Mask Generation with Meta-Learning Classifier Weight Transformer Network for Few-Shot Image Segmentation
ICCE-Taiwan(2023)
摘要
This paper proposes a meta-learning classification weight transfer network to generate masks as a few-shot image segmentation architecture. It generates good prior masks via a pretrained classification weight transfer architecture, and uses a pretrained feature extraction architecture on query images and support images. The network architecture exploits a top-down path in a feature augmentation module to adaptively transfer information from fine to coarse features for extracting features from query images. Finally, the classification module predicts the segmentation of the query image. The experimental results show that using the mean intersection of joints (mIOU) as the evaluation mechanism, the accuracy of the 1-shot experimental results is 1.7% higher than that of the baseline. In the 5-shot experimental results, the accuracy is also improved by 2.6%. Therefore, compared with the baseline, it clearly shows that the mask generated by the meta-learning classification weight transfer network can effectively help improve the performance of few-shot image segmentation system.
更多查看译文
关键词
meta-learning,few-shot image segmentation,semantic segmentation,few-shot learning
AI 理解论文
溯源树
样例
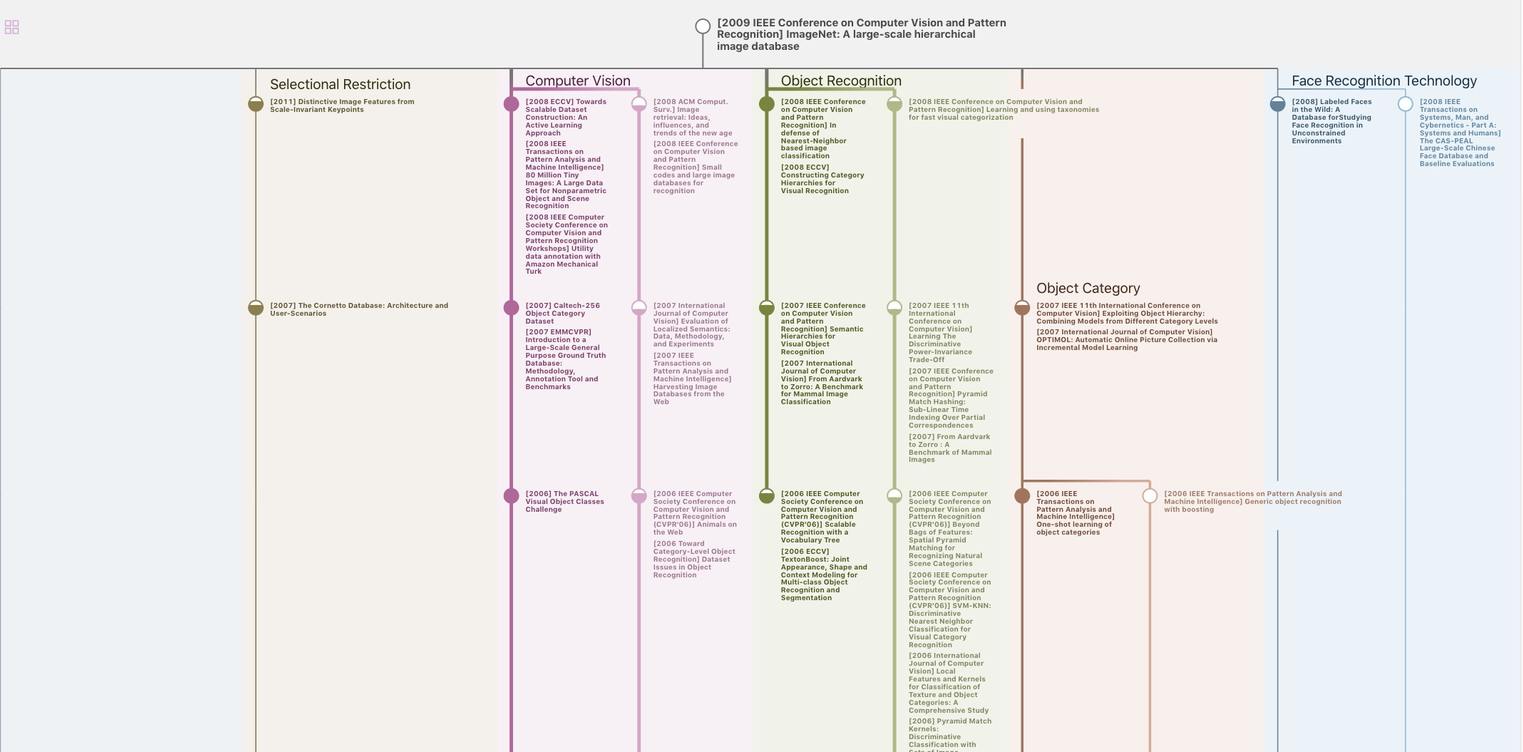
生成溯源树,研究论文发展脉络
Chat Paper
正在生成论文摘要