We combine this approach with probabilistic diffusion models to generate new samples of strain energy functions. This technique allows us to sample a vector of Gaussian white noise and translate it to NODE parameters thereby representing plausible strain energy functions. We extend our approach to spatially correlated diffusion resulting in heterogeneous material properties for arbitrary geometries. We extensively test our method with synthetic and experimental data on biological tissues and run finite element simulations with various degrees of spatial heterogeneity. We believe this approach is a major step forward including uncertainty in predictive, data-driven models of hyperelasticity.
MoreTranslated text
Key words
Hyperelasticity,Generative modeling,Neural ODEs,Data-driven modeling,Heterogeneous materials,Polyconvex,Hyper-network
AI Read Science
Must-Reading Tree
Example
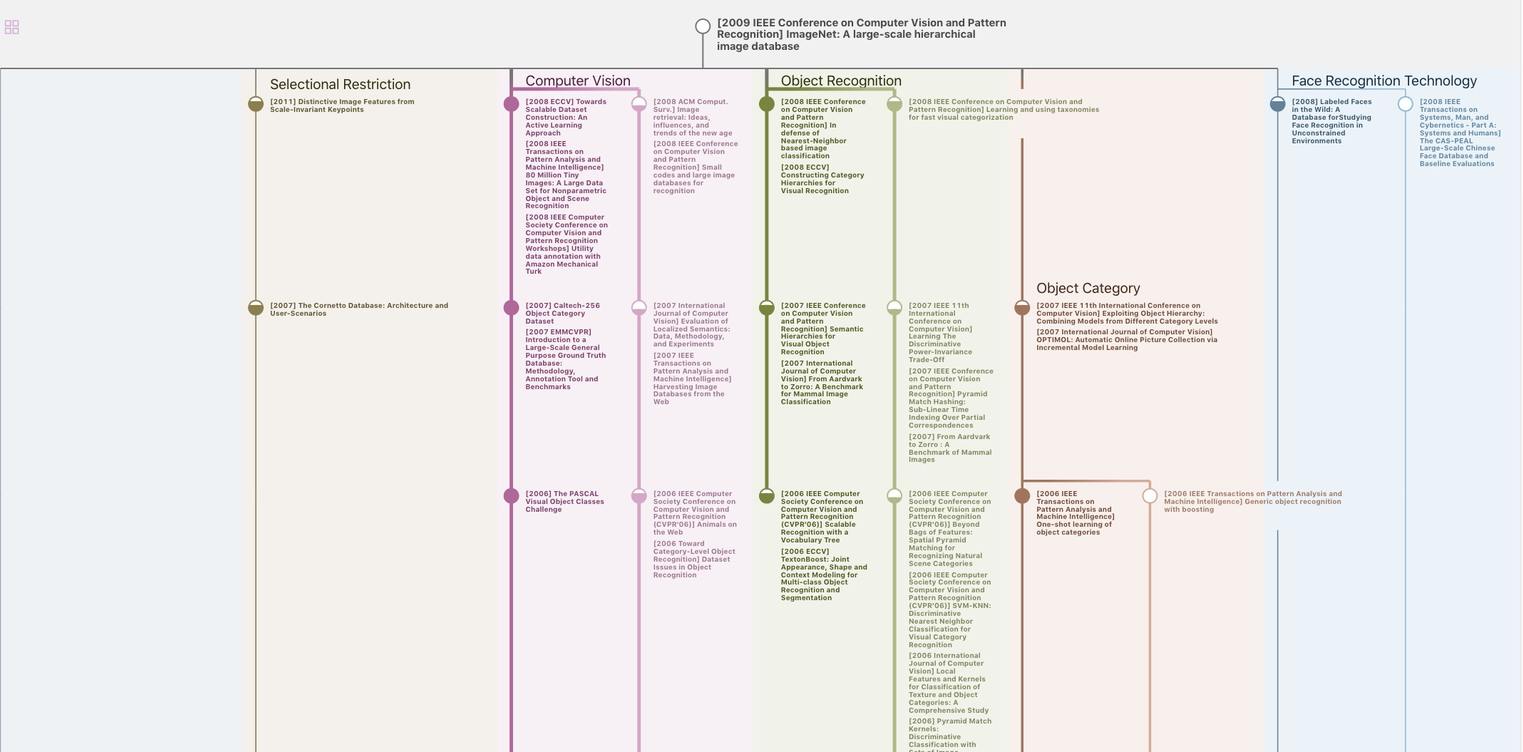
Generate MRT to find the research sequence of this paper
Chat Paper
Summary is being generated by the instructions you defined