Post-Training Overfitting Mitigation in DNN Classifiers
CoRR(2023)
摘要
Well-known (non-malicious) sources of overfitting in deep neural net (DNN) classifiers include: i) large class imbalances; ii) insufficient training-set diversity; and iii) over-training. In recent work, it was shown that backdoor data-poisoning also induces overfitting, with unusually large classification margins to the attacker's target class, mediated particularly by (unbounded) ReLU activations that allow large signals to propagate in the DNN. Thus, an effective post-training (with no knowledge of the training set or training process) mitigation approach against backdoors was proposed, leveraging a small clean dataset, based on bounding neural activations. Improving upon that work, we threshold activations specifically to limit maximum margins (MMs), which yields performance gains in backdoor mitigation. We also provide some analytical support for this mitigation approach. Most importantly, we show that post-training MM-based regularization substantially mitigates non-malicious overfitting due to class imbalances and overtraining. Thus, unlike adversarial training, which provides some resilience against attacks but which harms clean (attack-free) generalization, we demonstrate an approach originating from adversarial learning that helps clean generalization accuracy. Experiments on CIFAR-10 and CIFAR-100, in comparison with peer methods, demonstrate strong performance of our methods.
更多查看译文
关键词
dnn classifiers,mitigation,post-training
AI 理解论文
溯源树
样例
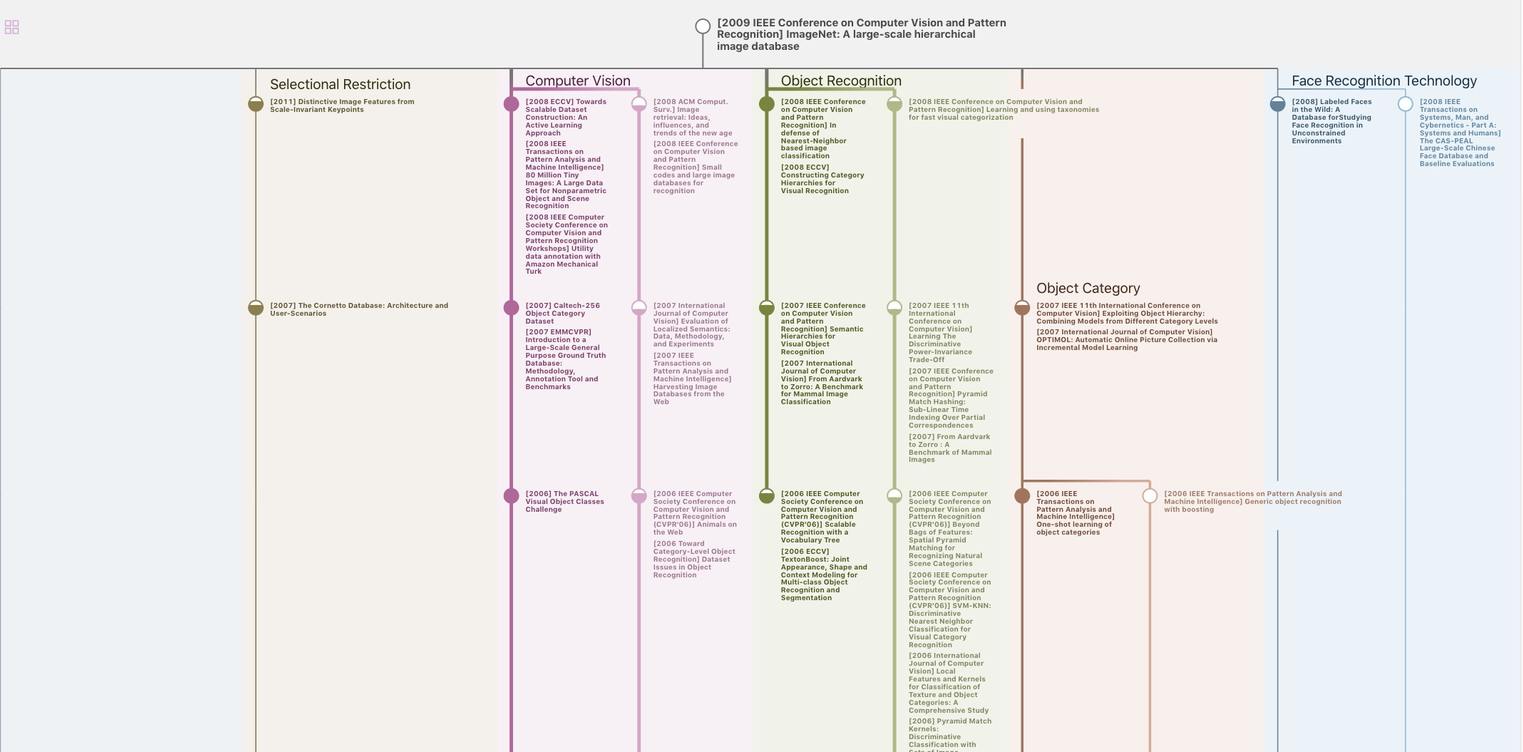
生成溯源树,研究论文发展脉络
Chat Paper
正在生成论文摘要