A Double Association-Based Evolutionary Algorithm for Many-Objective Optimization
Mathematical Biosciences and Engineering(2023)
摘要
In this paper, a double association-based evolutionary algorithm (denoted as DAEA) is proposed to solve many-objective optimization problems. In the proposed DAEA, a double association strategy is designed to associate solutions with each subspace. Different from the existing association methods, the double association strategy takes the empty subspace into account and associates it with a promising solution, which can facilitate the exploration of unknown areas. Besides, a new quality evaluation scheme is developed to evaluate the quality of each solution in subspace, where the convergence and diversity of each solution is first measured, and in order to evaluate the diversity of solutions more finely, the global diversity and local diversity is designed to measure the diversity of each solution. Then, a dynamic penalty coefficient is designed to balance the convergence and diversity by penalizing the global diversity distribution of solutions. The performance of DAEA is validated by comparing with five state-of-the-art many-objective evolutionary algorithms on a number of well-known benchmark problems with up to 20 objectives. Experimental results show that our DAEA has high competitiveness in solving many-objective optimizatiopn problems compared with the other compared algorithms.
更多查看译文
关键词
many-objective optimization,double association,quality evaluation,convergence,diversity
AI 理解论文
溯源树
样例
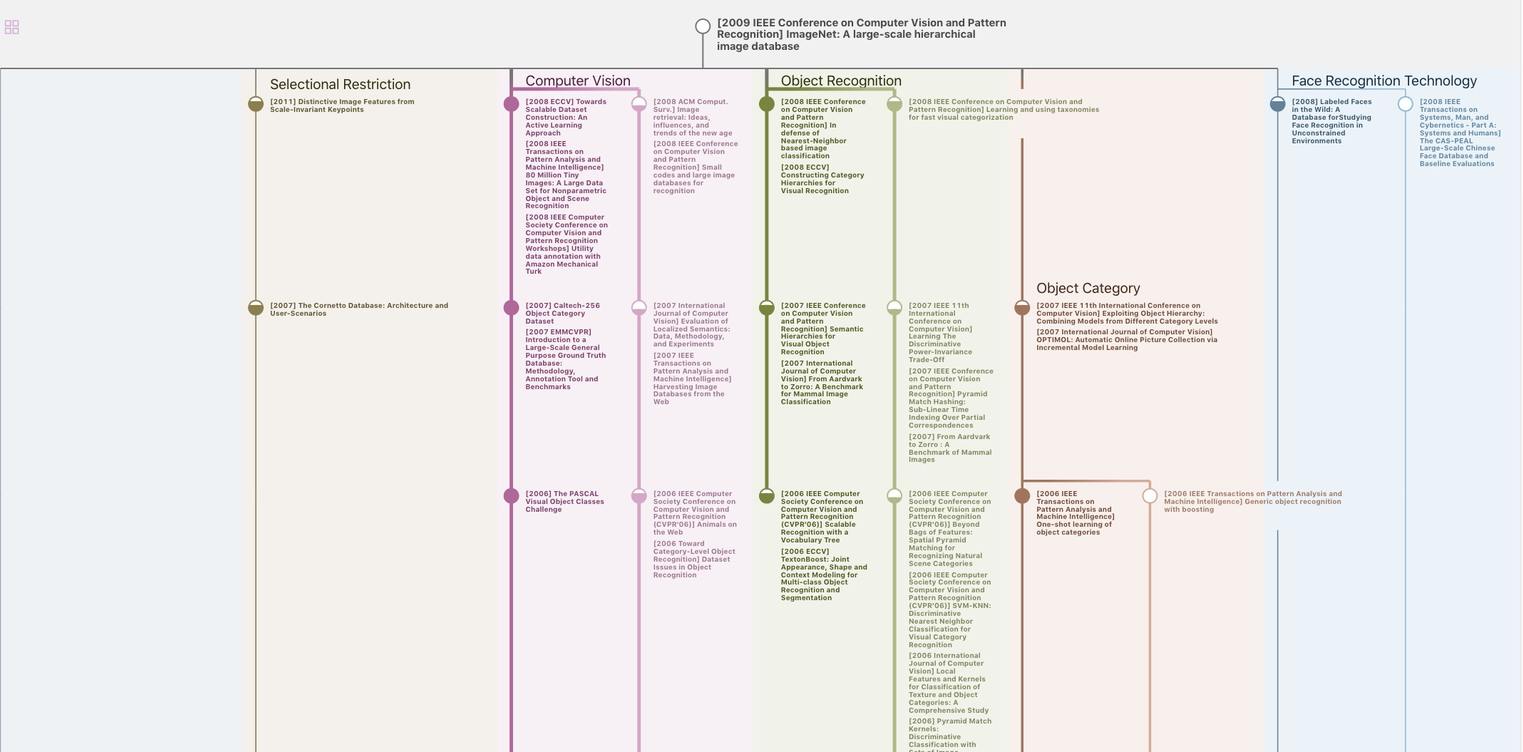
生成溯源树,研究论文发展脉络
Chat Paper
正在生成论文摘要